Open Access
ARTICLE
DaC-GANSAEBF: Divide and Conquer-Generative Adversarial Network—Squeeze and Excitation-Based Framework for Spam Email Identification
1 Department of Electrical Engineering, Faculty of Engineering at Rabigh, King Abdulaziz University, Jeddah, 21589, Saudi Arabia
2 Department of Electrical Engineering, College of Engineering, Northern Border University, Arar, 91431, Saudi Arabia
3 Center for Scientific Research and Entrepreneurship, Northern Border University, Arar, 73213, Saudi Arabia
4 Department of Information Technology, College of Computing and Information Technology, King Abdulaziz University, Jeddah, 21589, Saudi Arabia
5 Department of Information and Communication Technology, College of Computing Studies, Arab Open University, Kuwait Branch, Kuwait City, 13033, Al-Safat, State of Kuwait
6 Department of Information Systems and Technology, College of Computer Science and Engineering, University of Jeddah, Jeddah, 23218, Saudi Arabia
* Corresponding Author: Ahmed A. Alsheikhy. Email:
(This article belongs to the Special Issue: Applied Artificial Intelligence: Advanced Solutions for Engineering Real-World Challenges)
Computer Modeling in Engineering & Sciences 2025, 142(3), 3181-3212. https://doi.org/10.32604/cmes.2025.061608
Received 28 November 2024; Accepted 06 February 2025; Issue published 03 March 2025
Abstract
Email communication plays a crucial role in both personal and professional contexts; however, it is frequently compromised by the ongoing challenge of spam, which detracts from productivity and introduces considerable security risks. Current spam detection techniques often struggle to keep pace with the evolving tactics employed by spammers, resulting in user dissatisfaction and potential data breaches. To address this issue, we introduce the Divide and Conquer-Generative Adversarial Network Squeeze and Excitation-Based Framework (DaC-GANSAEBF), an innovative deep-learning model designed to identify spam emails. This framework incorporates cutting-edge technologies, such as Generative Adversarial Networks (GAN), Squeeze and Excitation (SAE) modules, and a newly formulated Light Dual Attention (LDA) mechanism, which effectively utilizes both global and local attention to discern intricate patterns within textual data. This approach significantly improves efficiency and accuracy by segmenting scanned email content into smaller, independently evaluated components. The model underwent training and validation using four publicly available benchmark datasets, achieving an impressive average accuracy of 98.87%, outperforming leading methods in the field. These findings underscore the resilience and scalability of DaC-GANSAEBF, positioning it as a viable solution for contemporary spam detection systems. The framework can be easily integrated into existing technologies to enhance user security and reduce the risks associated with spam.Keywords
Cite This Article
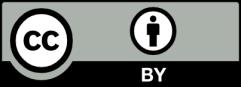
This work is licensed under a Creative Commons Attribution 4.0 International License , which permits unrestricted use, distribution, and reproduction in any medium, provided the original work is properly cited.