Open Access
ARTICLE
Quantum Inspired Adaptive Resource Management Algorithm for Scalable and Energy Efficient Fog Computing in Internet of Things (IoT)
1 Youth Office Haripur, Directorate of Youth Affairs, Government of Khyber Pakhtunkhwa, Haripur, 22620, Pakistan
2 School of Software Engineering, Dalian University of Technology, Ganjingzi District, Dalian, 116024, China
3 Department of Computer Engineering, College of Computer and Information Sciences, King Saud University, Riyadh, 11543, Saudi Arabia
4 Department of AI and Software, Gachon University, Seongnam-si, 13120, Republic of Korea
* Corresponding Author: Muhammad Shahid Anwar. Email:
Computer Modeling in Engineering & Sciences 2025, 142(3), 2641-2660. https://doi.org/10.32604/cmes.2025.060973
Received 13 November 2024; Accepted 10 February 2025; Issue published 03 March 2025
Abstract
Effective resource management in the Internet of Things and fog computing is essential for efficient and scalable networks. However, existing methods often fail in dynamic and high-demand environments, leading to resource bottlenecks and increased energy consumption. This study aims to address these limitations by proposing the Quantum Inspired Adaptive Resource Management (QIARM) model, which introduces novel algorithms inspired by quantum principles for enhanced resource allocation. QIARM employs a quantum superposition-inspired technique for multi-state resource representation and an adaptive learning component to adjust resources in real time dynamically. In addition, an energy-aware scheduling module minimizes power consumption by selecting optimal configurations based on energy metrics. The simulation was carried out in a 360-minute environment with eight distinct scenarios. This study introduces a novel quantum-inspired resource management framework that achieves up to 98% task offload success and reduces energy consumption by 20%, addressing critical challenges of scalability and efficiency in dynamic fog computing environments.Keywords
Cite This Article
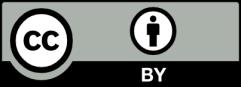
This work is licensed under a Creative Commons Attribution 4.0 International License , which permits unrestricted use, distribution, and reproduction in any medium, provided the original work is properly cited.