Open Access
ARTICLE
Deep Learning and Machine Learning Architectures for Dementia Detection from Speech in Women
1 Department of Computer Science, College of Engineering in Al-Lith, Umm Al-Qura University, Makkah, 24243, Saudi Arabia
2 Department of Information Systems, College of Computer and Information Sciences, Princess Nourah bint Abdulrahman University, Riyadh, 11671, Saudi Arabia
3 Department of Computer Science, College of Computer and Information Science, King Saud University, Riyadh, 11495, Saudi Arabia
* Corresponding Authors: Amel Ksibi. Email: ; Mohammed Zakariah. Email:
(This article belongs to the Special Issue: Intelligent Medical Decision Support Systems: Methods and Applications)
Computer Modeling in Engineering & Sciences 2025, 142(3), 2959-3001. https://doi.org/10.32604/cmes.2025.060545
Received 04 November 2024; Accepted 27 January 2025; Issue published 03 March 2025
Abstract
Dementia is a neurological disorder that affects the brain and its functioning, and women experience its effects more than men do. Preventive care often requires non-invasive and rapid tests, yet conventional diagnostic techniques are time-consuming and invasive. One of the most effective ways to diagnose dementia is by analyzing a patient’s speech, which is cheap and does not require surgery. This research aims to determine the effectiveness of deep learning (DL) and machine learning (ML) structures in diagnosing dementia based on women’s speech patterns. The study analyzes data drawn from the Pitt Corpus, which contains 298 dementia files and 238 control files from the Dementia Bank database. Deep learning models and SVM classifiers were used to analyze the available audio samples in the dataset. Our methodology used two methods: a DL-ML model and a single DL model for the classification of diabetics and a single DL model. The deep learning model achieved an astronomic level of accuracy of 99.99% with an F1 score of 0.9998, Precision of 0.9997, and recall of 0.9998. The proposed DL-ML fusion model was equally impressive, with an accuracy of 99.99%, F1 score of 0.9995, Precision of 0.9998, and recall of 0.9997. Also, the study reveals how to apply deep learning and machine learning models for dementia detection from speech with high accuracy and low computational complexity. This research work, therefore, concludes by showing the possibility of using speech-based dementia detection as a possibly helpful early diagnosis mode. For even further enhanced model performance and better generalization, future studies may explore real-time applications and the inclusion of other components of speech.Keywords
Cite This Article
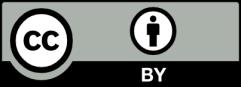
This work is licensed under a Creative Commons Attribution 4.0 International License , which permits unrestricted use, distribution, and reproduction in any medium, provided the original work is properly cited.