Open Access
ARTICLE
Congruent Feature Selection Method to Improve the Efficacy of Machine Learning-Based Classification in Medical Image Processing
1 Department of Computer Engineering, Aligarh Muslim University, Aligarh, 202002, India
2 College of Computer Science, King Khalid University, Abha, 61413, Saudi Arabia
3 School of Computing, Gachon University, Seongnam, 13120, Republic of Korea
4 Department of Computer Science and Information Systems, College of Applied Sciences, AlMaarefa University, Riyadh, 13713, Saudi Arabia
5 Department of Computer Engineering and Information, College of Engineering in Wadi Alddawasir, Prince Sattam bin Abdulaziz University, Al-Kharj, 16273, Saudi Arabia
* Corresponding Author: Hong Min. Email:
Computer Modeling in Engineering & Sciences 2025, 142(1), 357-384. https://doi.org/10.32604/cmes.2024.057889
Received 30 August 2024; Accepted 12 November 2024; Issue published 17 December 2024
Abstract
Machine learning (ML) is increasingly applied for medical image processing with appropriate learning paradigms. These applications include analyzing images of various organs, such as the brain, lung, eye, etc., to identify specific flaws/diseases for diagnosis. The primary concern of ML applications is the precise selection of flexible image features for pattern detection and region classification. Most of the extracted image features are irrelevant and lead to an increase in computation time. Therefore, this article uses an analytical learning paradigm to design a Congruent Feature Selection Method to select the most relevant image features. This process trains the learning paradigm using similarity and correlation-based features over different textural intensities and pixel distributions. The similarity between the pixels over the various distribution patterns with high indexes is recommended for disease diagnosis. Later, the correlation based on intensity and distribution is analyzed to improve the feature selection congruency. Therefore, the more congruent pixels are sorted in the descending order of the selection, which identifies better regions than the distribution. Now, the learning paradigm is trained using intensity and region-based similarity to maximize the chances of selection. Therefore, the probability of feature selection, regardless of the textures and medical image patterns, is improved. This process enhances the performance of ML applications for different medical image processing. The proposed method improves the accuracy, precision, and training rate by 13.19%, 10.69%, and 11.06%, respectively, compared to other models for the selected dataset. The mean error and selection time is also reduced by 12.56% and 13.56%, respectively, compared to the same models and dataset.Keywords
Cite This Article
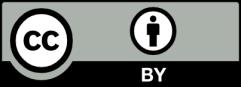
This work is licensed under a Creative Commons Attribution 4.0 International License , which permits unrestricted use, distribution, and reproduction in any medium, provided the original work is properly cited.