Open Access
REVIEW
Modeling and Comprehensive Review of Signaling Storms in 3GPP-Based Mobile Broadband Networks: Causes, Solutions, and Countermeasures
1 Department of Computer Science, Iqra National University, Peshawar, 25000, Pakistan
2 Department of Cybersecurity, College of Computer, Qassim University, Buraydah, 52555, Saudi Arabia
3 Department of Computer Science and Engineering, Al-Fayha College, Al Jubayl, 31961, Saudi Arabia
* Corresponding Author: Fahad Alturise. Email:
(This article belongs to the Special Issue: Computer Modeling for Future Communications and Networks)
Computer Modeling in Engineering & Sciences 2025, 142(1), 123-153. https://doi.org/10.32604/cmes.2024.057272
Received 13 August 2024; Accepted 25 November 2024; Issue published 17 December 2024
Abstract
Control signaling is mandatory for the operation and management of all types of communication networks, including the Third Generation Partnership Project (3GPP) mobile broadband networks. However, they consume important and scarce network resources such as bandwidth and processing power. There have been several reports of these control signaling turning into signaling storms halting network operations and causing the respective Telecom companies big financial losses. This paper draws its motivation from such real network disaster incidents attributed to signaling storms. In this paper, we present a thorough survey of the causes, of the signaling storm problems in 3GPP-based mobile broadband networks and discuss in detail their possible solutions and countermeasures. We provide relevant analytical models to help quantify the effect of the potential causes and benefits of their corresponding solutions. Another important contribution of this paper is the comparison of the possible causes and solutions/countermeasures, concerning their effect on several important network aspects such as architecture, additional signaling, fidelity, etc., in the form of a table. This paper presents an update and an extension of our earlier conference publication. To our knowledge, no similar survey study exists on the subject.Keywords
Control signaling is obligatory in all types of computer communication networks (wired or wireless) for operation and management. By definition, control signaling is the communication overhead that enables user authentication, authorization, charging, and billing [1]. Control signaling is also needed when, from a communication perspective, any important event happens such as mobile phones changing their connectivity state, sending or receiving data, and performing handovers [2]. The role and importance of control signaling will only increase in future networks and is expected to be a source of all intelligence available in the network [3]. Although control signaling is mandatory for the functioning of all types of communication networks, ideally it should be kept to a minimum as it occupies significant and scarce network resources (i.e., bandwidth and processing power). Maximum availability of these resources would simply mean that they could be used for user data communication instead and would help in not only fulfilling the user Quality of Service (QoS) but also trouble-free network operation. The usual method of measuring the amount of signaling traffic is the number of transactions or messages exchanged between network elements, while a single transaction might itself consist of one or several messages [4]. Because of its indisputable significance, a considerable amount of research work has already been done on minimizing the control signaling overhead for different types of computer networks. For example, in both Asynchronous Transfer Mode and Frame Relay Networks, the focus has been on optimal network performance by reducing the control signaling overhead [5]. A modern-day networking scenario consisting of a plethora of networking technologies available to the user is shown in Fig. 1.
Figure 1: Modern-day communication networks consisting of a plethora of heterogeneous technologies
However, today’s control signaling challenge to the 3rd Generation Partnership Project (3GPP) based mobile broadband networks is very severe, and signaling misbehavior can result in signaling storms [6], especially to the control plane [7]. The volume of signaling traffic depends upon the number of active mobile phones in the network [8]. Mobile broadband penetration has risen sharply, and inevitably with it comes the imminent signaling problem. The explosive growth in the use of mobile broadband for data access using smartphones gives rise to a data burden for which legacy wireless technologies were not designed. It also introduces another burden on the network in the form of the signaling load [9], resulting in degraded network performance [10]. Because the upsurge in mobile broadband data access is expected to occur in the future, the signaling influx will also thrive. What’s even more frightening is that many studies have highlighted that the increase in signaling traffic considerably outpaces the corresponding growth in data traffic. Signaling traffic is expected to be 50% up than the corresponding growth in data traffic over the next few years [4,11]. Such remarkable growth in the signaling traffic might overwhelm the capacity of 3GPP-based mobile broadband networks and turn into signaling storms. Signaling storms are a serious threat to all mobile broadband networks and in their severe forms can crash down a 3G/4G network completely or cause them to perform poorly [12]. The increase in the transmission signaling overhead increases the energy consumption of the network devices [13]. Several such incidents discussed in the next section have already occurred, costing the respective telecommunication companies big financial losses, in addition to leaving them with frustrated and unsatisfied customers.
Telenor, the world’s 14th largest telecom company, in June 2011 went out of service for 18 h due to a signaling load. The financial cost of this Telenor outage was estimated to be USD 18 million while affecting 3 million of its users [14,15]. Similarly, in April 2011, Verizon’s Long Term Evolution (LTE) network went out of service for 24 h after it crashed due to a signaling load it could not bear, impacting hundreds of thousands of its subscribers [16]. Moreover, this was not the only time Verizon 4G Network went down, but it crashed three times in December of the same year [17]. In Japan, the situation was not much different either. Its Telecom giant DoCoMo went down in January 2012 because of the signaling load and impacted its 2.52 million users in Tokyo only, and was the fifth such incident in only eight months [18]. More recently, in October 2021, around 2 million users of DoCoMo 3, 4, and 5G networks were left without voice and data services for more than 24 h due to excessive signaling [19,20]. Similarly, another Japanese operator, SoftBank, had to apologize to its customers after its 4G network went out of service for four hours due to software issues [21]. Surprisingly, such incidents happened in ten other countries at about the same time [22].
These network failure stories prove that the signaling storm problem is a real threat to stable network operation and requires feasible and long-lasting solutions. Telecom companies sensing this danger have mostly used over-provisioning as a solution. For example, Telenor tried to avoid control signaling problems in the future by investing in improving network capacity [15]. DoCoMo also followed the same route and spent 164 billion yen (USD 2.1 billion) to enhance its network capacity [18,23]. However, over-provisioning might only solve the problem temporarily, as growth in signaling traffic is not expected to slow down for some time but instead keep growing because of modern-day communication trending factors discussed in the next section. Therefore, innovative, multipronged, and long-term solutions are required. There might be a variety of reasons for signaling storms. Therefore, the research community needs to analyze them closely and come up with the corresponding feasible solutions. So far, the signaling storm problems have triggered a great deal of interest in the Telecom industry, but academic research is lagging. This is reflected in the references in this article, most of which are industry white papers, technical reports, and web links. This article draws the attention, of the academic research community to this issue by providing a detailed survey of the causes, of the signaling storm problems and their corresponding solutions in 3GPP-based mobile broadband networks and is an extension of our earlier work in [24]. To the author’s knowledge, no such survey exists on this subject. The rest of this article is arranged as follows.
Section 2 lists the main causes of the signaling storm problems in 3GPP-based mobile broadband networks along with its modeling. The corresponding potential solutions and countermeasures to these problems and their analytical models are discussed in Section 3. Finally, Section 4 concludes the paper and also provides future search directions.
2 Signaling Storms Main Causes
The reasons for signaling storms in 3GPP-based mobile broadband networks are diverse. While these factors can have a devastating effect on their own, their occurrence in tandem can further increase the possibility of causing severe signaling storms. While the reasons behind the causes themselves might be different, their result is an extra signaling load for the network. Some of these causes are shown in Fig. 2 and are discussed in the following section.
Figure 2: Signaling storms potential causes
2.1 Growth Trends in Mobile Broadband Data Access
Users in modern communication scenarios are increasingly becoming dependent on mobile broadband for Internet access compared to fixed broadband. As a result, a clear surge in mobile broadband data access and mobile users has been recorded. According to the Ericson Mobility report, global mobile data traffic grew by 70% between 2016 and 2017 [25]. The growth in mobile broadband data access is not going to slow down but instead is expected to gain more pace. As estimated by CISCO, the Compound Annual Growth Rate (CAGR) for mobile data access will be 46% in the period 2017–2022 [26] and 75% of the world’s mobile data traffic by 2020 will be composed of video [27]. Mobile broadband is the most dominant method for data access and has a share of 51.2% of total global online traffic, while the number of global unique mobile users was 3.7 billion in the first quarter of 2018 [28]. As the growth in data access is expected to continue into the next few years, the signaling traffic will also rise [24]. Therefore, with time, signaling storms will only get worse. With the growth in mobile broadband data access, many other factors will contribute to the signaling growth at the same time. In the Telecom industry, there have been many studies on this issue and all of them show a sharp increase not only in mobile broadband data access but also in the number of mobile subscribers, estimated at 5 billion in the second quarter of 2017 [29]. Legacy networks were not designed to cope with a control signaling load of this magnitude or to support billions of users. Therefore, network performance suffered and networks are facing frequent congestions, high call blocking and dropping probabilities, and poor user QoS. Growth in mobile broadband data access has not only affected 3G networks but also 4G networks such as LTE, which generate three times more signaling load in the Evolved Packet Core (EPC) [4]. Growth in data traffic is proposed to be calculated using the exponential model in Eq. (1) or the logistic growth model in Eq. (2).
where
where
2.2 Mobility Management (MM) Signaling Trends
The ability to communicate on the go has been probably the most dominant feature in the popularity and adoption of mobile networks. But this mobility support comes at a signaling cost from a network perspective [30]. All mobility-related functions such as paging, hand-offs, roaming, etc., are supported by the network with the help of mobility-specific control signaling. For MM, both the individual mobile node and the network need to coordinate through control signaling. The number of mobile Internet users is on the rise, as discussed in the previous section, machine-to-machine devices and applications with high mobility demands will result in heavy MM-related signaling [4,12,31]. The “always-on” communication model of the 3GPP-based mobile broadband networks for data access means a large number of active users for which MM functions must be performed. The 4G core network might be even more exposed to the MM signaling load due to its flat architecture, and all mobility-related signaling will have to traverse the core network [32]. In comparison, legacy, 2G, and 3G systems employed hierarchical architecture and because of that, were better suited to limiting the signaling load at a particular level in the hierarchy and not letting it traverse to the core network. Moreover, their hierarchical architecture also enabled these networks to employ localized MM techniques and procedures, which generate signaling overhead per the scope of mobility. Because of the 4G vision of heterogeneous network support, all types of available access media, such as 2G, 3GPP, 3GPP2, Wi-Fi, WiMAX, etc., could be used to improve the network capacity. But this also means that the EPC will have to deal with MM functions for a large number of users across heterogeneous radio systems. Therefore, this will further increase the amount of MM signaling that the core network must handle. Mobility-induced load can be estimated using Eq. (3). The total control signaling load
where
The handover signaling load
where
Similarly, the location update signaling load
where
The paging signaling load
where
One of the main attractions for mobile Internet users is the use of their favorite smart applications on smart devices such as cell phones and tablets. These applications can be used to send and receive rich-text messages, emojis, short animations, pictures, short videos, and audio clips. In addition to communication applications, users can have access to many other applications such as social networking, online games, news, weather updates, and maps. Some examples of these apps have been shown in Fig. 3. These applications must send periodic control messages, also referred to as “keep-alive or heartbeat or polling” messages, to their respective servers, even at times when the user is not communicating and without his knowledge [33,34]. The behavior of these applications is chatty, as they must poll the network many times, but each time requires a very small amount of data transfer [35,36]. Applications that are the chattiest can generate as many as 2400 signaling events in an hour [37]. From the network perspective, this is problematic, as it puts a lot of signaling load on the network and has caused signaling storms [12] and localized network outages [38]. The chattiness of applications is especially harmful in 3G networks, where the mobile node will be forced to go through a series of connections/disconnections to extend its battery life, also known as Fast Dormancy, discussed in the next section. Chatty applications, in addition to generating a heavy signaling load, will also consume scarce network resources such as bandwidth, Packet Data Protocol (PDP) states, and IP [39]. Thus, the chatty behavior of applications can have a very destructive effect on mobile networks. Similarly, highly active users who transfer only a small amount of data can have a significant inefficient signaling effect on the network [40]. Also, cloud-based services require constant synchronization with the cloud, which is achieved with the help of continuous heartbeat messages. All this chattiness will add to the problem of controlling signaling storms. The total control signaling load due to chatty applications
where
Figure 3: Chatty applications
In mobile communication, mobile devices must keep working for an extended period. Since all mobile devices have limited battery backup times, the mobile node may be asked to switch to a low-power mode when not communicating and a high-power mode when communicating, to save battery juice. This connectivity state transition has been visually shown in Fig. 4. This is exactly how Fast Dormancy works in 3G networks to prolong the battery life of mobile nodes. When a mobile node has no data to send or receive for 3 to 10 s, it will abandon all network connections by switching to idle mode and operating with low power to extend battery life. When the mobile node sends or receives any data, it must switch to active mode to resume all connections. From a signaling viewpoint, the mobile node must communicate its connectivity state changes to the network each time through control signaling. So that the network has an accurate view of the mobile node connectivity state at all times. Therefore, more connectivity state changes or transitions simply mean more control signaling for the network to cope with, as this might happen thousands of times daily [39]. Fast dormancy could be a problem for two reasons, the first opening and closing a connection on the radio link to send only a small amount of data is inefficient [12]. Secondly, the related network components such as the Radio Network Controllers (RNCs), which keep track of the mobile node connectivity state, were not designed for such a high transaction rate. This could lead to CPU overloads, leading to “brownouts” and poor service [12]. The mechanisms of Proprietary Fast Dormancy vary across different smartphone implementations. In some cases, the implementation has been optimized to prolong battery life, while in others the implementation has been optimized to minimize network signaling [41].
Figure 4: Mobile devices connectivity state transitions
The total control signaling load due to proprietary fast dormancy
where
Smartphones are designed in such a way that they always try their best to be connected to the network and be online at all times. However, if the remote network entity due to some technical problems cannot fulfill its connectivity or state change requests, the mobile node will still try repeatedly and will generate control signals in the process. For example, if the activation fails due to a breakdown of the network server, no service subscription, or simply because of insufficient capacity, the smartphone will repeatedly try to activate the PDP context. This causes a sharp increase in the PDP context activation signaling on the network and results in network congestion and control signaling overload. On one network of an operator, for example, the number of repeated PDP activations caused by failed activation accounted for 98.76% of the total PDP activations throughout the network. The success rate of activation was lower than 3%, resulting in a huge waste of network resources [39]. The total control signaling load due to abnormal signaling
where
Seeing the growth in mobile data demands, one can easily guess the growth in the use of smart devices as smart devices enable users to access mobile broadband data. Smart devices are becoming more common for data access because of their versatility not only in communication enabled by the Internet and its application but also in hardware. The modern smart device is usually equipped with digital cameras, a Global Positioning System, and different sensors. These devices range from smartphones to tablets and wearable devices such as smartwatches and health-monitoring body sensors, as shown in Fig. 5. Because of their great utility according to CISCO, smartphone usage, on average, has grown by 81% in 2012 [42]. According to another study in [27], almost half a billion mobile devices and connections were added in 2015. CISCO, in its annual report (2018–2023) claimed that smartphones will experience the second-fastest growth after Machine to Machine (M2M) [43]. But their popularity does not necessarily make them good for network control signaling. In fact, but from a signaling perspective, smart devices are not smart at all, and on average, generate 13 times more traffic than a non-smart device. In [39], the authors have discovered that despite their communication revolution, their use causes signaling storms. Nokia Siemens suggests that growth in the penetration of the smart devices combined with vendor-specific Operating System implementations can result in up to a 50% increase in signaling [4]. Surprisingly, the signaling load imposed on the network by various types of smart devices is different. This difference is due to the underlying behavior of the connectivity of these devices. As an example compared to laptops, smartphones download a very small amount of data (1/25th), but only three smartphones are enough to generate as much signaling as a single laptop [44]. This is because laptops, unlike smartphones, tend to connect to a network and stay connected. Since the use of smartphones outnumbers laptops, they generate the bulk of the signaling traffic, which can account for as much as 7% of a 3G subscriber’s contribution to network signaling costs [45]. In terms of signaling compared to smartphones, basic feature phones are more efficient as they only activate the PDP context when they communicate. But smartphones, on the other hand, activate a PDP context as soon as they are turned on and therefore have high PDP activation ratios, putting a more signaling burden on the network [39]. Also, a single smartphone can generate as much signaling traffic as 50 basic-feature phones; while a tablet has as many as 120 basic-feature phones, and a single laptop equals as many as 368 basic-feature phones [42]. Apart from the hardware, the operating systems of smart devices also differ in signaling performance and according to Huawei Android-based devices generate 3 times more signaling burden than the iPhone and 22 times the feature phone [39]. Estimation of signaling overhead generated due to the use of smart devices is proposed to be estimated using Eq. (10)
where
Figure 5: Smart devices
Although the new generation of mobile networks brings new enhancements and capabilities, it is always important to support the operation and integration of previous generations. Since 3G is the predecessor of 4G, the co-existence of both of these technologies is expected for many years in the future. Because of this, mobility support across these two technologies is a necessity. Despite having an All-IP core, the 4G systems have been designed to support almost all legacy systems such as 2G, 2.5G, and 3G [46], for capacity and compatibility reasons. But mobility support between 3G and 4G systems is signaling intensive [12]. Also, the communication and coordination between the respective cores of 3G and 4G systems for MM will put extra signaling burdens on the network as both networks maintain their respective user contexts. A scenario of 3G-LTE Mobility has been shown in Fig. 6.
Figure 6: 3G-LTE mobility scenario
The total signaling load due to 3G-LTE mobility
where
2.8 Licensed Small Cells Integration
One of the biggest challenges due to the growth in the number of mobile broadband users, growth in mobile data access and smart devices, is network capacity. Although operators use different techniques such as over-provisioning, new wireless transmission technologies, and efficient resource usage; the use of licensed small cells has been a very common and popular method for improving the needed network capacity, especially in urban areas [47]. But licensed small cell integration, giving rise to ultra-dense networks has a signaling overhead issues [48]. This is because they share common service procedures with macro cells and have a significant impact on the signaling load [12]. Also, a large number of small cells means that a highly mobile user in a cluster of small cells might increase the handoff and MM-related control signaling because of the frequent change of network point of attachment. Small cells need to be managed from the spectrum, mobility, and interference [49]. Integration of small cells may result in MM problems [50]. Some open challenges and opportunities to address signaling storms in the context of open radio access networks have been discussed in [51]. Fig. 7 represents small cells integration with macro cells.
Figure 7: Small cells integration
The total signaling load due to licensed small cells
where
Apart from the use of licensed small cells for better network capacity, Wi-Fi has also been used for traffic offloading and to relieve traffic burdens on the core network [52]. Wi-Fi 6 the new Wi-Fi standard is being viewed as both a competitor and complementary to 5G [53]. Although integrating Wi-Fi for traffic offloading is a promising solution for improving the network capacity, it also brings associated problems. One of these concerns is that all management of Wi-Fi cells will have to be done in the core network, and therefore more control traffic will traverse the common core. Support for Subscriber Identity Module-based authentication for Wi-Fi users is necessary but would require signaling to be exchanged with the relevant authentication servers, i.e., Home Subscriber Server (HSS) [12]. Similarly, other typical mobile network functions such as handoffs, authorization, and billing for Wi-Fi cell users will also generate more signaling load on the network.
The total signaling load due to Wi-Fi offloading
where
The diameter is a control signaling protocol developed by the Internet Engineering Task Force (IETF) [54]. The protocol provides functionalities commonly needed in mobile broadband networks such as Authentication, Authorization, and Accounting (AAA) functionality in IP networks. The diameter protocol is utilized by the 3GPP LTE network for several important management tasks such as MM, policy and charging, and QoS. Because of its critical role, the diameter signaling is on the rise and is expected to generate as many as 595 million messages per second with a CAGR of 23% by 2021 [55]. Early signaling storms have been attributed to diameter signaling and if its implementation and usage are not handled carefully, the signaling flood can overwhelm the network components with control signaling [12]. To reduce diameter control signaling, bulk operations on the large number of sessions managed by it are needed [56]. Diameter protocol may also introduce security vulnerabilities and can be used to launch denial-of-service attacks [57]. For the estimation of Diameter signaling, the following equation is proposed.
where
Alternatively, if the diameter signaling is viewed as a transaction then the total Diameter signaling load
where
2.11 Architectural Trends (3GPP LTE)
The network architecture of Mobile networks has come a long way from its infancy 1G systems to the current 4G and 5G systems. Earlier generations were based on hierarchical structures and were pure circuit-switched systems. However, with time as the importance of mobile broadband access was realized, the core network had to evolve to support packet-switched services. Therefore, the 3G core network was designed to have a hybrid architecture supporting both circuit and packet-switched services. To support the growing mobile broadband demand, the 4G system architecture has evolved to an all-IP core and flat architecture. Although the evolution to a flat architecture of mobile networks is for good reasons, it has a significant effect on how the evolved architecture tackles control signaling. For example, in 3G networks, the RNC works as a gateway between the access and the core network, limiting the flow of control signals from the access network to the core. The RNC can also use local MM, and an intra-RNC handover requires no global signaling. LTE, on the other hand, due to its flat architecture, makes the core network visible to the signaling load from the access network. In comparison to High-Speed Packet Access, a 3.5-G network, the LTE average signaling per subscriber is 42% higher [4]. Because of this, the core of the LTE network will face a signaling surge. The current LTE architecture lacks flexibility, cannot adapt to the user needs, and produces signaling storms [58,59]. A form of attack to choke the 5G core network with massive registration requests can lead to large-scale user service disruption [60]. Software-defined networking (SDN) has been proposed to provide the needed flexibility in the network architecture. SDN is based on the principle of separating the control plane and the data plan. But such separation can add to the network signaling [59].
Based on the discussion in this section, it can be safely stated that signaling surge due to architectural evolution mainly effect the core network. In the core network, control signaling load
where N is the number of active users or devices across the network, P is the average number of signaling messages per user or device, O is the overhead factor due to architectural changes, and H: is the additional handover and mobility management signaling factor handled by the core due to architectural evolution.
2.12 Security of Control Plane
Security is of prime importance for any communication network. A common attack on network infrastructure is to make a network component in the control plane process in large numbers some useless and pointless requests [61]. The radio resource control (RRC) protocol in 3G and 4G networks is particularly susceptible to security attacks [62]. Since the control plane is the driving force behind the network operations, choking the control plane will simply choke the entire network. Because of this fact, the control plane is a vulnerable target for attackers. In particular, in 3GPP-based networks, the attackers would generate numerous bearer setup requests or control signals, but would not complete the connection setup procedures, causing the related network entities to deal with a series of state transitions for bearer setup and release operations after timeouts, resulting simply in degraded QoS or in extreme cases Denial of Service (DoS) attacks [63,64].
The control signaling load
where A is the average number of malicious signaling requests per unit time, T is the timeout and retry factor and R is the impact factor on QoS due to security attacks.
2.13 Machine to Machine Communication (M2M) and Internet of Things (IoT)
Cisco predicts M2M devices to have the fastest growth in the period 2018 to 2023 [43]. The addition of a massive number of M2M devices will create a great burden for the mobile network system from the signaling perspective [65,66]. The introduction of M2M communications over mobile networks poses a signaling challenge; since the 3G and 4G mobile network protocols were not designed to handle frequent but small communications from a huge number of devices, as is the case in M2M traffic [64]. Apart from M2M, the Internet of Things (IoT) will also further fuel the signaling storms, as it enables communication for a very large number of smart devices [67]. The Industrial Internet of Things devices due to their low cost and complexity are vulnerable to being hacked to generate signaling storms in the networks [68,69].
According to a study performed in [70], there is a great variety among IoT devices regarding signaling, however, on average there are 55 K active devices per hour with an average traffic volume of 875 k per hour. While a typical IoT device (such as a humidity or temperature sensor) might generate only a small amount of data infrequently, they will contribute to a great extent to the signaling storms because of their sheer numbers. Therefore it is extremely important to analyze the signaling generated by IoT devices [71].
The control signaling load
where N is the number of active M2M and IoT devices, and R is the average signaling rate per device.
3 Signaling Storms Potential Solutions and Countermeasures
As discussed in the previous section, the causes behind signaling storm problems are many and of various types. Therefore, no single or super solution might exist, but instead, a coalescence of different solutions will be required to avoid signaling storms and maintain smooth network operations. To better tackle the signaling storm challenge, the operators must plan to ensure that their data and signaling processing capability, are per their projected growth and needs. They must also make certain that their network resources are used optimally. Only conventional strategies for increasing the network capacity, such as updating the air interface, and over-provisioning, will not be sufficient to address these challenges. Some solutions that operators can use to solve or at least control the signaling storm problems are shown in Fig. 8 and described individually in the next section.
Figure 8: Signaling storms potential solution
3.1 Network Performance Monitoring and Needs Projection
To solve the signaling storm problems due to the growth in mobile broadband data access, the operators must ensure that the network is scalable and adaptive. The operator must get key insights on their needs and requirements from the trends in data access demand in their network and market trends, and proactively plan their network upgrades. Insights into the current network health and signaling storms can be gathered by measuring key network performance indicators [72,73]. The operators must also analyze separately and consequently apply different solutions to the problems of signaling storms in the access and core networks. Knowing the network resources, user base, traffic patterns, and most current network traffic conditions can be useful from two aspects. First, the operator can use this knowledge to ensure that the key network components operate at their optimum performance at all times. Secondly, based on this knowledge, they can project their future needs and invest in their network infrastructure by employing Over-Provisioning, as discussed next. Needs projection can be done using several well-known Machine Learning (ML) and Artificial Intelligence (AI) models. Reference [74] uses a combination of time series prediction, adaptive threshold, and anomaly detection algorithms to predict signaling storms. Autoregressive integrated moving average (ARIMA) a statistical model represented in 19 is proposed for traffic load forecasting. A more general model based on ML is proposed in Eq. (20) can also be utilized.
where
3.2 Over-Provisioning and Enhanced Network Components
As discussed earlier in Section 1.2 that Over-Provisioning is the most basic and straightforward solution for operators if they are having trouble with network capacity. It is usually the first response of an operator to cope with an increased signaling load on the network, as was the case in Telenor [14,15], and Docomo [19] network outage incidents. Apart from using Over-Provisioning for more network bandwidth, the processing capacity of the most vulnerable network components, such as Serving GPRS (General Packet Radio Service) Support Node (SGSN) in 3G networks [39] can be enhanced as the needs arise. Although Over-Provisioning can be an important tool to fend off signaling storms due to growth in mobile broadband data access, their effect might only be temporary as the demand for user data grows continuously. Capacity planning is proposed to be done with the help of Eq. (21).
where
One of the key technologies the operators can utilize to fight the growth in mobile broadband data access; and to reduce the signaling and data traffic load is to intelligently offload traffic to Wi-Fi and femtocells from the macro cells [75,76]. This practice has already been successfully used and globally 33% of the total mobile data traffic was offloaded onto the fixed network through Wi-Fi or femtocells in 2012 [42]. A classical capacity enhancement technique finding renewed importance in the modern communication landscape is to use varying cell numbers and sizes as needed [47]. Using this technique, numerous small cells are used in high-density users in urban areas and a smaller number of large cells in rural areas where low user density is expected. To achieve the same, 4G and 5G networks support a variety of cell sizes, ranging from 10 m to 100 km. Offloading can be combined with energy efficiency to improve more than one aspect of the network [77]. However, the operators must be careful when using this feature as using many small cells might generate a lot of mobility and cell management-related signaling, as discussed before. Offloading benefits are proposed to be quantized with the help of the following equation.
where
The benefits of Traffic Offloading can be quantified using the following equation:
where
3.4 Distributed Mobility Management (DMM)
An answer to the signaling storm problems due to MM, might be to distribute the processing burden of one network component among multiple network components citeDMMSig citeDMM5G. To achieve this, Distributed Mobility Management (DMM) protocols and procedures might be utilized while preserving the flat architecture of the 4G networks. To this end, the IETF has chartered a special group by the name of DMM [78] to specify distributing mobility-related functions from the perspective of Mobile IP. To relieve the core network from performing MM for the heterogeneous access networks, the access networks might continue using their respective MM components while relaying only that information to the core network which is of particular interest. Different handover architectures for future networks have been compared based on their degree of distribution among multiple network components and their respective signaling overhead in [2,79]. From this comparison, the authors have concluded that a fully distributed approach generates less signaling overhead for the network than centralized and semi-distributed approaches, but puts high signaling burdens on the mobile node. A semi-distributed handover architecture distributes the signaling overhead more evenly among the networking components and the mobile node for better scalability and therefore might be better suited for future-generation networks. An efficient handover management and mobility management schemes for Wi-Fi networks have been introduced in [80–82]. Signaling improvements due to DMM are proposed to be estimated using the following equation.
where
3.5 Signaling Efficient Smart Application Design
Signaling storms due to the chatty or polling nature of the applications running on smart devices do not come under the direct control of network operators [4]. Therefore, there is not much the operators can do to solve this issue. However, the operators could share the network perspective on the signaling performance of these applications with the developers to advise them and provide application development guidelines to promote more intelligent application design. An intelligent application in this context would be an application that fulfills communication needs, taking into account network signaling performance [83]. As an example, instead of polling the network continuously for updates, push mechanisms might be utilized to push new updates if any [38], from the corresponding network entity to the mobile node. Smart timers could be used to reduce the unneeded signaling activity of apps that are chatty in nature [33]. In 3G networks, this would help reduce the number of control signals by reducing the number of times the mobile node, needs to change its connectivity state and also by reducing the PDP activation ratio. Another method used for the same purpose might be to have different PDP expiration timers depending on the user’s activity. Therefore, a highly active user might have a high value of PDP activation and vice versa for less active users, to reduce the PDP activation ratio and thus reduce the control signaling. The effect of polling reduction is quantized with the help of the following equation:
where
To optimize network signaling caused by chatty or polling applications, we propose a model where the rate of PDP activations per unit time,
Here,
3.6 Signaling Efficient Dormancy States
The cause behind the inefficient control signaling of proprietary fast dormancy is due to its several dormancy states. Therefore, a straightforward solution would be to have a simple model with a fewer number of transition states [84]. Few connectivity states would mean that the mobile node connectivity would change less often, and consequently, the control signaling needed to indicate this change to the network would be less. In contrast to the three-state energy model of 3G networks, LTE has only two states, i.e., RRC_CONNECTED and RRC_IDLE [38,63]. Because of the two states model, an LTE mobile node quickly transitions back to the connected mode to resume its data connections, which also results in less signaling overhead. Fast dormancy can be utilized in smartphones to optimize battery life and in some cases, it can be used for optimal control signaling [41]. Another way of mitigating control signaling due to fast dormancy is to use networked controlled fast dormancy; where the corresponding network entity can control the fast dormancy behavior to optimize both the control signaling and battery life of the mobile node. However, these solutions might only solve the problem temporarily, as the signaling challenge is an ongoing battle due to the expected continued growth in mobile broadband users and data access [12]. The inactive state has been proposed to be utilized for both reducing energy consumption and reducing communication delays [85]. By simplifying dormancy states and optimizing transitions, operators can mitigate control signaling caused by proprietary fast dormancy mechanisms, and improve network efficiency. The authors in [86] provide an insight into signaling attacks targeting RRC protocol generating signaling storms for 3G networks. We propose to use the following simple equation to estimate signaling load due to dormancy state transitions.
where the signaling load
Gains due to optimized dormancy are quantified as follows:
where
3.7 Signaling Behavior Monitoring
A very powerful technique that the operators can employ to fend off a signaling storm on more than one front, is to monitor the signaling behaviors of notorious network components and mechanisms in real-time; and take countermeasures if unwanted or malicious behavior is detected [87]. Statistical methods can also be employed for this purpose [88]. This is similar to the well-investigated area of research of using Artificial Intelligence and Machine Learning to detect DoS attacks. Signaling behavior monitoring can be used to detect abnormal RRC signaling [72,73]. A stochastic-based approach to reduce RRC state transitions because of keep-alive messages could also be employed [89]. For example, operators can monitor the repeated requests from a mobile node and when these requests surpass a certain threshold, they can be stopped from generating excessive signaling [39]. It can also be used to solve the problems of continuous virus scan [89] and control plane security [64]. Although an operator can detect a false signaling behavior, it cannot enforce a good signaling behavior on devices that are outside its control, such as mobile nodes. In general, keeping their network resources adequate following projections and efficient network operation will help. The operators can only educate regarding the complexities and sensitivities that might occur because of the signaling behavior of these devices. Similar to smartphone application developers, smartphone, and IoT device manufacturers should also take into account the network signaling load generated by these devices in the design phase of these devices. To reduce traffic congestion of particular links and some overloaded network components, a combined placement and routing algorithm approach is proposed in [90]. To manage signaling storms effectively, we propose the following model, where the signaling load
Here, T represents the threshold level for signaling parameters, and
3.8 Merging Network Components
Signaling load problems generated due to the mobility between 3G and LTE networks can be solved by minimizing the need for global signaling by combining the functionalities of multiple network components in one component and favoring local communications over global communication. As an example, signaling overhead over the S4 interface, between the SGSN of 3G and Mobility Management Entiry (MME) of 4G can be eliminated by internalizing their interaction, by co-locating both the network components in a single device [12]. This approach reduces the signaling overhead by eliminating the need for remote interaction between the SGSN and MME. Co-locating SGSN and MME can reduce control signaling by 30% and combining MME, SGSN and Serving Gateway (S-GW) as shown in Fig. 9, reduce the signaling by as much as 70% [12]. Similarly, the signaling load due to both separate user contexts in 3G and 4G, can be minimized by maintaining a common user context in the core network only. Paging is an important procedure of MM, and the severity of its signaling load can be mitigated by designing more robust paging schemes. For example, tracking area update in 4G networks is more efficient in signaling, than the routing area update in 3G networks. More optimized paging algorithms, which predict a user’s location from his mobility history, will further help in reducing the MM control signaling.
Figure 9: Combining MME, SGSN, and SG-W
The solution to signaling load problems, due to the evolution of network architecture, is tricky. Most of the solutions will have their related cost in terms of scalability. Big signaling savings can be achieved by physically combining more than one network component while keeping intact their logical and functional operations. Combining key network components reduces the need for global signaling and thus reduces the signaling load on the network. Moving the control plane close to the base stations also reduces the impact of signaling storms on mobile networks [59]. Also introducing additional network elements into the 4G architecture, such as Diameter Routing Agents (DRA’s) discussed in the next section, for controlling signaling storm issues, can be employed. A simple model to estimate the amount of traffic reduction by merging different components is proposed as follows.
where
To optimize signaling load, operators can merge network components and implement optimized paging schemes.
where
3.9 Utilizing Diameter Routing Agents
The signaling inefficiencies due to the growing use and responsibilities of the Diameter protocol curtailed using DRAs in the core networks [12]. DRAs are special devices that are used to reduce the number of connections between the core network components. The introduction of DRA agents simplifies the addition of new network components and also reduces Diameter signaling overhead. Having a common core for both 3G and LTE networks will also help in minimizing the diameter signaling load. A 3G and LTE packet gateway will implement a single policy across all access networks and thus reduce the need for diameter signaling [12]. According to the authors in [91], the main cause of 90% of signaling storms-induced network failures is due to the failure of the DRA agents. Potential reduction is signaling through optimized DRAs and its potential benefits can be estimated in a similar way to Eqs. (30), and (31), respectively.
3.10 Utilization of Distributed Mobility Anchors
The signaling load due to the integration of small licensed cells into the 4G architecture can be reduced on the core network by employing distributed and localized MM anchors. These components will handle local user requests on the local level, relaying only limited and necessary information to the core network. For example, specialized MM and distributed MME or gateways can be used to manage mobility across a cluster of licensed small cells. On the protocol level, special MM protocols that manage mobility for a group of users rather than individual users could be utilized to reduce the signaling cost. An example of such a protocol is Network Mobility (NEMO) [92] protocol developed by IETF. NEMO enables a mobile router to manage mobility signaling for all users inside a bus or train to convert it into a mobile pico/microcell. Signaling optimization in NEMO might directly be proportional to the number of users in its cell [93,94]. A group handover protocol to address signaling storm problems in non-terrestrial networks due to the high mobility of satellites has been proposed in [95]. The integration of Wi-Fi cells is somewhat similar to the integration of licensed small cells; therefore, more or less the same solution can be applied. To take advantage of distributed processing in this context, the user Home Location Register (HLR) or HSS profiles can be cached in the AAA servers dedicated to the authentication of Wi-Fi users [12]. One advantage of Wi-Fi from a radio network perspective is that Wi-Fi is based on the always-on model and therefore does not suffer the same connect/disconnect issue as 3G. The application of distributed mobility anchors for reducing signaling storms is similar to the use of DRA’s explained in the previous section for the same purpose. Likewise its signaling reduction potential and corresponding benefits can be estimated in a similar fashion using Eqs. (30), and (31), respectively.
3.11 Security Policy and Procedures
For mobile devices that overload the network with fake requests for resource allocation, a technique was proposed in reference [96]. This technique keeps track of the number of timeouts of important resource allocation due to inactivity and blocks the mobile devices from communication if the number exceeds a specific threshold to minimize the unwanted signaling load on the network. Also, techniques to avoid DoS and Distributed DoS (DDoS) attacks could prove very helpful. Wireless and Mobile networks can be monitored to detect signaling anomalies and network attacks [89,97] and to mitigate the effect of signaling storms [6]. Reduction is signaling through implementing security procedures and its potential benefits can be estimated using Eqs. (30), and (31), respectively.
3.12 Optimized Data Transmission Protocols
The signaling storm trouble due to M2M and IoT can be handled by using networking protocols that are optimized and customized to infrequent and small data transmissions [64]. The transmission of non-real-time data can be scheduled when the network is going through a period of underutilization. Real-time small units of Sensor data from IoT networks might be combined with regular data transmissions. Optimized dynamic resource allocation can be used to control signaling storms caused by massive M2M devices [98]. Signaling reduction through the use of optimized data transmission protocols can be calculated using Eqs. (30), and (31), respectively.
3.13 Network Function Virtualization
Network Function Virtualization (NFV) will hugely decrease signaling storms as it enhances the flexibility, scalability, and automation of the network, hence optimizing control signaling and preventing overload threats. The Management and Orchestration systems, regarding NFV, reduce the amount of signaling load that is caused by NFV as it dynamically provisions Virtual Network Functions to enhance efficiency in terms of utilization of available resources and prevent signaling bottlenecks when there is maximum demand [99,100]. This is made possible by NFV’s ability to create network slices and apply Service Function Chaining (SFC) where different types of traffic are segmented, based on specific signaling pathways, tailored to the nature of data involved in such transmission, thus limiting the propagation of signaling storms across the network [99–101]. The design of NFV is cloud-native, and it can make provisions for horizontal scaling and support for distributed deployments. Therefore, it promptly adapts to shifting network conditions, further reducing the effect of signaling storms with dynamic control of signaling processes [100]. This is what makes NFV a vital technology in the management and control of signaling surges in a highly complex network environment. However, NFV may introduce some new security challenges that are important to consider [102].
3.14 Future Generation Networks
Mobile networks have evolved from 1G to the most current 5G technology, as represented in Fig. 10. 5G is connecting around 40% of the world and is expected to account for around 75% of mobile data share in 2029 [103]. Next-generation Wireless Networks (NGNs), such as 5G and 6G, will have a dramatic capacity increase, transforming network operations for both users and network operators. The increase is expected to address the increasing levels of data transfer rates in present-day communication [104–106]). 5G will support dynamic and efficient resource allocation as compared to its predecessors [107]. 5G networks seek to integrate higher connection density with latency in the order of milliseconds, along with increased data rates. These are some driving forces behind IoT, AR, and VR [105,108]. Such flexibility and scalability could be achieved in these networks by the integration of technologies like SDN and NFV [104]. Further, 6G will integrate advanced technologies including terahertz communications and AI to offer even more data throughput and quality of service with much ease comparable to previous generations [106]. Although 5G and 6G have promising prospects, many challenges need to be met for them to meet future demands [109]. The expected increase in data traffic raises an issue of whether added capacity enhancements will be enough to make effective management of both data and signaling demand [110]. Because signaling storms might still be a challenge for 5G core [111,112]. Integrating revolutionary technologies such as NFV, small cells, spectrum sharing, etc., might open the door to new security challenges and security [113]. The control plane in the current 5G architecture may face serious security challenges [114]. It is thus a gargantuan challenge for network operators facing the complexity of handling enormous numbers of connected devices while in addition requiring real-time processing and low-latency communication [105]. A logical 6G mobile network architecture is proposed, emphasizing cost-effective, flexible, AI-driven, secure features to address 5G design lessons and inspire further research. 6G mobile network architecture must be designed, emphasizing cost-effectiveness, flexibility, native AI-driven, and security features in the light of lessons learned from 5G [115].
Figure 10: Generations of mobile networks
3.15 Use of Artificial Intelligence (AI) and Machine Learning (ML)
AI and ML can be used to optimize network performance by identifying patterns and making data-driven decisions to optimize network configurations, routing protocols, security, scalability, and resource allocation [116]. ML algorithms can be used to analyze historical records of data traffic and user mobility patterns and make future predictions [117]. The management of signaling storms in future-generation networks by using AI and ML would be critical for sustaining the stability and performance of these networks. AI and ML can predict and mitigate such storms by examining the historical signaling data and spotting the patterns before such events. In this regard, Deep learning has been used for network monitoring and traffic analysis [118], and has recently been proven to predict signal storms and trace back the roots; therefore, measures can be taken before the storm escalates [74]. Such ability will make possible efficient congestion control and load balancing, thus less probable causes of traffic overloads [119].
Similarly, AI-driven solutions strengthen the network infrastructure resilience through adaptive threshold-based signaling management as its mechanism, which automatically modifies signaling parameters according to real-time traffic conditions to which the network is subjected [74]. Such flexibility is useful, as network demand varies with increases in complexity and variability for widespread IoT device adoption and diverse applications [120]. Furthermore, integrating AI into network slicing can resource distribute fair space in different slices so that critical services are performing even when signaling events are peaking [121]. AI can also be used to optimize handovers by introducing proactive behaviour [122]. It is, therefore, critical to deploy AI and ML technologies strategically to solve the signaling intensity problems efficiently so that future-generation networks are both reliable and efficient.
In this section, we have discussed in detail the potential solutions to the signaling storm problems outlined in Section 2 and have provided the related analytical models for measuring their potential benefits. Table 1 provides a summary and a useful comparison of the respective potential solutions concerning the six different properties. The table consists of seven different columns. The first column “Signaling Storm Problems” lists all the different signaling storm problems discussed in this paper. The second column “Risk Severity” provides information on the potential risk of the problem for smooth network operations and is measured as “High,” “Med” or “Low”. The risk severity is decided based on the scope of the problems they cause inside the network if left untreated, and also based on their expected increasing role in future-generation networks. The third column in the table “Potential Solutions/Countermeasures” lists the potential solution(s) and countermeasures for each corresponding signaling storm problem. The fourth column “Within Operators Control” means if the potential solution is within the control of network operators and if they can take concrete steps to implement the solution, or if the solution is outside the scope of network operators. The fifth column “Solution Requirements” outlines the corresponding changes required to the network for the solutions to work. Such as new network components or new network functionalities and protocols required for the functionality of the solution. The sixth column “Signaling Cost” specifies if the potential solution will introduce its signaling for its functionality. A solution might have no “No Cost” meaning no additional signaling cost is introduced, or negligible cost “Negl”, meaning a very small additional signaling cost that can be neglected or ignored, or “consid” meaning considerable cost that should be taken seriously. The seventh and last column “Solution Fidelity” provides information on whether the respective solution is valid in the longer term (L.T) or will only work for the short term (ST) and in the future might require other innovative solutions.
In this article, we have provided a detailed survey of the different causes of the signaling storm problems in 3GPP-based mobile broadband networks. We have provided simple analytical models to quantify the effect of both the causes of signaling storms and the potential benefits of the solutions. Potential solutions and countermeasures have been compared to each other using key properties with the help of a table, which helps determine which solutions are feasible in the long term for better network signaling performance. From this survey study, we can conclude that the signaling storm problem is here to stay, as most of the current solutions will solve the signaling storm problem momentarily. More efforts focusing on long-term solutions and designing more robust networks are required. The network operators would need accurate and timely insights into their current network performance and based on them make precise projections regarding their future network growth and needs. Only then they will be able to formulate strategies that will help them in taming the signaling storms in the future. It is apparent from several studies that mobile networks are experiencing explosive growth in nearly all network usage aspects. The increase in broadband data access demands, an ever-growing number of mobile users and smart devices, new networking scenarios (such as integration of heterogeneous access networks); mobility support across heterogeneous systems, M2M and IoT, architectural evolution, and existing signaling inefficiencies are all resulting in too much control signaling for the network to handle. While numerous incidents of network operations being crippled by these signaling storms have already been reported, the control signaling threat keeps growing due to the projections of the increase in mobile broadband data access. Growing data demands and control signaling could simply be accommodated in the network if the network capacity somehow grows such that the network never gets overwhelmed. Future generation networks such as 5G, and 6G promise network capacity that could revolutionize how mobile networks operate, not just from the user perspective but also from the operator’s point of view. Although these new technologies are extremely promising, it remains to be seen if their promised capacity would be enough to keep on with the growing data demands and consequently keep a check on the signaling demands.
This study is limited to the 3GPP-based networks only, which have been already in service for some time, such as 3G and 4G networks. In the future, this study could be extended to comprehensively focus on 5G which is actively being deployed worldwide currently, and 6G. These technologies have been proposed as revolutionary technologies to fulfill their design goals and will have a deep impact on how core network devices perform their basic functions. Therefore, will have a detrimental impact on the signaling efficiency of future networks. It might also be interesting to investigate the effects of signaling storms on a particular network service.
Acknowledgement: None.
Funding Statement: The researchers would like to thank the Deanship of Graduate Studies and Scientific Research at Qassim University for financial support (QU-APC-2024-9/1).
Author Contributions: The authors confirm contribution to the paper as follows: study conception and design: Muhammad Qasim Khan, Fazal Malik; funding acquisition: Fahad Alturise; draft manuscript preparation: Muhammad Qasim Khan, Noor Rahman; project administration: Fahad Alturise. All authors reviewed the results and approved the final version of the manuscript.
Availability of Data and Materials: Not applicable.
Ethics Approval: Not applicable.
Conflicts of Interest: The authors declare no conflicts of interest to report regarding the present study.
References
1. More fuel for the signaling storm. 2012 Apr 20. Available from: http://lteconference.wordpress.com/2012a/04/20/new-ipad-more-fuel-for-the-signalling-storm/. [Accessed 2024]. [Google Scholar]
2. Khan MQ, Andresen SH. A semi and fully distributed handover algorithm for heterogeneous networks using MIIS. In: Proceedings of IEEE Symposium on Computers and Communications (ISCC2012; Cappadocia, Turkey; pp. 145–150. [Google Scholar]
3. Husic JB, Bajric H, Barakovic S. Evolution of signaling information transmission. Int Sch Res Notices. 2015;2012:9. [Google Scholar]
4. Nokia Siemens Network. Signaling is growing 50% faster than data traffic (White Paper); 2012. Available from: http://docplayer.net/6278117-Signaling-is-growing-50-faster-than-data-∖traffic.html. [Accessed 2024]. [Google Scholar]
5. Stallings W. Data and computer communications. London, UK: Pearson Prentice Hall, Pearson Education, Inc.; 2007. [Google Scholar]
6. Abdelrahman OH, Gelenbe E. Signalling storms in 3G mobile networks. In: 2014 IEEE International Conference on Communications (ICC), 2014; Sydney, Australia; p. 1017–22. [Google Scholar]
7. Moradi M, Wu W, Li LE, Mao ZM. SoftMoW: Recursive and reconfigurable cellular WAN architecture. In: Proceedings of the 10th ACM International on Conference on Emerging Networking Experiments and Technologies, 2014; Sydney, Australia; p. 377–90. [Google Scholar]
8. Oteyo IN, Bainomugisha E. Volume of signaling traffic reaching cellular networks from mobile phones. In: 2017 IEEE AFRICON, 2017; Cape Town, South Africa: IEEE; p. 831–6. [Google Scholar]
9. Metzger F, Rafetseder A, Romirer-Maierhofer P, Tutschku K. Exploratory analysis of a GGSN’s PDP context signaling load. J Comput Netw Commun. 2015;2014:13. [Google Scholar]
10. Elnashar A, El-Saidny MA, Reda M. Managing smartphones signalling load in UMTS networks: a practical analysis. 2018. doi:10.48550/arXiv.1810.02972. [Google Scholar] [CrossRef]
11. 3GPP. Technical specification group radio access network, LTE RAN enhancements for diverse data applications (Release 11). In: 2013 IEEE Wireless Communications and Networking Conference (WCNC), 2012; Shanghai, China. [Google Scholar]
12. Brown G. The evolution of the signaling challenge in 3G and 4G networks (White Paper); 2012. Available from: https://www.academia.edu/5914958/White_Paper_The_Evolution_of_the_Signaling_Challenge_in_3G_and_4G_Networks_Prepared_by_Gabriel_Brown_Senior_Analyst_Heavy_Reading_The_Scope_of_the_Signaling_Challenge. [Accessed 2024]. [Google Scholar]
13. Imran M, Hayat O, Ngah R. Signalling overhead diminution in M-MIMO using NOMA transmission. Discover Appl Sci. 2024;6(1):15. doi:10.1007/s42452-024-05648-0. [Google Scholar] [CrossRef]
14. Fisher AD. Signal storm caused Telenor outages; 2011. Available from: http://www.newsinenglish.no/2011/06/16/signal-storm-caused-telenor-outages/. [Accessed 2024]. [Google Scholar]
15. Telenor Group (TELN). Telenor explains mobile outage; 2011. Available from: http://www.lightreading.com/telenor-explains-mobile-outage/d/d-id/687713. [Accessed 2024]. [Google Scholar]
16. 5G Technology World. Verizon reports LTE network crash; 2011. Available from: https://www.ecnmag.com/news/2011/04/verizon-reports-lte-network-crash. [Accessed 2024]. [Google Scholar]
17. 5G Technology World. With month’s 3rd outage, is Verizon still ‘most reliable?’ 2011. Available from: https://money.cnn.com/2011/12/29/technology/verizon_4g_outage/index.htm. [Accessed 2024]. [Google Scholar]
18. Fujimura N. DoCoMo to spend 164 billion yen on network after service outage; 2012. Available from: http://www.bloomberg.com/news/2012-01-27/docomo-to-spend-164-billion-yen-on-network-after-service-outage.html. [Accessed 2024]. [Google Scholar]
19. Global Data Technology. Docomo outage demonstrates the danger of signalling storms; 2021. Available from: https://www.verdict.co.uk/docomo-signalling-storm-outages/. [Accessed 2024]. [Google Scholar]
20. Nikkei. Docomo mobile service disruptions in Japan extend into second day; 2021. Available from: https://asia.nikkei.com/Business/Telecommunication/Docomo-mobile-service-disruptions-in-Japan-extend-into-secondday#:~:text=In%20January%202019%2C%20the%20communications,for%20about%20four%20and%20a. [Accessed 2024]. [Google Scholar]
21. SoftBank Corp. Apology for mobile communication service troubles; 2018. Available from: https://www.softbank.jp/en/corp/news/press/sbkk/2018/20181206_02/. [Accessed 2024]. [Google Scholar]
22. Mainichi. Gov’t to demand SoftBank explain cell service outage; 11 other countries also hit; 2018. Available from: https://mainichi.jp/english/articles/20181207/p2a/00m/0na/016000c. [Accessed 2024]. [Google Scholar]
23. Fujimura N. NTT Docomo to spend 164 billion yen on network upgrades; 2012. Available from: https://www.bloomberg.com/news/articles/2012-01-27/docomo-to-spend-164-billion-yen-on-network-after-service-outage. [Accessed 2024]. [Google Scholar]
24. Khan MQ. Signaling storm problems in 3GPP mobile broadband networks, causes and possible solutions: a review. In: 2018 International Conference on Computing, Electronics Communications Engineering (iCCECE), 2018; Southend, UK: University of Essex; p. 183–8. [Google Scholar]
25. Ericsson. Ericsson mobility report (White Paper); 2017. Available from: https://www.ericsson.com/assets/local/mobility-report/documents/2017/ericsson-mobility-report-june-2017.pdf. [Accessed 2024]. [Google Scholar]
26. Cisco. Cisco visual networking index: forecast and trends, 2017–2022 (White Paper); 2018. Available from: https://twiki.cern.ch/twiki/pub/HEPIX/TechwatchNetwork/HtwNetworkDocuments/white-paper-c11-741490.pdf. [Accessed 2024]. [Google Scholar]
27. Cisco. Cisco visual networking index: global mobile data traffic forecast update, 2015–2020 (White Paper); 2016. Available from: http://www.audentia-gestion.fr/cisco/pdf/mobile-white-paper-c11-520862.pdf. [Accessed 2024]. [Google Scholar]
28. Statista. Mobile Internet-Statistics & Facts; 2018. Available from: https://www.statista.com/topics/779/mobile-internet/. [Accessed 2024]. [Google Scholar]
29. GSMA. GSMA intelligence: global mobile trends 2017 (White Paper); 2017. Available from: https://www.gsma.com/asia-pacific/resources/global-mobile-trends-2017/. [Accessed 2024]. [Google Scholar]
30. Shayea I, Ergen M, Hadri Azmi M, Aldirmaz Çolak S, Nordin R, Daradkeh YI. Key challenges, drivers and solutions for mobility management in 5G networks: a survey. IEEE Access. 2020;8:172534–52. [Google Scholar]
31. Hayat O, Kaleem Z, Zafarullah M, Ngah R, Hashim SZM. Signaling overhead reduction techniques in device-to-device communications: paradigm for 5G and beyond. IEEE Access. 2021;9:11037–50. doi:10.48550/arXiv.1810.02972. [Google Scholar] [CrossRef]
32. Nokia Siemens Networks. Enabling mobile broadband growth–Evolved Packet Core (White Paper); 2011. Available from: http://www.mforum.ru/arc/20110319_NSN_EPC_wp_MForum.pdf. [Accessed 2024]. [Google Scholar]
33. Hobfeld CSFLT, Tran-Gia P. Angry apps: the impact of network timer selection on power consumption, signalling load, and web QoE. J Comput Netw Commun. 2013. doi:10.1155/2013/176217. [Google Scholar] [CrossRef]
34. Jin Y, Liu F, Yi X, Chen M. Reducing cellular signaling traffic for heartbeat messages via energy-efficient D2D forwarding. In: 2017 IEEE 37th International Conference on Distributed Computing Systems (ICDCS), 2017; Atlanta, GA, USA; p. 1301–11. [Google Scholar]
35. Zhang H, Xue K, Hong P. Facing the signaling storm: a method with stochastic concept. In: 2014 Sixth International Conference on Wireless Communications and Signal Processing (WCSP), 2014; Hefei, China; p. 1–6. [Google Scholar]
36. Tao Z, Chen L, Zhen S, Han P. Simulation and analysis of EVDO network for mobile internet traffic behavior. In: Wireless Internet. 7th International ICST Conference, WICON 2013, 2013 Apr 11–12; Shanghai, China. [Google Scholar]
37. Parker T. Chatty smartphones stressing networks; 2012. Available from: https://www.fiercewireless.com/tech/chatty-smartphones-stressing-networks. [Accessed 2024]. [Google Scholar]
38. AT&T. Best practices for 3G and 4G APP development (White Paper); 2012. Available from: https://www.scribd.com/document/368235239/best-practices-3g-4g-app-development-pdf. [Accessed 2024]. [Google Scholar]
39. Yang C. Weather the signaling storm; 2011. Available from: https://www.huawei.com/mediafiles/CORPORATE/PDF/Magazine/communicate/61/HW_094153.pdf. [Accessed 2024]. [Google Scholar]
40. Li J, Liu Y, Pei W, Cao Z. Behaviors of high-frequency subscribers in cellular data networks. Secur Commun Netw. 2018;2018(2):12. [Google Scholar]
41. GSMA. Fast dormancy best practises. Available from: https://www.scribd.com/document/64209207/Fast-Dormancy-Best-Practices. [Accessed 2024]. [Google Scholar]
42. CISCO. Cisco visual networking index: global mobile data traffic forecast update, 2012–2017 (White Paper); 2013. Available from: http://boletines.prisadigital.com/Cisco_Visual_Networking.pdf. [Accessed 2024]. [Google Scholar]
43. CISCO. Cisco Annual Internet Report (2018–2023) (White Paper); 2020. Available from: https://www.cisco.com/c/en/us/solutions/collateral/executive-perspectives/annual-internet-report/white-paper-c11-741490.html. [Accessed 2024]. [Google Scholar]
44. AIRVANA, INC. Airvana identifies 8X network load multiplier effect for smartphones; 2009. Available from: https://m.marketscreener.com/AIRVANA-INC-57222/news/AIRVANA-INC-Airvana-Identifies-8X-Network-Load-Multiplier-Effect∖-for-Smartphones-13261085/. Airvana press release [Accessed 2024 Jul 9]. [Google Scholar]
45. Signals Research Group. The impact of smartphones on 3G network performance; 2010. Available from: http://signalsresearch.com/issue/lte-world-summit-2010-7/. [Accessed 2024]. [Google Scholar]
46. Stefania S, Issam T, Matthew B. LTE–the UMTS long term evolution: from theory to practice. Hoboken, NJ, USA: Wiley; 2011. [Google Scholar]
47. GSMA. Improving wireless connectivity through small cell deployment; 2016. Available from: https://www.gsma.com/publicpolicy/wp-content/uploads/2016/12/GSMA_Small_Cell_Deployment_Booklet.pdf. [Accessed 2024]. [Google Scholar]
48. Adedoyin MA, Falowo OE. Combination of ultra-dense networks and other 5G enabling technologies: a survey. IEEE Access. 2020;8:22893–932. doi:10.1109/Access.6287639. [Google Scholar] [CrossRef]
49. Claussen H, Lopez-Perez D, Ho L, Razavi R, Kucera S. Small cell networks: deployment, management, and optimization. Hoboken, NJ, USA: John Wiley & Sons; 2018. p. 505–47. [Google Scholar]
50. Gures E, Shayea I, Alhammadi A, Ergen M, Mohamad H. A comprehensive survey on mobility management in 5G heterogeneous networks: architectures, challenges and solutions. IEEE Access. 2020;8:195883–913. doi:10.1109/ACCESS.2020.3030762. [Google Scholar] [CrossRef]
51. Tabiban A, Alameddine HA, Salahuddin MA, Boutaba R. Signaling storm in O-RAN: challenges and research opportunities. IEEE Commun Mag. 2024;62(6):58–64. doi:10.1109/MCOM.002.2300317. [Google Scholar] [CrossRef]
52. Wireless Broadband Alliance (WBA). Telco operators should be much more strategic when it comes to incorporating WiFi into their product offering; 2017. Available from: https://wballiance.com/more-than-60-of-global-mobile-data-traffic-will-be-offloaded-onto-wifi. [Accessed 2024]. [Google Scholar]
53. Oughton EJ, Lehr W, Katsaros K, Selinis I, Bubley D, Kusuma J. Revisiting wireless internet connectivity: 5G vs Wi-Fi 6. Telecommun Policy. 2021;45(5):102127. doi:10.1016/j.telpol.2021.102127. [Google Scholar] [CrossRef]
54. Calhoun P, Loughney J, Guttman E, Zorn G, Arkko J. Diameter base protocol IETF RFC 3588. Available from: http://www.ietf.org/rfc/rfc3588.txt. [Accessed 2024]. [Google Scholar]
55. Oracle. LTE diameter signaling index 6th edition (White Paper); 2017. Available from: http://www.oracle.com/us/industries/communications/comms-dsi-6th-signaling-info-3941804.pdf. [Accessed 2024]. [Google Scholar]
56. Jones M, Liebsch M, Morand L. RFC 9390: diameter group signaling. USA: RFC Editor; 2023. [Google Scholar]
57. Tan Z, Ding B, Zhang Z, Li Q, Guo Y, Lu S. Device-centric detection and mitigation of diameter signaling attacks against mobile core. In: 2021 IEEE Conference on Communications and Network Security (CNS), 2021; p. 29–37. [Google Scholar]
58. Pozza M, Pozza M, Lindholm H, Lindholm H, Flinck H, Flinck H, et al. Solving signaling storm through long-term evolution refactoring. Tiny Trans Comput Sci. 2016;4. [Google Scholar]
59. Pozza M, Rao A, Bujari A, Flinck H, Palazzi CE, Tarkoma S. A refactoring approach for optimizing mobile networks. In: 2017 IEEE International Conference on Communications (ICC), 2017; Paris, France; p. 1–6. [Google Scholar]
60. Zhang B, Zeinaty P, Limam N, Boutaba R. Mitigating signaling storms in 5G with blockchain-assisted 5GAKA. In: 2023 19th International Conference on Network and Service Management (CNSM), 2023; Niagara Falls, ON, Canada; p. 1–9. [Google Scholar]
61. Ettiane R, Chaoub A, Elkouch R. Toward securing the control plane of 5G mobile networks against DoS threats: attack scenarios and promising solutions. J Inf Secur Appl. 2021;61:102943. [Google Scholar]
62. Gorbil G, Abdelrahman OH, Pavloski M, Gelenbe E. Modeling and Analysis of RRC-Based Signalling Storms in 3G Networks. IEEE Trans Emerg Top Comput. 2016 Jan–Mar;4(1):113–27. doi:10.1109/TETC.2015.2389662. [Google Scholar] [CrossRef]
63. Bassil R, Elhajj IH, Chehab A, Kayssi A. Effects of signaling attacks on LTE networks. In: 2013 27th International Conference on Advanced Information Networking and Applications Workshops, 2013; Barcelona, Spain; p. 499–504. [Google Scholar]
64. Gorbil G, Abdelrahman OH, Gelenbe E. Storms in mobile networks. In: Proceedings of the 10th ACM Symposium on QoS and Security for Wireless and Mobile Networks. Q2SWinet ’14, 2014; New York, NY, USA: ACM; p. 119–26. doi:10.1145/2642687.2642688. [Google Scholar] [CrossRef]
65. Htike Z, Haniz A, Matsumura T, Kojima F. An efficient group-based mobility management mechanism for cellular M2M networks. In: 2021 IEEE 18th Annual Consumer Communications & Networking Conference (CCNC), 2021; p. 1–7. [Google Scholar]
66. Ahuja K, Bala I. M2M in 5G cellular networks: challenges, proposed solutions, and future directions. In: Wireless communication security. 2022. p. 1–22. doi:10.1002/9781119777465.ch1. [Google Scholar] [CrossRef]
67. Al-Sarawi S, Anbar M, Abdullah R, Al Hawari AB. Internet of Things market analysis forecasts, 2020–2030. In: 2020 Fourth World Conference on Smart Trends in Systems, Security and Sustainability (WorldS4), 2020; p. 449–53. [Google Scholar]
68. Hoffmann M, Kryszkiewicz P. Signaling storm detection in IIoT network based on the open RAN architecture. In: IEEE INFOCOM, 2023-IEEE Conference on Computer Communications Workshops (INFOCOM WKSHPS), 2023; New York, NY, USA; p. 1–2. [Google Scholar]
69. Shehu Yalli J, Hilmi Hasan M, Abubakar Badawi A. Internet of Things (IoTorigins, embedded technologies, smart applications, and its growth in the last decade. IEEE Access. 2024;12:91357–82. doi:10.1109/ACCESS.2024.3418995. [Google Scholar] [CrossRef]
70. Geissler S, Wamser F, Bauer W, Krolikowski M, Gebert S, Hobfeld T. Signaling traffic in internet-of-things mobile networks. In: 2021 IFIP/IEEE International Symposium on Integrated Network Management (IM), 2021; p. 452–8. [Google Scholar]
71. Geibler S, Lutu A, Wamser F, Favale T, Vomhoff V, Krolikowski M, et al. Untangling IoT global connectivity: the importance of mobile signaling traffic. IEEE Trans Netw Serv Manage. 2024;21(4):4435–49. doi:10.1109/TNSM.2024.3414975. [Google Scholar] [CrossRef]
72. Abdelrahman OH. Detecting network-unfriendly mobiles with the random neural network. Probab Eng Inf Sci. 2016;30(3):514–31. doi:10.1017/S0269964816000140. [Google Scholar] [CrossRef]
73. Abdelrahman O, Gelenbe E. A data plane approach for detecting control plane anomalies in mobile networks. In: Internet of things. IoT infrastructures: second international summit, IoT 360°. Rome, Italy: Springer International Publishing; 2015 Oct 27–29. p. 210–21. [Google Scholar]
74. Feng DJ, Li S, Yang Z, Xiang Y, Zheng J, Huang H. Research of deep learning and adaptive threshold based signaling storm prediction and top cause tracking. IEEE Access. 2023;11:120603–11. [Google Scholar]
75. Rebecchi F, Dias de Amorim M, Conan V, Passarella A, Bruno R, Conti M. Data offloading techniques in cellular networks: a survey. IEEE Commun Surv Tutor. 2015;17(2):580–603. doi:10.1109/COMST.9739. [Google Scholar] [CrossRef]
76. Marvi M, Aijaz A, Khurram M. Toward an automated data offloading framework for multi-RAT 5G wireless networks. IEEE Trans Netw Serv Manage. 2020;17(4):2584–97. doi:10.1109/TNSM.2020.3026948. [Google Scholar] [CrossRef]
77. Bacanin N, Antonijevic M, Bezdan T, Zivkovic M, Venkatachalam K, Malebary S. Energy efficient offloading mechanism using particle swarm optimization in 5G enabled edge nodes. Cluster Comput. 2023;26(1):587–98. doi:10.1007/s10586-022-03609-z. [Google Scholar] [CrossRef]
78. Internet Engineering Task Force (IETF). Distributed Mobility Management (DMM) IETF working group. Available from: https://datatracker.ietf.org/wg/dmm/about/. [Accessed 2024]. [Google Scholar]
79. Khan MQ, Andresen SH, Ul Haq MI. Handover architectures for heterogeneous networks using the Media Independent Information Handover (MIH). Comput Inform. 2016;35(1):177–202. [Google Scholar]
80. Khan MQ, Andresen SH. Zero scanning time for 802.11 networks by using Media Independent Information Server (MIIS). In: 2013 IEEE 27th International Conference on Advanced Information Networking and Applications (AINA), 2012; Los Alamitos, CA, USA: IEEE Computer Society; p. 467–73. doi:10.1109/AINA.2012.100. [Google Scholar] [CrossRef]
81. Khan MQ, Andresen SH. An intelligent scan mechanism for 802.11 networks by using Media Independent Information Server (MIIS). In: Proceedings of the 2011 IEEE Workshops of International Conference on Advanced Information Networking and Applications, WAINA’11, 2011; Biopolis, Singapore: IEEE Computer Society; p. 221–5. doi:10.1109/WAINA.2011.26. [Google Scholar] [CrossRef]
82. Khan MQ, Andresen SH. PoA selection in 802.11 networks using Media Independent Information Server (MIIS). In: Proceedings of the 2012 26th International Conference on Advanced Information Networking and Applications Workshops, WAINA’12, 2012; Biopolis, Singapore: IEEE Computer Society; p. 454–9. doi:10.1109/WAINA.2012.142 [Google Scholar] [CrossRef]
83. Choi Y, Yoon CH, Kim YS, Heo SW, Silvester JA. The impact of application signaling traffic on public land mobile networks. IEEE Commun Mag. 2014;52(1):166–72. doi:10.1109/MCOM.2014.6710079. [Google Scholar] [CrossRef]
84. Yong L, Hou YB. Study on signaling storm in 3G networks. Appl Mech Mater. 2013;336-338(20):2455–9. doi:10.4028/www.scientific.net/AMM.336-338.2455. [Google Scholar] [CrossRef]
85. Ding Z, Lin Y, Xu W, Lv J, Gao Y, Dong W. Energy optimization for mobile applications by exploiting 5G inactive state. IEEE Trans Mob Comput. 2024;23:1–15. [Google Scholar]
86. Gorbil G, Abdelrahman OH, Pavloski M, Gelenbe E. Modeling and analysis of RRC-based signalling storms in 3G networks. IEEE Trans Emerg Topics Comput. 2016;4(1):113–27. doi:10.1109/TETC.6245516. [Google Scholar] [CrossRef]
87. Dong S, Xia Y, Peng T. Network abnormal traffic detection model based on semi-supervised deep reinforcement learning. IEEE Trans Netw Serv Manage. 2021;18(4):4197–212. doi:10.1109/TNSM.2021.3120804. [Google Scholar] [CrossRef]
88. Hu Z, Odarchenko R, Gnatyuk S, Zaliskyi M, Chaplits A, Bondar S, et al. Statistical techniques for detecting cyberattacks on computer networks based on an analysis of abnormal traffic behavior. Int J Comput Netw Inf Secur. 2020;9(6):1. [Google Scholar]
89. Abdelrahman OH, Gelenbe E, Görbil G, Oklander B. Mobile network anomaly detection and mitigation: the NEMESYS approach. In: Gelenbe E, Lent R, editors. Information sciences and systems 2013. Cham: Springer; 2013. p. 429–438. doi:10.1007/978-3-319-01604-7_42. [Google Scholar] [CrossRef]
90. Kurata M, Ikami A, Itahara S, Suzuki M. Signaling storm mitigation by geographically distributed C-plane NF placement and routing. In: 2024 IEEE 10th International Conference on Network Softwarization (NetSoft), 2024; Saint Louis, MO, USA; p. 100–8. [Google Scholar]
91. Yu P, Deng Y, Zhang W, Yuan H, Mao A, Chen S. Uninterruptible IMS: maintaining the registration and call services during signaling storm in IP multimedia subsystem. In: Ning Z, Xiong Z, editors. Fifth International Conference on Computer Communication and Network Security (CCNS 20242024; Guangzhou, China: SPIE. doi:10.1117/12.3038087. [Google Scholar] [CrossRef]
92. Thubert P, Petrescu A, Wakikawa R, Devarapalli V. Network Mobility (NEMO) Basic Support Protocol RFC 3963. Available from: http://datatracker.ietf.org/doc/rfc3963/. [Accessed 2024]. [Google Scholar]
93. Khan MQ, Andresen SH. Pros and cons of route optimization schemes for network mobility and their implications on handovers. IEEJ Trans Electr Elect Eng. 2012;7(6):622–32. doi:10.1002/tee.v7.6. [Google Scholar] [CrossRef]
94. Khan MQ, Andresen SH, Khan KN. Pros and Cons of route optimization schemes for Network Mobility (NEMO) and their effects on handovers. In: Proceedings of the 8th International Conference on Frontiers of Information Technology, FIT ’10, 2010; New York, NY, USA: Association for Computing Machinery. doi:10.1145/1943628.1943652. [Google Scholar] [CrossRef]
95. Zhang B, Hu P, Azirani AA, Salahuddin MA, Barradas D, Limam N, et al. Secure and efficient group handover protocol in 5G non-terrestrial networks. In: ICC 2024-IEEE International Conference on Communications, 2024; Denver, CO, USA; p. 5063–8. [Google Scholar]
96. Gelenbe E, Abdelrahman OH, Gorbil G. Detection and mitigation of signaling storms in mobile networks. In: 2016 International Conference on Computing, Networking and Communications (ICNC), 2016; Kauai, HI, USA; p. 1–5. [Google Scholar]
97. Li B, Zhao S, Zhang R, Shi Q, Yang K. Anomaly detection for cellular networks using big data analytics. IET Commun. 2019;13(20):3351–9. doi:10.1049/iet-com.2019.0765. [Google Scholar] [CrossRef]
98. Chen TH, Chang JW, Wei HY. Dynamic inter-channel resource allocation for massive M2M control signaling storm mitigation. In: 2016 IEEE 84th Vehicular Technology Conference (VTC-Fall), 2016; Montreal, QC, Canada; p. 1–5. [Google Scholar]
99. Bradai A, Rehmani MH, Haque I, Nogueira M, Bukhari SHR. Software-Defined Networking (SDN) and Network Function Virtualization (NFV) for a hyperconnected world: challenges, applications, and major advancements. J Netw Syst Manage. 2020. doi:10.1007/s10922-020-09542-z. [Google Scholar] [CrossRef]
100. Yousaf FZ, Bredel M, Schaller S, Schneider F. NFV and SDN-key technology enablers for 5G networks. IEEE J Selected Areas Commun. 2017;35(11):2468–78. doi:10.1109/JSAC.2017.2760418. [Google Scholar] [CrossRef]
101. Barakabitze AA, Ahmad A, Mijumbi R, Hines A. 5G network slicing using SDN and NFV: a survey of taxonomy, architectures and future challenges. Comput Netw. 2020;167:106984. doi:10.1016/j.comnet.2019.106984. [Google Scholar] [CrossRef]
102. Madi T, Alameddine HA, Pourzandi M, Boukhtouta A. NFV security survey in 5G networks: a three-dimensional threat taxonomy. Comput Netw. 2021;197:108288. doi:10.1016/j.comnet.2021.108288. [Google Scholar] [CrossRef]
103. Ericsson. 5G to account for around 75 percent of mobile data traffic in 2029. Available from: https://www.ericsson.com/en/reports-and-papers/mobility-report/dataforecasts/mobile-traffic-forecast. [Accessed 2024]. [Google Scholar]
104. Ibrahim AAZ, Hashim F. An architecture of 5G based on SDN NV wireless network. Indones J Electr Eng Comput Sci. 2019;14(2):725–34. [Google Scholar]
105. Chowdhury MZ, Shahjalal M, Ahmed S, Jang YM. 6G wireless communication systems: applications, requirements, technologies, challenges, and research directions. IEEE Open J Commun Soc. 2020;1:957–75. doi:10.1109/OJCOMS. [Google Scholar] [CrossRef]
106. Alwis CD, Kalla A, Pham QV, Kumar P, Dev K, Hwang WJ, et al. Survey on 6G frontiers: trends, applications, requirements, technologies and future research. IEEE Open J Commun Soc. 2021;2:836–86. doi:10.1109/OJCOMS.2021.3071496. [Google Scholar] [CrossRef]
107. Coumar SO, Surender R. Efficient network resource management for improving radio access through machine learning approach in 5G networks. J Instit Eng (IndiaSeries B. 2024;105:1–9. [Google Scholar]
108. Hoffmann M, Kunzmann G, Dudda T, Irmer R, Jukan A, Macher G, et al. A secure and resilient 6G architecture vision of the german flagship project 6G-ANNA. IEEE Access. 2023;11:102643–60. doi:10.1109/ACCESS.2023.3313505. [Google Scholar] [CrossRef]
109. Parastar P, Lutu A, Alay Z, Caso G, Perino D. Spotlight on 5G: performance, device evolution and challenges from a mobile operator perspective. In: IEEE INFOCOM 2023-IEEE Conference on Computer Communications, 2023; New York, NY, USA; p. 1–10. [Google Scholar]
110. Kumar A, Thulajanaik B, Manjanaik N. An adaptive compression technique for efficient video reconstruction in future generation wireless network. Int J Intell Eng Syst. 2023;03, 16;3(16):497–505. [Google Scholar]
111. Oh I, Yoon Y, Sahlabadi M, Kim H, Yim K. Security elements and threat analysis of 5G mobile network based on 3GPP 5G NR standard. In: Barolli L, editor. Advances in intelligent networking and collaborative systems. Cham: Springer Nature Switzerland; 2024. p. 97–106. [Google Scholar]
112. Wani MS, Rademacher M, Horstmann T, Kretschmer M. Security vulnerabilities in 5G non-stand-alone networks: a systematic analysis and attack taxonomy. J Cybersecur Priv. 2024;4(1):23–40. doi:10.3390/jcp4010002. [Google Scholar] [CrossRef]
113. Salahdine F, Han T, Zhang N. Security in 5G and beyond recent advances and future challenges. Secur Priv. 2023;6(1):e271. doi:10.1002/spy2.271. [Google Scholar] [CrossRef]
114. Patil R, Tian Z, Gurusamy M, McCloud J. 5G core network control plane: network security challenges and solution requirements. Comput Commun. 2024;229:107982. [Google Scholar]
115. Liu G, Huang Y, Li N, Dong J, Jin J, Wang Q, et al. Vision, requirements and network architecture of 6G mobile network beyond 2030. China Commun. 2020;17(9):92–104. doi:10.23919/JCC.2020.09.008. [Google Scholar] [CrossRef]
116. Umoga UJ, Sodiya EO, Ugwuanyi ED, Jacks BS, Lottu OA, Daraojimba OD, et al. Exploring the potential of AI-driven optimization in enhancing network performance and efficiency. Magna Sci Adv Res Rev. 2024;10(1):368–78. doi:10.30574/msarr. [Google Scholar] [CrossRef]
117. Baz A, Logeshwaran J, Natarajan Y, Patel SK. Enhancing mobility management in 5G networks using deep residual LSTM model. Appl Soft Comput. 2024;165:112103. doi:10.1016/j.asoc.2024.112103. [Google Scholar] [CrossRef]
118. Abbasi M, Shahraki A, Taherkordi A. Deep learning for network traffic monitoring and analysis (NTMAa survey. Comput Commun. 2021;170:19–41. doi:10.1016/j.comcom.2021.01.021. [Google Scholar] [CrossRef]
119. Militano L, Zafeiropoulos A, Fotopoulou E, Bruschi R, Lombardo C, Edmonds A, et al. AI-powered infrastructures for intelligence and automation in beyond-5G systems. In: 2021 IEEE Globecom Workshops (GC Wkshps), 2021; Madrid, Spain; p. 1–6. [Google Scholar]
120. Abdalla AS, Upadhyaya PS, Shah VK, Marojevic V. Toward next generation open radio access networks: what O-RAN can and cannot do!. IEEE Netw. 2022;36(6):206–13. doi:10.1109/MNET.108.2100659. [Google Scholar] [CrossRef]
121. Afolabi I, Taleb T, Samdanis K, Ksentini A, Flinck H. Network slicing and softwarization: a survey on principles, enabling technologies, and solutions. IEEE Commun Surv Tut. 2018;20(3):2429–53. doi:10.1109/COMST.2018.2815638. [Google Scholar] [CrossRef]
122. Park HS, Kim H, Lee C, Lee H. Mobility management paradigm shift: from reactive to proactive handover using AI/ML. IEEE Netw. 2024;38(2):18–25. doi:10.1109/MNET.2024.3357108. [Google Scholar] [CrossRef]
Cite This Article
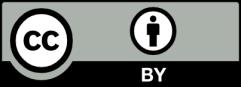
This work is licensed under a Creative Commons Attribution 4.0 International License , which permits unrestricted use, distribution, and reproduction in any medium, provided the original work is properly cited.