Open Access
REVIEW
Advancements in Liver Tumor Detection: A Comprehensive Review of Various Deep Learning Models
1 Deptartment of Artificial Intelligence and Data Science, Vardhaman College of Engineering, Hyderabad, 501218, India
2 Deptartment of CSE, V.S.B College of Engineering, Karur, 639111, India
3 Deptartment of Electrical and Electronics Engineering, Vardhaman College of Engineering, Hyderabad, 501218, India
4 Centre for Research Impact & Outcome, Chitkara University, Rajpura, 140401, Punjab, India
5 Department of Computer Science and Engineering, School of Engineering and Technology, Central University of Haryana, Mahendergarh, 123031, Haryana, India
6 Department of Computer Science and Information Engineering, National Yunlin University of Science and Technology, Yunlin, 64002, Taiwan
7 Intelligence Recognition Industry Service Research Center, National Yunlin University of Science and Technology, Yunlin, 64002, Taiwan
* Corresponding Author: Shih-Yu Chen. Email:
Computer Modeling in Engineering & Sciences 2025, 142(1), 91-122. https://doi.org/10.32604/cmes.2024.057214
Received 11 August 2024; Accepted 18 November 2024; Issue published 17 December 2024
Abstract
Liver cancer remains a leading cause of mortality worldwide, and precise diagnostic tools are essential for effective treatment planning. Liver Tumors (LTs) vary significantly in size, shape, and location, and can present with tissues of similar intensities, making automatically segmenting and classifying LTs from abdominal tomography images crucial and challenging. This review examines recent advancements in Liver Segmentation (LS) and Tumor Segmentation (TS) algorithms, highlighting their strengths and limitations regarding precision, automation, and resilience. Performance metrics are utilized to assess key detection algorithms and analytical methods, emphasizing their effectiveness and relevance in clinical contexts. The review also addresses ongoing challenges in liver tumor segmentation and identification, such as managing high variability in patient data and ensuring robustness across different imaging conditions. It suggests directions for future research, with insights into technological advancements that can enhance surgical planning and diagnostic accuracy by comparing popular methods. This paper contributes to a comprehensive understanding of current liver tumor detection techniques, provides a roadmap for future innovations, and improves diagnostic and therapeutic outcomes for liver cancer by integrating recent progress with remaining challenges.Keywords
Cite This Article
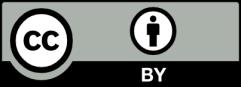
This work is licensed under a Creative Commons Attribution 4.0 International License , which permits unrestricted use, distribution, and reproduction in any medium, provided the original work is properly cited.