Open Access
ARTICLE
A Novel Self-Supervised Learning Network for Binocular Disparity Estimation
1 Department of Computer Science and Engineering, Major in Bio Artificial Intelligence, Hanyang University, Ansan-si, 15577, Republic of Korea
2 School of Electrical and Computer Engineering, Louisiana State University, Baton Rouge, LA 70803, USA
3 Department of Computer Engineering, College of Computer and Information Sciences, King Saud University, Riyadh, 11574, Saudi Arabia
4 School of Automation, University of Electronic Science and Technology of China, Chengdu, 610054, China
* Corresponding Authors: Bo Yang. Email: ; Wenfeng Zheng. Email:
Computer Modeling in Engineering & Sciences 2025, 142(1), 209-229. https://doi.org/10.32604/cmes.2024.057032
Received 06 August 2024; Accepted 11 October 2024; Issue published 17 December 2024
Abstract
Two-dimensional endoscopic images are susceptible to interferences such as specular reflections and monotonous texture illumination, hindering accurate three-dimensional lesion reconstruction by surgical robots. This study proposes a novel end-to-end disparity estimation model to address these challenges. Our approach combines a Pseudo-Siamese neural network architecture with pyramid dilated convolutions, integrating multi-scale image information to enhance robustness against lighting interferences. This study introduces a Pseudo-Siamese structure-based disparity regression model that simplifies left-right image comparison, improving accuracy and efficiency. The model was evaluated using a dataset of stereo endoscopic videos captured by the Da Vinci surgical robot, comprising simulated silicone heart sequences and real heart video data. Experimental results demonstrate significant improvement in the network’s resistance to lighting interference without substantially increasing parameters. Moreover, the model exhibited faster convergence during training, contributing to overall performance enhancement. This study advances endoscopic image processing accuracy and has potential implications for surgical robot applications in complex environments.Keywords
Cite This Article
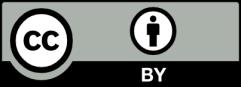
This work is licensed under a Creative Commons Attribution 4.0 International License , which permits unrestricted use, distribution, and reproduction in any medium, provided the original work is properly cited.