Open Access
REVIEW
Data-Driven Healthcare: The Role of Computational Methods in Medical Innovation
1 Department of Mechanical Engineering, Dr. Mahalingam College of Engineering and Technology, Pollachi, Coimbatore, Tamil Nadu, 642003, India
2 Department of Mechanical Engineering, JKKN College of Engineering and Technology, Kumarapalayam, Namakkal, Tamil Nadu, 637209, India
3 Department of Mechanical Engineering, Gharda Institute of Technology, Lavel, Maharashtra, 415708, India
4 Department of Electrical and Electronics Engineering, Sri Eshwar College of Engineering, Coimbatore, Tamilnadu, 641202, India
5 Department of Mechanical Engineering, Vel Tech Rangarajan Dr. Sagunthala R&D Institute of Science and Technology, Chennai, 600062, India
* Corresponding Author: Hariharasakthisudhan Ponnarengan. Email:
Computer Modeling in Engineering & Sciences 2025, 142(1), 1-48. https://doi.org/10.32604/cmes.2024.056605
Received 26 July 2024; Accepted 17 October 2024; Issue published 17 December 2024
Abstract
The purpose of this review is to explore the intersection of computational engineering and biomedical science, highlighting the transformative potential this convergence holds for innovation in healthcare and medical research. The review covers key topics such as computational modelling, bioinformatics, machine learning in medical diagnostics, and the integration of wearable technology for real-time health monitoring. Major findings indicate that computational models have significantly enhanced the understanding of complex biological systems, while machine learning algorithms have improved the accuracy of disease prediction and diagnosis. The synergy between bioinformatics and computational techniques has led to breakthroughs in personalized medicine, enabling more precise treatment strategies. Additionally, the integration of wearable devices with advanced computational methods has opened new avenues for continuous health monitoring and early disease detection. The review emphasizes the need for interdisciplinary collaboration to further advance this field. Future research should focus on developing more robust and scalable computational models, enhancing data integration techniques, and addressing ethical considerations related to data privacy and security. By fostering innovation at the intersection of these disciplines, the potential to revolutionize healthcare delivery and outcomes becomes increasingly attainable.Keywords
Computational models have emerged as powerful tools in several fields, including biomedical engineering, that enable researchers and practitioners to simulate complex biological processes and systems with significant precision [1,2]. Computational models are mathematical and algorithmic representations of real-world occurrences designed to predict behavior, understand underlying mechanisms, and guide experimental and clinical practices. Computational models influence the increasing computational power and sophisticated software algorithms to handle large datasets and intricate systems that are often challenging to study through traditional experimental methods. These models are essential to address questions related to complex biological systems, their interactions and behaviors [3]. However, challenges exist in ensuring the reliability and robustness of these models for biomedical applications [4]. In biomedical engineering, computational models enable cost- and time-efficient evaluations of fundamental hypotheses and parameter sensitivity studies, ultimately aiding in optimizing scaffold design in tissue engineering [5]. Additionally, mathematical models are used to improve the design of biomimetic devices, such as optimizing the construction of biomimetic models and understanding oxygen heterogeneities in microfluidic devices [6]. Different types of computational models used in biomedical engineering are shown in Fig. 1.
Figure 1: Computational models in biomedical engineering
In biomedical engineering, computational models are critical in advancing our understanding of physiological processes and disease mechanisms. They offer a framework to integrate diverse data types—from molecular and cellular levels to tissue and organ scales into coherent simulations that provide insights into biological functions and pathologies [7]. For example, computational models can simulate the heart’s electrical activity, predict the spread of infectious diseases, or optimize the design of medical devices [8]. These applications not only enhance our theoretical knowledge but also have practical implications for diagnostics, treatment planning, and the development of personalized medicine.
The development of computational models typically involves several key steps: defining the biological problem, formulating mathematical representations, implementing algorithms, validating models against experimental data, and refining models based on feedback. This iterative process ensures that the models are both accurate and reliable. Techniques such as finite element analysis [9–11], agent-based modeling, and machine learning [12,13] are commonly employed to build these models, each offering unique advantages depending on the specific application. The process of developing finite elements and machine learning-based computational models for biomedical applications is represented in Fig. 2.
Figure 2: Process of developing computational models
Despite their potential, computational models face challenges [14], including the need for high-quality data, computational resources, and integrating multi-scale and multi-disciplinary knowledge. However, with continuous advancements in computational technologies and interdisciplinary collaboration, the future of computational modeling in biomedical engineering promises even greater contributions to science and healthcare.
Computational models enable the analysis of physiological functions, disease progression, and treatment outcomes. Applications include personalized medicine through predictive modeling of patient responses to therapies [15], the development of medical devices with optimized designs [16], and virtual clinical trials that reduce the need for extensive human testing. Additionally, computational models aid in understanding genetic variations and their impacts on health, supporting drug discovery, and enhancing surgical planning through precise anatomical simulations [17]. These models significantly advance research, improve healthcare delivery, and foster innovations in biomedical technologies.
Bioinformatics is an interdisciplinary field that combines biology, computer science, and information technology to analyze and interpret biological data. It involves developing and applying computational tools and techniques for managing, processing, and understanding biological information, particularly at the molecular level [18]. This discipline is crucial for handling the vast amounts of data generated by genomic, proteomic, and other high-throughput experimental methods. Bioinformatics is critical in modern healthcare and biomedical research [19]. One of its primary applications is in genomics, which enables the analysis of DNA sequences to identify genetic variants associated with diseases [20]. This knowledge is fundamental for personalized medicine, where treatments are tailored to an individual’s genetic makeup, improving efficacy and reducing adverse effects. In drug discovery [21] and development, bioinformatics tools identify potential drug targets by analyzing biological pathways and protein structures. This accelerates the identification of new therapeutic compounds and the repurposing of existing drugs [22]. Additionally, bioinformatics aids in designing and optimizing clinical trials by stratifying patients based on genetic and molecular profiles, leading to more efficient and successful trials [23]. Proteomics, the large-scale study of proteins, also benefits from bioinformatics by enabling the analysis of protein expression, interactions, and functions. This information is critical to understanding the disease mechanisms and developing diagnostic biomarkers [24]. In transcriptomics, bioinformatics facilitates the analysis of RNA sequences to study gene expression patterns and regulatory mechanisms, providing insights into cellular functions and disease states. Furthermore, bioinformatics supports the integration and analysis of diverse types of biological data, such as epigenomic, metabolomic, and microbiome data, offering a holistic view of biological systems. This integrative approach is essential for advancing precision medicine, where comprehensive data analysis leads to more accurate diagnoses and personalized treatment strategies.
Machine learning (ML) is a subset of artificial intelligence that focuses on developing algorithms and statistical models that enable computers to perform tasks without explicit instructions. These models can identify patterns, make predictions, and improve over time by learning from data. The applications and impacts of machine learning in biomedical engineering are represented in Fig. 3. ML’s ability to process and analyze vast amounts of complex data makes it exceptionally well-suited for applications in biomedical engineering, where the integration of diverse and voluminous datasets is essential [25,26]. Machine learning is revolutionizing various domains in biomedical engineering, enhancing research, diagnostics, and treatment methodologies. One prominent application is in medical imaging [27], where ML algorithms detect and diagnose conditions from images such as X-rays, MRIs, and CT scans. These models can accurately identify anomalies, often surpassing human performance, leading to earlier and more reliable diagnoses [28]. Another critical area is genomics, where ML facilitates the analysis of genetic data to uncover the relationships between genetic variants and diseases. This capability is crucial for advancing personalized medicine, enabling the development of tailored treatments based on an individual’s genetic profile [29,30]. ML algorithms can predict disease risk, treatment responses, and potential side effects, significantly improving patient outcomes. In drug discovery, ML accelerates the identification of potential therapeutic compounds by analyzing biological data and predicting their efficacy and safety [31]. This reduces the time and cost associated with traditional drug development and increases the likelihood of successful clinical outcomes. ML models can also optimize clinical trial designs by identifying suitable patient cohorts and predicting trial outcomes [32]. Furthermore, ML is instrumental in developing wearable health technologies and mobile health applications. These devices use ML algorithms to monitor vital signs, detect real-time health issues, and provide personalized health recommendations [33]. This continuous monitoring and proactive health management enhance patient care and enable timely medical interventions.
Figure 3: Applications and impacts of machine learning in biomedical engineering
Integrating wearable technology into biomedical engineering represents a transformative development in the healthcare sector. The evolution of wearable devices is shown in Fig. 4. Wearable technology refers to electronic devices worn on the body, either as accessories or embedded into clothing, to monitor various health parameters and provide real-time data [34]. Biomedical engineering, which merges engineering principles with biological sciences, benefits immensely from these advancements. Wearable devices enable continuous, non-invasive monitoring of physiological signals such as heart rate, blood pressure, glucose levels, and even brain activity [35]. The integration process involves sophisticated design and engineering to ensure these devices are both functional and comfortable for daily use. Biomedical engineers work to develop sensors that are accurate, durable, and capable of wireless communication with other devices, such as smartphones or medical databases. This connectivity allows for the seamless transfer of health data, facilitating remote patient monitoring and telemedicine. Wearable technology also plays a crucial role in personalized medicine. Healthcare providers can tailor treatments and interventions more precisely by collecting and analyzing data unique to everyone. For example, wearable glucose monitors for diabetic patients provide continuous feedback on blood sugar levels, enabling more precise insulin dosing and better management of the condition. The integration of wearable technology in biomedical engineering extends to rehabilitation and physical therapy. Devices such as smart insoles or motion sensors can monitor a patient’s progress and adherence to prescribed exercises, providing valuable feedback to both patients and therapists [36].
Figure 4: Evolution of wearable devices in biomedical engineering
Wearable technology in healthcare is rapidly evolving, offering profound benefits. Currently, devices such as continuous glucose monitors and heart rate monitors facilitate real-time tracking of chronic diseases and enhance management efficacy. In preventive care, wearables provide early detection and intervention, with devices like electrocardiograms and ECG monitors that identify irregularities and prompt timely medical responses [37]. Future advancements include smart textiles and implantable devices, promising comprehensive health monitoring and targeted treatments. Integration with artificial intelligence and big data analytics enables predictive healthcare, revolutionizing personalized medicine. These advancements indicate a transformative era in healthcare, improving outcomes and reducing costs.
The interplay and synergy between bioinformatics and machine learning have revolutionized computational biomedical engineering. Mathematics provides vast biological datasets, while machine learning offers powerful algorithms for pattern recognition and predictive analytics. This integration decodes genomic sequences, identifies biomarkers, and elucidates protein structures, advancing precision medicine. Notable case studies include deep learning algorithms predicting cancer susceptibility and treatment outcomes, machine learning identifying drug targets, and models forecasting disease outbreaks. Despite these advancements, the field faces challenges such as data heterogeneity, privacy concerns, and the need for robust model validation.
Emerging trends and prospects in computational biomedical engineering are promising. Integrating artificial intelligence with multi-omics data advances in quantum computing and the rise of personalized medicine driven by precise genomic and phenotypic data are set to revolutionize healthcare. Blockchain technology for secure data sharing is also gaining traction. Addressing current challenges such as data integration, privacy, and translating computational findings into clinical practice remains critical. The future promises a seamless integration of computational tools with clinical workflows, fostering predictive, preventive, and personalized medicine, ultimately enhancing patient outcomes and deepening our understanding of complex biological systems. The present review outlines the research carried out in computational modeling in biomedical engineering by exploring bioinformatics, machine learning, and wearable technologies.
2 Computational Modelling in Biomedical Science
Computational modeling stands as a cornerstone in biomedical science, and it presents a virtual platform to simulate and analyze complex biological systems. This field leverages mathematical representations and sophisticated algorithms to emulate biological processes that offer often unattainable insights through traditional experimental methods. By harnessing the power of computational models, researchers can explore the dynamic interactions within cells, tissues, and organs, predict system behaviors under various conditions, and test hypotheses in silico before proceeding to costly and time-consuming laboratory experiments. The robustness of computational models lies in their ability to integrate data from diverse sources, enabling a holistic understanding of biological functions and disease mechanisms. This overview sets the stage for understanding how computational models are applied to various aspects of biomedical science, from cellular interactions to organ-level functions, ultimately facilitating advancements in medical research and clinical practice.
Building on the foundational role of computational modeling in biomedical science, the application of deterministic optimization algorithms further enhances the precision and efficiency of these models, as demonstrated in their superior ability to identify local and global optima in biological systems. Deterministic optimization algorithms using sensitivity equations, particularly when combined with a multistart strategy based on Latin hypercube sampling, outperform both stochastic methods and finite difference-based approaches in finding local and global optima. The study also provides an open-source software package for these algorithms, with outcomes including Epo receptor model parameters and time-course data for the JAK2/STAT5 model [38]. White et al. showed that using an ensemble of models improves prediction accuracy, with higher consensus among model outputs serving as a reliable indicator of confidence. Computational tests on a metabolic model of CHO cells demonstrate that ensemble predictions are more accurate than individual models, especially when consensus is high and variance is low [39]. Villaverde et al. introduced a methodology for generating high-confidence predictions from dynamic models by combining model parameters into meta-parameters and creating an ensemble of calibrated models. Computational tests on a CHO cell metabolic model showed that ensemble predictions are more accurate than individual models, with higher consensus among the ensemble linked to greater prediction accuracy [40]. Tiwary expressed that computational medicine holds great potential for understanding complex diseases and developing drugs with fewer side effects, especially through the use of network models. While network models have made significant advances in cancer research, similar approaches are needed for diseases like T2D and psychiatric disorders to improve diagnostics and treatment [41]. Walpole et al. found that multiscale models efficiently capture biological information across different scales by integrating continuous and discrete quantitative biomedical engineering methods to analyze data nonintuitively. They enable high-throughput hypothesis testing, quantify unmeasurable values, and translate findings to in vivo systems [42].
Bouteiller et al. created a multiscale modeling platform that links biomolecular models to multi-cellular networks, with a systematic validation approach at various levels to ensure accuracy. This iterative validation enhances the platform’s predictive power, enabling the study of how low-level changes affect higher-level functions [43]. Jacop et al. highlighted the effectiveness of combining computational and experimental approaches to study complex biological systems. Computational models successfully predicted the location and movement of key catalytic ions in large spliceosomal complexes, confirmed by cryoEM experiments [44]. Iris et al. suggested treating information as potentially unreliable and using a negative selection process to test and refine hypotheses systematically. They further concluded that modeling complex biological systems is a biology-driven challenge supported by computational sciences, complicated by the vast amount of biological data available [45].
The applications of computational modeling in understanding biological systems are vast and varied. At the cellular level, models simulate metabolic pathways, signal transduction networks, and gene regulatory circuits, providing insights into cellular behavior and response to stimuli [46,47]. At the organ and system levels, computational models aid in understanding physiological processes such as cardiovascular dynamics, respiratory functions, and neural activities. These models are instrumental in elucidating disease mechanisms, allowing researchers to investigate the progression of conditions like cancer, diabetes, and neurodegenerative diseases [48]. By simulating pathological conditions, computational models contribute to developing therapeutic strategies and optimizing treatment protocols [49,50]. Furthermore, these models facilitate the design and testing of biomedical devices, ensuring their efficacy and safety before clinical application. The ability to integrate multi-scale data from molecular interactions to whole-organism dynamics makes computational modeling an indispensable tool in the quest to decode the complexities of biological systems.
Cellular and molecular modeling focuses on the intricate details of cellular functions and molecular interactions. These models help decipher the complexities of cellular metabolism, gene expression, protein dynamics, and tumour growth [51]. For example, molecular dynamics simulations provide a detailed view of protein folding and enzyme-substrate interactions, as shown in Fig. 5 [52].
Figure 5: Molecular dynamics simulation reprinted with permission from Reference [52]. Copyright 2018 Hollingsworth et al.
Cellular models simulate the behavior of cell populations, such as tumor growth and immune response, offering valuable insights for cancer research and immunotherapy development. By bridging the gap between molecular and cellular levels, these models enhance our understanding of how molecular alterations translate into cellular phenotypes and contribute to disease progression [53,54]. Techniques such as molecular docking and Monte Carlo simulations allow researchers to predict the binding affinities and interactions between drugs and their targets, accelerating the drug discovery process [55]. Additionally, systems biology approaches integrate various omics data to build comprehensive models of cellular networks, enabling the prediction of cellular responses to different environmental and genetic perturbations [56].
Organ and system-level modeling extends the principles of computational modeling to larger biological structures. These models simulate the integrated functions of organs and systems, capturing the interactions between different tissues and their contributions to overall physiological processes. For instance, cardiac models simulate the electrical and mechanical activities of the heart, aiding in the study of arrhythmias and the design of cardiac devices [57], as shown in Fig. 6.
Figure 6: (a) Computational mesh (b) Simulation protocol (c) Simulation results in activation time reprinted with permission from Reference [57]. Copyright 2023 Fedele et al.
Respiratory models help understand lung mechanics and gas exchange, while neural models elucidate brain function and cognitive processes [58]. System-level models also play a crucial role in personalized medicine, enabling the prediction of individual treatment responses based on patient-specific data. Computational fluid dynamics (CFD) models are used to study blood flow in vascular systems, informing the design of stents and artificial heart valves [59–61], as shown in Fig. 7. Furthermore, multi-scale models that combine cellular and organ-level dynamics provide a comprehensive understanding of disease progression, such as the spread of cancer metastases or the development of chronic conditions like fibrosis [62,63].
Figure 7: Computational fluid dynamics (a) Boundary conditions (b) Results of velocity and pressure (LHA, RHA, PHA—Left, Right and proper hepatic artery) adapted from Reference [61]
Case studies highlight the practical applications and successes of computational modeling in biomedical science. One notable example is using computational models to study the spread of infectious diseases. During the COVID-19 pandemic, epidemiological models provided critical insights into the transmission dynamics of the virus, informing public health interventions and policy decisions [64,65]. Another example is using computational fluid dynamics (CFD) to optimize the design of medical devices, such as stents and prosthetic valves. These models simulate blood flow and mechanical forces, ensuring that devices function effectively within the human body [66–68]. In oncology, computational models have been used to simulate tumour growth and response to therapies, enabling the optimization of treatment regimens and the development of personalized medicine approaches [69,70]. Additionally, models of neural activity have been employed to study brain disorders such as epilepsy and Parkinson’s disease, guiding the development of targeted therapies and neuromodulation techniques [71,72]. These case studies underscore the transformative impact of computational modeling on biomedical research and clinical practice, demonstrating how virtual simulations can drive innovation and improve health outcomes.
3 Bioinformatics and Its Role in Biomedical Innovation
Bioinformatics represents the fusion of biology, computer science, and information technology, driving innovation in biomedical research and healthcare. This interdisciplinary field focuses on the development and application of computational tools to manage, analyze, and interpret biological data. With the advent of high-throughput technologies such as next-generation sequencing (NGS) as shown in Fig. 8, bioinformatics has become indispensable for handling the massive volumes of data generated [73,74]. By enabling the extraction of meaningful information from complex datasets, bioinformatics facilitates discoveries in genomics, proteomics, and systems biology, paving the way for personalized medicine and targeted therapies. The scope of bioinformatics extends from basic research, where it aids in understanding fundamental biological processes, to clinical applications, where it supports the diagnosis and treatment of diseases. This introduction sets the stage for understanding how bioinformatics transforms biological data into actionable insights that drive biomedical innovation.
Figure 8: Methods used for genome analysis and next generation sequencing adapted from Reference [74]
The arsenal of techniques and tools in bioinformatics is vast and continually evolving. Sequence analysis is a fundamental technique involving the alignment, comparison, and annotation of DNA, RNA, and protein sequences [75]. Tools such as BLAST (Basic Local Alignment Search Tool) and ClustalW are widely used for sequence alignment and similarity searches [76]. Structural bioinformatics focuses on predicting and analyzing macromolecular structures, employing tools like MODELLER for homology modeling and PyMOL for molecular visualization, as shown in the Fig. 9 [77]. Genomic and proteomic data analysis involves using software platforms such as Galaxy and Bioconductor to process and interpret large-scale datasets, identifying genes, variants, and biomarkers associated with diseases. Other critical techniques include phylogenetic analysis, which explores evolutionary relationships among organisms, and systems biology approaches that integrate various types of biological data to build comprehensive models of cellular and organismal functions [78]. These techniques and tools are essential for deciphering the complexity of biological systems and translating raw data into meaningful biological insights.
Figure 9: Molecular docking using PyMOL (a) 2D model (b) 3D model adapted from Reference [77]
Sequence analysis forms the backbone of bioinformatics, enabling the exploration of genetic information encoded in DNA and RNA. This technique involves comparing sequences to identify regions of similarity and divergence, inferring evolutionary relationships and functional annotations. Sequence alignment algorithms, such as Smith-Waterman and Needleman-Wunsch, facilitate the identification of homologous regions between sequences [79]. The annotation of genomes involves predicting coding regions, regulatory elements, and functional motifs, providing insights into gene function and regulation. Advances in sequence analysis have driven breakthroughs in genomics, such as the identification of disease-associated variants and the characterization of microbial communities. Moreover, comparative genomics, which involves the analysis of multiple genomes to understand evolutionary relationships and functional conservation, has provided deep insights into the genetic basis of complex traits and diseases. The development of databases such as GenBank and EMBL has facilitated the storage, retrieval, and analysis of sequence data, making it accessible to researchers worldwide [80].
Structural bioinformatics delves into biological macromolecules’ three-dimensional (3D) architecture, such as proteins and nucleic acids. Understanding the structure of these molecules is crucial for elucidating their functions and interactions. Techniques such as homology modeling, molecular docking, and molecular dynamics simulations are employed to predict and analyze macromolecular structures. Structural bioinformatics also aids in drug discovery by identifying binding sites and predicting the interactions between drugs and their targets [81,82]. Integrating structural data with functional and interaction data accelerates identifying therapeutic targets and designing novel drugs. For example, homology modeling allows researchers to predict the structure of a protein based on the known structures of related proteins, while molecular docking simulations predict how small molecules, such as potential drugs, interact with their protein targets. These techniques are complemented by experimental methods such as X-ray crystallography and NMR spectroscopy, which provide high-resolution structural data that can be used to validate and refine computational models.
Genomic and proteomic data analysis involves the comprehensive examination of organisms’ genetic and protein landscapes. Genomic analysis includes genome-wide association studies (GWAS), which identify genetic variants linked to diseases, and transcriptome analysis, which examines gene expression patterns [83]. Proteomic analysis involves the large-scale study of protein expression, interactions, and modifications. Techniques such as mass spectrometry and protein microarrays generate vast amounts of proteomic data, which are analysed using bioinformatics tools to identify biomarkers and understand disease mechanisms. Integrating genomic and proteomic data provides a holistic view of biological systems, advancing precision medicine. For instance, researchers can gain insights into the molecular pathways underlying disease development and progression by combining genomic data that identifies genetic predispositions to diseases with proteomic data that reveals protein expression changes. This integrated approach is essential for developing targeted therapies and personalized treatment strategies.
Bioinformatics is a driving force behind the advent of personalized medicine, where treatments are tailored to an individual’s genetic and molecular profile. Genomic sequencing allows the identification of genetic variants associated with diseases, enabling the development of targeted therapies. Bioinformatics tools facilitate the analysis of these variants, predicting their functional impact and guiding treatment decisions. In oncology, for instance, identifying cancer-specific mutations informs the selection of targeted therapies, improving treatment efficacy and reducing side effects. Integrating bioinformatics with clinical data also supports the stratification of patients in clinical trials, enhancing trial design and outcomes. Personalized medicine extends beyond oncology to other fields, such as cardiology, where genetic markers can predict responses to medications, and neurology, where genetic insights can guide the treatment of neurodegenerative diseases. The ability to tailor medical treatments to individual patients based on their genetic and molecular profiles represents a paradigm shift in healthcare, promising more effective and safer therapies.
Genomic sequencing has revolutionized our understanding of the genetic basis of diseases. High-throughput sequencing technologies, such as NGS, enable the rapid and cost-effective sequencing of entire genomes. Bioinformatics plays a crucial role in processing and interpreting the vast amounts of data generated, identifying genetic variants and predicting their functional impact. This information is essential for diagnosing genetic disorders, understanding disease mechanisms, and developing targeted therapies. In infectious diseases, genomic sequencing allows the characterization of pathogens, tracking their evolution and transmission dynamics, and informing public health strategies. For example, during the COVID-19 pandemic, genomic sequencing was used to track the spread of different virus variants, informing containment measures and vaccine development [84]. In rare genetic disorders, sequencing the genomes of affected individuals and their families can identify causative mutations, leading to accurate diagnoses and the development of personalized treatment plans.
Tailored therapeutic approaches represent the pinnacle of personalized medicine, where treatments are customized based on an individual’s genetic and molecular profile. Bioinformatics facilitates the identification of biomarkers and therapeutic targets, enabling the development of precision therapies [85]. For example, in cancer treatment, the analysis of tumor genomes reveals mutations that drive cancer progression, guiding the selection of targeted therapies. Similarly, pharmacogenomics studies how genetic variations influence drug response, informing the choice and dosage of medications. By aligning treatment strategies with an individual’s unique biological characteristics, tailored therapeutic approaches enhance efficacy and minimize adverse effects, improving patient outcomes. This approach also involves the development of companion diagnostics tests that identify the presence of specific biomarkers that predict response to treatments. By integrating genetic, proteomic, and clinical data, tailored therapeutic approaches ensure that patients receive the most appropriate and effective treatments, paving the way for a new era of precision medicine.
The quantitative results from key bioinformatics and computational studies in biomedical research are represented in Table 1. The Response Database Initiative (RDI) system changed 33% of treatment decisions made by experienced HIV physicians, with the alternative regimens predicted to have better virologic responses and use fewer drugs. Physicians rated the system as easy to use, helpful, and likely to be used frequently in future decisions [86]. Drusbosky et al. showed that computational modeling and digital drug simulations accurately predict treatment responses in myelodysplastic syndromes (MDS) and can be used for pre-clinical testing of new drugs. These models have shown clinical relevance by serving as substitutes for cell lines in MDS research [87]. Cook et al. predicted Transforming Growth Factor β (TGFβ) inhibition by a computational hybrid cellular automaton (HCA) model to significantly reduce prostate cancer cell viability, osteoclast formation, and osteoblast differentiation in bone metastases. These in silico predictions were validated through in vivo experiments using animal models of bone metastatic prostate cancer [88]. Niederer et al. developed computational models in cardiology to integrate patient-specific data to create virtual simulations, revealing hidden diagnostic information and predicting treatment outcomes. This approach drives the development of personalized treatment methods through advanced modeling tools and techniques [89]. Winslow et al. expressed that computational medicine utilizes models to describe molecular networks, physiology, and anatomy to enhance personalized therapies. Statistical learning and computational approaches are applied to biomolecular data and anatomical variations to understand disease mechanisms and improve treatment outcomes [90]. Ogilvie et al. discussed that mechanistic computational modeling, like the ModCell™ platform, is used to create predictive models of tumor, patient, and drug interactions in oncology. Based on molecular data, virtual patient models enhance personalized medicine and targeted drug development by predicting individual treatment responses [91].
De Bruijn et al. have expressed that the genetic diagnostic yield for inherited hearing loss and retinal dystrophy varies between 40%–70%, which highlights the need for improved sequencing and analysis techniques. Advancements in long-read sequencing, optical mapping, and bioinformatics tools offer opportunities to investigate noncoding regions and structural variations, enhancing disease mechanism understanding and diagnostic accuracy [92]. Gilissen et al. identified new Mendelian disease genes through exome sequencing in about 60% of projects, with continued advancements in bioinformatics and sequencing technology expected to increase this success rate. As these improvements progress, exome sequencing is poised to become the primary tool for Mendelian disease gene identification [93]. Torkamani et al. identified that the typical human genome contains 5.5–6.1 million variants, with around 12,000 likely having functional effects, and the rate of functional variants varies significantly between populations. These findings have crucial implications for clinical sequencing efforts and personalized medicine [94]. Londin et al. expressed that the linkage analysis and genome-wide studies have advanced our understanding of the genetic architecture of diseases, but gaps remain. Next-generation sequencing has significantly improved the study of genetic sequences, complementing genome-wide association studies (GWAS) to better identify disease-causing variants and expand knowledge of genetic diseases [95].
4 Machine Learning in Medical Diagnostics
Machine learning (ML), a subset of artificial intelligence, is revolutionizing healthcare by enabling the analysis of complex medical data to derive actionable insights [96]. ML algorithms learn from data, identifying patterns and making predictions without explicit programming. This capability is particularly valuable in healthcare, where vast amounts of data are generated from clinical records, medical imaging, genomic studies, and wearable devices [97]. By processing and analyzing these datasets, ML enhances diagnostic accuracy, predicts disease outcomes, and informs treatment decisions, contributing to improved patient care and healthcare efficiency [98]. The applications of ML span various domains, including personalized medicine, drug discovery, and public health, making it a cornerstone of modern biomedical research and clinical practice [99]. This overview sets the stage for understanding the transformative impact of ML in healthcare, emphasizing its role in driving innovation and improving health outcomes.
The development of diagnostic algorithms is one of the most significant contributions of ML to healthcare. These algorithms analyze clinical data to identify patterns indicative of diseases, aiding in early diagnosis and treatment planning [100]. Techniques such as supervised learning, unsupervised learning, and reinforcement learning are employed to build diagnostic models. Supervised learning algorithms, such as support vector machines (SVM), decision trees, and neural networks, are trained on labelled datasets to recognize disease patterns [101]. These algorithms learn from examples of diagnosed cases, developing the ability to predict the presence of diseases in new, unseen cases. Unsupervised learning techniques, like clustering and dimensionality reduction, uncover hidden structures in data, identifying novel disease subtypes and biomarkers [102]. For example, clustering algorithms can group patients with similar symptoms or genetic profiles, facilitating the identification of previously unrecognized disease subtypes. Reinforcement learning, which involves learning optimal actions through trial and error, has potential applications in personalized treatment planning and adaptive clinical decision support systems [103].
Medical imaging is a critical area where ML has demonstrated a substantial impact. A general workflow of medical imaging is shown in Fig. 10 [104]. ML algorithms analyze medical images, such as X-rays, MRIs, and CT scans, to detect abnormalities with high precision. Convolutional neural networks (CNNs), a type of deep learning model, excel in image recognition tasks, identifying features that may be imperceptible to the human eye. These models have been successfully applied in diagnosing conditions like tumors, fractures, and neurological disorders [105]. By automating image analysis, ML reduces the workload on radiologists, speeds up diagnosis, and improves the accuracy of interpretations [106]. For instance, CNNs can be trained to recognize malignant tumors in mammograms, providing a second opinion that assists radiologists in making accurate diagnoses [107]. Additionally, ML models are used to segment medical images, delineate anatomical structures and identify regions of interest, which is crucial for planning surgical procedures and monitoring disease progression [108]. The integration of ML in medical imaging holds promise for enhancing diagnostic precision, reducing human error, and enabling earlier detection of diseases [109].
Figure 10: General workflow of medical imaging. Adapted from Reference [104]
Predictive modeling is another key application of ML in medical diagnostics. By analyzing historical patient data, ML models can predict the likelihood of disease onset, progression, and response to treatment by developing models as shown in Fig. 11 [110]. These predictions enable healthcare providers to implement preventive measures, personalize treatment plans, and improve patient outcomes. For instance, ML models can predict the risk of chronic diseases such as diabetes, cardiovascular diseases, and cancer-based on genetic, demographic, and lifestyle factors [111]. Early predictions allow for timely interventions, potentially reducing the incidence and severity of these conditions. Predictive modeling is also used in hospital readmission prediction, identifying patients at high risk of readmission and allowing for targeted interventions to prevent it [112]. Moreover, predictive models can forecast the outbreak of infectious diseases by analyzing epidemiological data and supporting public health efforts in disease control and prevention [113]. The ability of ML to integrate and analyze vast amounts of data from diverse sources makes it an invaluable tool for predictive modeling, enhancing the capacity to anticipate and mitigate health risks [114,115].
Figure 11: Disease prediction model using laboratory test. Adapted from Reference [110]
The impact of ML in medical diagnostics is evidenced by numerous success stories and case studies. For example, Google’s DeepMind developed an ML algorithm that accurately diagnoses eye diseases from retinal scans, potentially preventing blindness in millions of patients [116]. Similarly, IBM Watson’s oncology platform uses ML to analyze clinical and research data, providing oncologists with evidence-based treatment recommendations [117]. Another notable example is the use of ML in detecting diabetic retinopathy, where deep learning models have achieved high accuracy in identifying this condition from retinal images, enabling early intervention and treatment [118]. These applications demonstrate the transformative potential of ML in improving diagnostic accuracy, enhancing patient care, and advancing medical research. In cardiology, ML models have been developed to predict the occurrence of arrhythmias from electrocardiogram (ECG) data, allowing for timely interventions that can prevent serious complications [119]. These case studies underscore the practical benefits of ML in real-world clinical settings, highlighting its role in driving innovation and improving health outcomes.
ML’s ability to quickly and accurately analyze vast amounts of data makes it invaluable for early disease detection. For example, in the context of infectious diseases, ML models can analyze epidemiological data to predict outbreaks and track the spread of diseases like COVID-19. Early detection and intervention can significantly mitigate the impact of such diseases on public health [120]. Furthermore, ML algorithms are used in genetic testing to identify individuals at high risk for hereditary conditions, enabling proactive monitoring and preventive care. For instance, ML models can analyze genetic data to identify mutations associated with increased risk of breast cancer, allowing for early surveillance and preventive measures [121]. In oncology, ML algorithms analyze patterns in medical images to detect early-stage tumors that might be missed by human observers, facilitating timely treatment and improving survival rates [122]. The ability to detect diseases at an early stage, when they are most treatable, underscores the critical role of ML in preventive healthcare and its potential to save lives through early intervention.
The integration of ML in medical diagnostics has led to significant improvements in diagnostic accuracy. By learning from large datasets, ML models can identify subtle patterns and correlations that may be overlooked by human practitioners. This enhanced accuracy is particularly valuable in complex and rare diseases where traditional diagnostic methods may fall short [123]. For instance, ML models have been used to diagnose rare genetic disorders by analyzing whole-exome sequencing data, providing accurate diagnoses that guide appropriate treatment [124]. In radiology, ML algorithms improve the detection of subtle abnormalities in medical images, enhancing the diagnostic accuracy of conditions such as lung cancer, brain tumors, and cardiovascular diseases [125]. Moreover, ML models can continuously learn and improve from new data, adapting to emerging trends and ensuring up-to-date diagnostic capabilities. As ML continues to evolve, its applications in medical diagnostics are expected to expand, further enhancing the precision and effectiveness of healthcare delivery. The ability of ML to provide consistent, reproducible, and high-accuracy diagnoses represents a significant advancement in medical diagnostics, contributing to better patient outcomes and more efficient healthcare systems [126].
The quantitative insights from recent machine learning and collaborative research in healthcare and neuroscience are shown in Table 2. Huang et al. used analytically derived distributions (ADDs) to evaluate the accuracy of machine learning metrics like sensitivity, specificity, and Area Under the Receiver Operating Characteristic (AUROC). It compares ADDs to simulation-based approaches, highlighting their effectiveness in assessing model performance metrics [127]. Sidey-Gibbons et al. studied machine learning models, including regularized General Linear Model regression, Support Vector Machines, and single-layer Artificial Neural Networks, and achieved high accuracy, sensitivity, and specificity in classifying cell nuclei from breast masses. Combining these models into a voting ensemble slightly improved predictive performance, offering a practical introduction to machine learning for medical researchers [128]. Samuelson evaluated the diagnostic performance of new imaging devices, focusing on sensitivity, specificity, and Area Under the Curve (AUC) as key metrics. A positive change in AUC indicates improved diagnostic information for radiologists, while variations in sensitivity and specificity are expected due to differing clinical practices [129]. Erickson et al. expressed that machine learning is a powerful tool for medical image analysis, focusing on computing image features and selecting the best combination for classification or metric computation. Various machine learning methods, including open-source options, offer different strengths and weaknesses, requiring careful application for accurate results [130]. Müller et al. identified issues like statistical biases and incorrect use of evaluation metrics in current medical image segmentation model assessments, particularly in handling class imbalance. They proposed guidelines to standardize evaluation methods, improving the quality, reproducibility, and comparability of model performance across studies [131]. Ahmad found that deep learning algorithms showed higher sensitivity but lower specificity than radiology experts in detecting abnormalities. Sensitivity was slightly higher for the algorithms, with a statistically significant difference, while their specificity varied depending on the comparison [132]. Balki et al. reviewed methodologies for determining the required training sample size for machine learning models in medical imaging, identifying pre-hoc model-based and post-hoc curve-fitting approaches but noting a lack of standardization. They empathized with the need to develop streamlined sample size determination methods to improve model performance in medical imaging research [133].
5 Wearable Technology and Real-Time Health Monitoring
The evolution of wearable health devices has been driven by advancements in sensor technology, miniaturization, and wireless communication and categorized majorly as shown in Fig. 12 [134]. Early wearable devices, such as pedometers and heart rate monitors, provided basic health metrics. These devices were primarily used by athletes and fitness enthusiasts to monitor physical activity and optimize training regimens [135]. However, over the past decade, wearable technology has evolved dramatically, resulting in sophisticated devices capable of real-time monitoring of a wide range of physiological parameters [136]. Modern wearables include smartwatches, fitness trackers, continuous glucose monitors, and even smart clothing embedded with sensors. These devices can monitor heart rate variability, oxygen saturation, sleep patterns, and stress levels, among other metrics [137]. The integration of wearable devices with smartphones and cloud-based platforms has enabled seamless data collection, storage, and analysis, enhancing their utility in health monitoring and management. This evolution has been facilitated by advancements in microelectronics, flexible materials, and energy-efficient wireless communication protocols [138]. As a result, wearable devices have become more accurate, user-friendly, and accessible to a broader population, transforming how individuals manage their health and well-being.
Figure 12: Major categories of personal wearable devices. Adapted from Reference [134]
The integration of wearable technology with computational methods has revolutionized health monitoring. Wearable devices continuously collect data on various health metrics, which are then processed and analyzed using advanced computational techniques [139]. Machine learning algorithms are pivotal in analyzing wearable data, identifying patterns, and providing actionable insights. For example, algorithms can detect anomalies in heart rate or sleep patterns, alerting users and healthcare providers to potential health issues [140]. The integration of computational methods with wearables not only enhances real-time monitoring but also facilitates personalized health interventions. Data from wearables can be integrated with electronic health records (EHRs) to provide a comprehensive view of an individual’s health status, enabling personalized treatment plans and early interventions [141]. Furthermore, the use of cloud computing allows for the storage and processing of large volumes of data, enabling continuous monitoring and real-time feedback. Predictive analytics and artificial intelligence (AI) can be applied to wearable data to forecast health trends and recommend preventive measures, thus enhancing overall health management [142].
Wearable devices generate vast amounts of data, necessitating robust methods for data collection and analysis. Data from wearables includes physiological metrics such as heart rate, blood pressure, glucose levels, and physical activity. Advanced data processing techniques, including signal processing and machine learning, are employed to clean, analyze, and interpret this data [143]. Wearable data analytics provide valuable insights into an individual’s health status, lifestyle, and behavior, enabling personalized recommendations for health improvement. The continuous collection and analysis of wearable data also supports longitudinal studies, enhancing our understanding of health trends and disease progression over time [144]. Additionally, data from wearables can be used to monitor adherence to treatment regimens and assess the effectiveness of interventions. The integration of wearable data with other health data sources, such as genetic and clinical data, allows for a comprehensive analysis of health determinants, leading to more accurate and holistic health assessments [145]. Privacy and security considerations are paramount in wearable data collection, requiring robust encryption and data protection measures to ensure that sensitive health information is safeguarded [146].
Real-time monitoring systems leverage wearable technology to provide continuous, real-time health monitoring. These systems are particularly valuable for managing chronic conditions such as diabetes, hypertension, and cardiovascular diseases [147]. For instance, continuous glucose monitors (CGMs) track blood sugar levels throughout the day, providing diabetic patients with real-time feedback and helping them manage their condition more effectively [148,149]. Similarly, wearable ECG monitors detect irregular heart rhythms, enabling early intervention in cases of arrhythmia [150]. Real-time monitoring systems empower individuals to take proactive control of their health, reducing the risk of complications and improving overall well-being. These systems also facilitate remote patient monitoring, allowing healthcare providers to track patients’ health status from a distance and intervene when necessary. This is particularly beneficial for elderly patients and those with mobility issues, as it reduces the need for frequent hospital visits and enhances their quality of life [151]. Moreover, real-time monitoring systems can be integrated with telemedicine platforms, enabling virtual consultations and remote diagnostics, thus expanding access to healthcare services [152,153].
The applications of wearable technology in healthcare are extensive and transformative. Wearables are used for fitness tracking, chronic disease management, remote patient monitoring, and preventive healthcare [154]. In fitness tracking, devices monitor physical activity, sleep, and other health metrics, motivating individuals to adopt healthier lifestyles. For chronic disease management, wearables provide continuous monitoring, enabling timely interventions and better disease control. Remote patient monitoring through wearables reduces the need for frequent hospital visits, enhancing patient convenience and reducing healthcare costs [155]. In preventive healthcare, wearables facilitate early detection of potential health issues, allowing for timely intervention and reducing the burden on healthcare systems. Wearable technology also plays a crucial role in clinical trials by providing continuous and objective data on participants’ health status and treatment responses. This leads to more accurate and reliable trial outcomes, accelerating the development of new therapies and medical devices [156]. Furthermore, wearables can be used in workplace wellness programs to monitor employees’ health and promote a healthier work environment.
Wearable technology plays a crucial role in chronic disease management. Devices such as CGMs, wearable blood pressure monitors, and smart inhalers provide continuous monitoring and real-time feedback for conditions like diabetes, hypertension, and asthma. This continuous monitoring enables patients to manage their conditions more effectively, reducing the risk of complications and hospitalizations [157]. Wearables also support telemedicine, allowing healthcare providers to monitor patients remotely and adjust treatment plans based on real-time data. This remote management improves patient outcomes and enhances the efficiency of healthcare delivery [158]. For example, CGMs enable diabetic patients to continuously track their glucose levels and adjust their diet, exercise, and medication accordingly. Wearable blood pressure monitors provide hypertensive patients with real-time data on their blood pressure, helping them manage their condition and avoid hypertensive crises [159]. Smart inhalers for asthma patients track inhaler usage and environmental triggers, ensuring optimal medication adherence and reducing the risk of asthma attacks [160]. The integration of wearable technology with mobile health applications further enhances chronic disease management by providing patients with personalized insights and recommendations based on their real-time data.
In preventive healthcare, wearable devices offer significant benefits by facilitating early detection and intervention. For instance, wearable ECG monitors can detect early signs of cardiovascular issues, prompting timely medical intervention and potentially preventing serious complications [161]. Fitness trackers encourage physical activity and healthy lifestyle choices, reducing the risk of chronic diseases such as obesity, diabetes, and cardiovascular diseases. By providing continuous health monitoring and personalized feedback, wearables empower individuals to take proactive steps toward maintaining their health, ultimately reducing the incidence and impact of chronic diseases [162]. Wearable technology also supports population health management by providing aggregated data on health trends and risk factors, enabling public health officials to design targeted interventions and health promotion programs [163]. Moreover, wearable devices can be used in screening programs for conditions such as sleep apnoea, atrial fibrillation, and osteoporosis, identifying individuals at risk and facilitating early diagnosis and treatment. The integration of wearable data with AI and predictive analytics further enhances preventive healthcare by identifying emerging health risks and recommending personalized preventive measures [164].
The quantitative outcomes of AI and wearable health monitoring systems are shown in Table 3. Teng et al. expressed that wearable medical systems allow continuous physiological monitoring, enabling early disease detection and timely intervention. The study further highlighted key advances in technologies such as measurement, motion artifact reduction, energy harvesting, and networks, with examples of clinical applications for improved healthcare outcomes [165]. Babu et al. identified that wearable devices enable non-invasive, continuous monitoring of physiological parameters, offering affordable and sensitive solutions for health tracking. These advancements have the potential to revolutionize the early detection, diagnosis, and management of various clinical conditions [166]. Sharma et al. found that wearable biosensors enable continuous monitoring of critical biomarkers in biological fluids, which are increasingly used in healthcare. Advances in multiplexed biosensing, microfluidic sampling, and flexible materials have improved their functionality, but cohort validation studies are necessary for clinical acceptance [167]. Yin et al. demonstrated the feasibility of a hierarchical health decision support system integrating wearable medical sensor (WMS) data into clinical decision support systems (CDSS), achieving high diagnostic accuracy for diseases such as arrhythmia (86%), type-2 diabetes (78%), and urinary bladder disorder (99%). The authors estimated that storing diagnostic modules for all 69,000 known diseases in the WMS tier would require only 62 GB, making it practical for current systems [168]. Koydemir et al. found that wearable and implantable technologies are advancing healthcare by enabling real-time health management and tracking, contributing to improved outcomes. The authors have reviewed biomedical applications, materials, and standards while addressing technical challenges and proposed potential solutions [169]. El-Amrawy et al. found that wearable devices varied in accuracy and precision for tracking step counts and heart rate, with some (e.g., Apple Watch, MisFit Shine) showing high accuracy, while others (e.g., Samsung Gear 2, Jawbone UP) performed less accurately. Despite these variations, the devices were generally effective in tracking physical activity and heart rate, offering potential clinical benefits for improving health outcomes like obesity and heart disease [170].
6 Synergy and Innovation at the Intersection
The convergence of computational engineering and biomedical science has led to numerous successful integrations, driving innovation in healthcare [171]. One notable case study is the development of personalized cancer treatment strategies. By integrating genomic sequencing data with computational models, researchers have been able to identify cancer-driving mutations and tailor therapies to individual patients [172], as shown in Fig. 13. This approach has significantly improved treatment outcomes and reduced adverse effects. For instance, The Cancer Genome Atlas (TCGA) project has leveraged computational models to analyze vast amounts of genomic data, identifying key mutations and pathways involved in various cancers [173]. These insights have led to the development of targeted therapies that are personalized to the genetic profile of each patient’s tumor, thereby increasing the efficacy of treatments and minimizing toxicities.
Figure 13: Personalized chemotherapy for breast cancer using predictive models. Adapted from Reference [172]
Another successful integration is using machine learning algorithms to analyze medical imaging data, enhancing diagnosis accuracy and enabling early disease detection [174,175]. Deep learning models, such as convolutional neural networks (CNNs), have been employed to identify patterns in medical images indicative of diseases like cancer, cardiovascular disorders, and neurological conditions, as shown in Fig. 14 [176]. These models have demonstrated diagnostic accuracies that rival or even surpass those of experienced radiologists. For example, Google’s DeepMind has developed an algorithm that can accurately detect over eye diseases from retinal scans, potentially preventing vision loss in millions of patients [177].
Figure 14: Deep convolutional neural network in prostate cancer diagnosis. Adapted from Reference [176]
In addition to these examples, computational models have been used to simulate the spread of infectious diseases, such as during the COVID-19 pandemic. Epidemiological models provided critical insights into transmission dynamics, informing public health interventions and policy decisions. These models have been instrumental in predicting the impact of various containment strategies, such as social distancing and vaccination campaigns, thereby aiding governments and health organizations in making informed decisions to control the spread of the virus [178]. Personalized medicine represents one of the most significant breakthroughs at the intersection of computational engineering and biomedical science. By leveraging computational tools to analyze genetic, molecular, and clinical data, personalized medicine aims to tailor treatments to individual patients. Advances in genomic sequencing, bioinformatics, and machine learning have enabled the identification of genetic variants associated with diseases, guiding the development of targeted therapies [179,180].
For example, in oncology, the use of next-generation sequencing (NGS) allows for the comprehensive analysis of tumor genomes, identifying mutations that drive cancer progression. These genetic insights inform the selection of targeted therapies that specifically inhibit the activity of mutated proteins, improving treatment efficacy and reducing side effects [181]. Implementing computational models that predict tumor behavior and drug response has further refined these treatment strategies, enabling oncologists to personalize therapies based on the unique genetic makeup of each patient’s tumor [182]. In the realm of pharmacogenomics, personalized medicine has made significant strides by tailoring drug prescriptions based on an individual’s genetic profile. By analyzing genetic variants that affect drug metabolism, efficacy, and toxicity, computational models can predict how patients will respond to specific medications [183]. This approach minimizes adverse drug reactions and optimizes therapeutic outcomes, particularly for complex diseases like depression, cardiovascular disorders, and autoimmune diseases. Moreover, personalized medicine extends to the prevention and early detection of diseases. Predictive models that integrate genetic, lifestyle, and environmental data can identify individuals at high risk for conditions such as diabetes, cardiovascular diseases, and certain cancers. This enables the implementation of personalized prevention strategies, such as lifestyle modifications and regular screenings, to mitigate disease risk and improve long-term health outcomes [184].
The integration of computational and biomedical sciences has led to significant improvements in health outcomes. For example, using computational models to simulate cardiac function has improved the design of pacemakers and other cardiac devices, enhancing their efficacy and patient outcomes [185]. Computational fluid dynamics (CFD) models simulate blood flow within the heart and blood vessels, enabling the optimization of device designs to ensure proper function and minimize complications such as thrombosis and mechanical failure. These advancements have translated into better patient outcomes, with improved device performance and longer-lasting solutions for cardiac conditions [186]. In the field of infectious diseases, computational models have been used to predict the spread of epidemics, inform public health interventions, and reduce the impact of outbreaks. During the COVID-19 pandemic, models that integrated data on virus transmission, population movement, and public health measures provided critical insights into the dynamics of the outbreak. These models guided the implementation of containment strategies, such as lockdowns, social distancing, and vaccination campaigns, significantly reducing infection rates and saving lives [187].
Additionally, integrating wearable technology with computational methods has enabled continuous health monitoring, leading to early detection of health issues and timely interventions. Wearable devices that monitor vital signs, physical activity, and physiological parameters generate vast amounts of data that are analyzed in real time by machine learning algorithms. These algorithms detect deviations from normal patterns, alerting users and healthcare providers to potential health issues before they become critical [188]. For instance, wearable ECG monitors can detect early signs of arrhythmias, allowing for prompt medical intervention and preventing serious cardiac events. Similarly, continuous glucose monitors (CGMs) provide real-time feedback to diabetic patients, enabling better blood sugar control and reducing the risk of complications [189].
In neurology, computational models have enhanced the understanding and treatment of neurological disorders. For example, models that simulate neural activity and brain networks have been used to develop and optimize deep brain stimulation (DBS) therapies for conditions such as Parkinson’s disease and epilepsy. By accurately targeting specific brain regions and adjusting stimulation parameters, these models have improved the efficacy of DBS, leading to better symptom management and improved quality of life for patients [190].
Heller et al. found that multidisciplinary collaborations are seen as crucial to research success by 71% of academic surgeons. PhD researchers (81%) are more involved in these collaborations compared to MD/MD-PhD researchers (27%). The key benefits include increased publications, research opportunities for surgical residents, and National Institutes of Health funding [191]. Mike et al. reported that the electrical engineering students working on biomedical signal processing projects lack the essential medical expertise required to achieve solutions applicable by physicians. This knowledge gap is only acknowledged by students in the advanced phases of the project, leading to issues in the critical phases of goal setting and planning [192]. Spira et al. have expressed that the computational biomedicine section was created to advance interdisciplinary computational approaches to clinical problems, combining expertise in analytics, clinical work, and bioinformatics. This initiative has boosted research and training capacity and facilitated productive industry collaborations [193]. Cassel et al. stated that interdisciplinary computing spans diverse fields like art, science, and engineering, requiring collaboration between computing specialists and experts from other areas. While some institutions support this, others face barriers such as limited resources and motivation [194]. Golecki et al. acknowledged that implementing an interdisciplinary curriculum can be challenging due to differences in perspectives, constraints of the education system, and lack of supporting frameworks [195]. Munshi et al. concluded that advances in biomedical research require researchers skilled in both life and physical sciences, as well as computational, mathematical, and engineering tools to develop computational models to solve the issues in biomedical engineering [196].
7 Challenges and Ethical Considerations
Despite the significant advancements at the intersection of computational engineering and biomedical science, several technical challenges remain. One major challenge is the need for high-quality, large-scale datasets to train and validate computational models. Data quality, completeness, and standardization are critical for the accuracy and reliability of these models. In many cases, biomedical data is fragmented, coming from diverse sources with varying formats and standards. Integrating such heterogeneous data into a coherent and usable form presents substantial technical difficulties. Additionally, the sheer volume of biomedical data, often referred to as “big data,” necessitates advanced storage, processing, and analytical capabilities [197].
Computational resource requirements, including processing power and storage capacity, also represent a barrier to the widespread adoption of advanced computational techniques in biomedical research. High-performance computing (HPC) environments and cloud computing platforms are often required to handle the computational load, which can be cost-prohibitive and logistically challenging for many research institutions [198]. Moreover, ensuring the robustness and generalizability of computational models poses a significant challenge. Models trained on specific datasets may not perform well when applied to different populations or conditions, leading to model transferability and applicability issues in diverse clinical settings. This necessitates rigorous validation and continuous updating of models to ensure they remain accurate and relevant [199].
The scalability of computational models is another critical challenge. As biomedical data grows in volume and complexity, models must be scalable to handle large datasets efficiently. This requires advancements in computational algorithms, parallel processing, and distributed computing. Techniques such as distributed machine learning and federated learning are being explored to address scalability issues, allowing models to be trained on decentralized data sources without compromising data privacy and security [200]. Ensuring that models can scale without compromising accuracy and performance is essential for their practical application in clinical settings. Scalable models must be capable of processing and analyzing real-time data from various sources, such as electronic health records (EHRs), genomic databases, and wearable devices. This involves not only computational efficiency but also the ability to integrate and harmonize data from different modalities and formats [201].
Additionally, the development of user-friendly tools and platforms that allow researchers and clinicians to leverage computational models without requiring extensive technical expertise is crucial for broader adoption. These tools should facilitate the easy deployment, monitoring, and updating of models in clinical workflows, ensuring they remain effective and relevant as new data becomes available [202].
Data integration is a fundamental requirement for successfully applying computational models in biomedical science. Integrating diverse data types, such as genomic, proteomic, imaging, and clinical data, is essential for gaining a comprehensive understanding of biological systems and disease mechanisms. However, data integration poses significant challenges due to differences in data formats, standards, and quality [203]. Developing robust data integration techniques to harmonize and analyze multi-modal data is critical for advancing precision medicine. This involves creating standardized data formats and protocols that facilitate the seamless exchange and integration of data across different platforms and institutions. Interoperability standards, such as Fast Healthcare Interoperability Resources (FHIR) and Health Level Seven (HL7), play a crucial role in enabling data integration and interoperability in healthcare systems [204].
Additionally, ensuring the interoperability of data across different platforms and institutions is essential for collaborative research and data sharing. This requires the development of data governance frameworks that establish clear guidelines for data access, sharing, and usage while maintaining data privacy and security. Advanced data integration techniques, such as data warehousing, data lakes, and semantic data integration, are being explored to address these challenges and comprehensively analyze multi-modal biomedical data [205].
The ethical implications of computational biomedical engineering are profound and multifaceted. Data privacy and security are paramount concerns, given the sensitive nature of medical and genetic data. Ensuring that patient data is protected and used ethically is essential for maintaining trust in biomedical research and healthcare. Additionally, using machine learning in medical decision-making raises ethical questions about transparency, accountability, and bias [206]. It is crucial to ensure that ML models are transparent, interpretable, and free from biases that could lead to inequitable healthcare outcomes. This involves developing explainable AI (XAI) techniques that provide clear and understandable explanations for model predictions and decisions, allowing clinicians and patients to trust and effectively use these technologies [207].
Ethical considerations also extend to using computational models in clinical trials, where informed consent and the ethical use of data are critical. Researchers must ensure that participants are fully informed about how their data will be used and that their privacy is protected. Additionally, the potential for algorithmic bias in ML models must be addressed to ensure that these technologies do not exacerbate existing healthcare disparities or create new ones. The ethical use of computational models also involves considerations around data ownership and control [208]. Patients and research participants should have control over their data, including the ability to consent to its use and share in the benefits derived from its analysis. This requires the development of ethical frameworks and policies that balance the need for data access and sharing with the protection of individual rights and privacy. Data privacy and security are critical ethical considerations in computational biomedical engineering. The collection, storage, and analysis of large-scale biomedical data raise concerns about the potential for data breaches and misuse. Ensuring that data is anonymized, encrypted, and stored securely is essential for protecting patient privacy [209].
Additionally, clear policies and regulations governing data access and use are necessary to prevent unauthorized use and ensure that data is used ethically. Implementing robust cybersecurity measures and compliance with data protection regulations, such as the General Data Protection Regulation (GDPR) in the European Union and the Health Insurance Portability and Accountability Act (HIPAA) in the United States, is crucial for maintaining data privacy and security. Advanced encryption techniques, secure multi-party computation, and blockchain technology are being explored to enhance data privacy and security in biomedical research [210]. These technologies can provide decentralized and tamper-proof systems for storing and sharing biomedical data, ensuring that data integrity is maintained and that access is restricted to authorized users. Ensuring transparency and accountability in data handling practices is also essential for maintaining trust. This involves implementing audit trails and data provenance mechanisms that track how data is collected, processed, and used, providing a clear record of data transactions and usage [211].
The ethical use of machine learning in medicine is another critical consideration. ML models must be developed and deployed in a manner that is transparent, interpretable, and free from biases. Ensuring that ML algorithms are trained on diverse and representative datasets is essential for preventing bias and ensuring equitable healthcare outcomes [212]. Additionally, using ML in medical decision-making should involve human oversight to ensure that decisions are ethical and clinically sound. This includes implementing clinical decision support systems (CDSS) that provide recommendations based on ML models but allow clinicians to make the final decision. Such systems should provide clear explanations for their recommendations, enabling clinicians to understand and trust the underlying logic [213].
Ethical guidelines and frameworks for developing and using ML in medicine are essential for ensuring that these technologies are used responsibly and ethically. This includes establishing ethical review boards and developing standards for the ethical evaluation of ML models. These guidelines should address issues such as algorithmic transparency, accountability, fairness, and the potential for unintended consequences. Moreover, ongoing monitoring and evaluation of ML models are necessary to identify and mitigate potential biases and ensure they continue to perform accurately and fairly over time. This involves implementing feedback mechanisms that allow for continuous learning and improvement of ML models based on real-world data and outcomes [214].
Current models for estimating data breach costs suffer from significant limitations, including inconsistencies in cost assessments and inadequate consideration of various breach scenarios. Algarni et al. addressed these issues by developing a new, more robust model that provides a comprehensive framework for accurately estimating costs across different types of data breaches [215]. Yazijy et al. expressed that the current data protection measures are insufficient for handling large volumes of biomedical data, focusing more on compliance than proactive privacy design. This slows biomedical research and leaves data vulnerable to cyberattacks [216]. Perakslis et al. concluded that the simplest of strategies could have prevented most of the largest data breaches. They have further expressed that the researcher must clearly understand his or her responsibilities and liability [217]. Azencott expressed that genomic data is sensitive and prone to re-identification using auxiliary information despite its importance for scientific progress. Formal models like k-anonymity, l-diversity, and differential privacy can enhance genomic data privacy but face challenges in balancing utility and computational efficiency [218]. AI and machine learning have made significant progress in healthcare, with applications ranging from virtual assistants to automated diagnoses. However, these advances bring new challenges related to the security and privacy of sensitive biomedical data, particularly with issues like overfitting, data leakage, and adversarial attacks. To address these, methods such as differential privacy and federated learning are being explored alongside privacy-preserving techniques like private record linkage. Ensuring the security and reliability of AI systems in healthcare requires ongoing testing in real-world clinical settings and multidisciplinary efforts to balance performance with privacy [219].
The future of computational biomedical engineering is marked by emerging trends and technologies that hold the potential to revolutionize healthcare. One of the most promising trends is the integration of artificial intelligence (AI) with multi-omics data, such as genomics, proteomics, and metabolomics. AI-driven approaches can analyze these complex datasets to uncover new insights into disease mechanisms and treatment responses, paving the way for more precise and personalized medical interventions [220]. Quantum computing represents another groundbreaking technology poised to transform computational biomedical engineering. With its unparalleled processing power, quantum computing can solve complex biological problems beyond classical computers’ reach. This includes simulating molecular interactions with high accuracy, optimizing drug discovery processes, and improving the modeling of biological systems at an unprecedented scale [221].
Additionally, the rise of personalized medicine, driven by precise genomic and phenotypic data, is set to transform healthcare by enabling tailored treatments and interventions. Advances in gene editing technologies, such as CRISPR-Cas9, combined with computational models, are expected to enable the development of novel therapies that can correct genetic defects at their source [222]. Furthermore, the application of AI in analyzing patient data from wearable devices and electronic health records (EHRs) will facilitate continuous health monitoring and early disease detection, significantly enhancing preventive care. Blockchain technology is also gaining traction in healthcare for secure data sharing and ensuring the integrity of medical records. Blockchain can provide a decentralized and tamper-proof system for storing and sharing patient data, ensuring privacy and security while facilitating collaboration among researchers and clinicians [223].
Future research in computational biomedical engineering should address key challenges and advance the state-of-the-art. Developing more robust and scalable computational models is essential for handling biomedical data’s increasing volume and complexity. This includes improving algorithms for data integration, model training, and validation to ensure the accuracy and reliability of computational predictions [224]. Enhancing data integration techniques to harmonize multi-modal data is critical for advancing precision medicine. Research should prioritize the development of standardized data formats, protocols, and interoperability frameworks that facilitate seamless data exchange across different platforms and institutions. This will enable researchers to build comprehensive models integrating genomic, proteomic, clinical, and environmental data, providing a holistic view of health and disease [225]. Additionally, research should focus on developing interpretable and transparent machine learning models to ensure ethical and equitable healthcare outcomes. Explainable AI (XAI) techniques that provide clear and understandable explanations for model predictions are essential for gaining the trust of clinicians and patients. Research efforts should also address the ethical implications of AI in healthcare, including issues related to bias, fairness, and accountability [226]. Collaborative efforts between computational scientists, biomedical researchers, and clinicians are essential for translating computational innovations into clinical practice. Interdisciplinary research initiatives and partnerships should be encouraged to foster the exchange of knowledge and expertise, driving innovation and improving health outcomes.
The development of robust computational models is a critical research priority. To handle large and complex datasets, these models must be accurate, reliable, and scalable. Advances in machine learning, data mining, and high-performance computing are essential for building models that can process and analyze multi-modal data efficiently [227]. Robust computational models should be validated using rigorous methods to ensure their accuracy and generalizability across different populations and clinical settings. This involves using cross-validation techniques, independent test datasets, and real-world clinical data to evaluate model performance. Continuous monitoring and updating models based on new data and outcomes are necessary to maintain their relevance and effectiveness [228]. User-friendly tools and platforms that enable researchers and clinicians to leverage computational models without requiring extensive technical expertise are crucial for broader adoption. These tools should provide intuitive interfaces for data input, model training, and result interpretation, making it easier for non-experts to use computational models in their work. Furthermore, developing modular and interoperable models that can be easily integrated with existing healthcare systems and workflows is essential for their practical application [229]. This includes the use of open standards and APIs (Application Programming Interfaces) that facilitate data exchange and model integration with electronic health records (EHRs) and other clinical systems [230]. Enhancements in data integration techniques are essential for advancing precision medicine. Integrating diverse data types, such as genomic, proteomic, imaging, and clinical data, provides a holistic view of biological systems and disease mechanisms. Developing robust algorithms and platforms for data harmonization and analysis is critical for overcoming the challenges of data heterogeneity and complexity [231].
Advanced data integration techniques, such as data warehousing, data lakes, and semantic data integration, are being explored to analyze multi-modal biomedical data comprehensively. These techniques involve aggregating data from multiple sources into a centralized repository, where it can be harmonized and analyzed using advanced computational methods [232]. Interoperability standards, such as Fast Healthcare Interoperability Resources (FHIR) and Health Level Seven (HL7), play a crucial role in enabling data integration and interoperability in healthcare systems. Research efforts should focus on developing and adopting these standards to facilitate seamless data exchange and integration across different platforms and institutions [233]. Data integration also involves the development of advanced analytical tools that can process and analyze large volumes of integrated data in real-time. This includes using cloud computing and distributed computing technologies that provide the computational power to handle big data analytics. Additionally, developing visualization tools that can present integrated data in an accessible and interpretable manner is essential for enabling researchers and clinicians to derive actionable insights from complex datasets [234].
Policy and regulation play a crucial role in shaping the future of computational biomedical engineering. Clear policies and regulations governing data privacy, security, and ethical use are essential for maintaining trust in biomedical research and healthcare. Ensuring compliance with data protection regulations, such as the General Data Protection Regulation (GDPR) in the European Union and the Health Insurance Portability and Accountability Act (HIPAA) in the United States, is crucial for protecting patient privacy [235]. Ethical guidelines and frameworks for developing and using machine learning in medicine are essential for ensuring that these technologies are used responsibly and ethically. This includes establishing ethical review boards and the development of standards for the ethical evaluation of machine learning models. These guidelines should address algorithmic transparency, accountability, fairness, and the potential for unintended consequences [236]. Policies should also encourage data sharing and collaboration while ensuring data privacy and security. This involves developing data governance frameworks that establish clear data access, sharing, and usage guidelines while maintaining data privacy and security. Incentives for data sharing, such as funding for collaborative research initiatives and the development of data-sharing platforms, can facilitate the exchange of knowledge and expertise, driving innovation and improving health outcomes [237]. Additionally, policy and regulation should support developing and adopting interoperability standards and frameworks that enable seamless data exchange and integration across different platforms and institutions. This includes funding for developing and implementing these standards, as well as incentives for their adoption by healthcare providers and research institutions [238]. Collaborative efforts between policymakers, researchers, and clinicians are necessary to develop policies and regulations supporting innovation while safeguarding ethical and legal standards. This includes engaging stakeholders from various sectors in the policy-making process to ensure that diverse perspectives inform policies and address the needs and concerns of all stakeholders [239].
Benchmarking studies are essential for comparing the performance of computational methods but must be carefully designed to ensure accurate and unbiased results. Weber et al. highlighted the potential pitfalls, such as bias in dataset selection and evaluation metrics, which can mislead users and developers, and stressed the need for a thorough discussion of limitations. Well-designed benchmarks provide valuable insights for method users and developers, aiding in the informed selection of methods and guiding future research efforts [240]. Massey et al. concluded that the absence of verification, validation, and performance benchmarks hinders progress in computational electromagnetics. The authors also proposed a benchmark suite with application-specific problems, reference solutions, and performance measures, emphasizing the challenge of comparing computational costs across methods [241]. Mangul et al. reported that despite challenges like tool dependencies, limited documentation, and the high volume of new methods, systematic and standardized benchmarking practices are essential for ensuring reproducibility and transparency in computational biology [242]. Ellrott et al. found that cloud-based virtualization and computing methods can enhance the reproducibility and reusability of biomedical analysis tools. The authors have also reviewed recent data challenges, highlighting key lessons for improving biomedical data analysis through crowd-sourced benchmarking [243]. Peters et al. highlighted that benchmarks are essential for evaluating computational biology methods, but developing good benchmarks is challenging. Many tool developers generate biased benchmarks, which can lead to reporting bias [244].
Computational engineering in biomedicine raises critical privacy and security concerns, necessitating robust data protection measures for handling sensitive patient information. These privacy-preserving technologies and methodologies are essential for advancing computational engineering in biomedical science while safeguarding sensitive patient data [245]. Federated learning emerges as a crucial solution in ICT-enabled biomedical research, allowing collaborative learning without sharing raw patient data. Federated learning, in particular, addresses the challenge of data collection in ICT environments, enabling service providers to leverage valuable insights without directly accessing raw patient information [246]. Advanced encryption techniques safeguard data during transmission and storage in biomedical applications, ensuring confidentiality of sensitive medical information. Blockchain technology offers secure, transparent data management in computational biomedicine, providing an immutable ledger for maintaining data integrity and traceability [247]. Homomorphic encryption permits computations on encrypted biomedical data securely, enabling analysis of sensitive information while maintaining data confidentiality. Encryption techniques, including homomorphic encryption, allow for secure data analysis and collaboration among researchers and healthcare providers [248]. Edge computing reduces data transmission risks in biomedical applications by processing data locally, minimizing exposure to potential security breaches. Blockchain technology enhances data traceability and integrity, which is crucial for maintaining trust in biomedical research and applications [249].
Robust authentication and access control mechanisms form the foundation of data security in biomedical informatics. Robust authentication and access control systems protect biomedical data integrity, ensuring only authorized personnel can access sensitive information [250]. Ethical frameworks guide the responsible use of computational tools in biomedicine, balancing innovation with patient privacy and data security concerns. Ethical considerations play a vital role in guiding the development and implementation of these technologies, ensuring that innovation in computational engineering aligns with patient rights and data protection regulations [251]. Differential privacy and secure multi-party computation facilitate large-scale data analysis while protecting individual privacy. Differential privacy algorithms protect individual patient data in large-scale analyses by adding controlled noise to data, preserving privacy in biomedical research [252]. Edge computing brings data processing closer to the source, reducing the risk of data breaches during transmission. Secure multi-party computation enables collaborative analysis without data exposure, allowing multiple parties to jointly compute without revealing inputs [253]. By integrating these privacy and security measures, the convergence of computational engineering and biomedical science can foster innovation while maintaining the highest data protection standards and patient confidentiality [254].
The convergence of computational engineering and biomedical science has led to transformative healthcare and medical research advancements. This review explored several critical areas where this intersection has yielded significant benefits, highlighting the role of computational models, bioinformatics, machine learning, and wearable technology in driving innovation.
1. Computational models have become invaluable tools in biomedical science, enabling the simulation of complex biological systems. These models provide insights into cellular and molecular processes and organ-level functions, which are difficult or costly to study using traditional experimental methods. They facilitate personalized medicine by predicting patient-specific treatment responses, which helps optimize therapeutic strategies. Despite their potential, computational models face challenges related to data quality, computational power, and model validation. Future research should focus on building more scalable, reliable models that integrate diverse data for improved clinical applications.
2. Bioinformatics has transformed the analysis of biological data, especially with the advent of high-throughput technologies like genomics and proteomics. It has enabled the identification of disease-associated genetic variants, supporting the development of personalized medicine and targeted therapies. Structural bioinformatics and sequence analysis have furthered our understanding of protein interactions, enhancing drug discovery and optimization. As bioinformatics evolves, integrating multi-omics data and improving data management techniques will be crucial for advancing precision medicine and unlocking new therapeutic possibilities.
3. Machine learning (ML) has revolutionized medical diagnostics by automating the analysis of complex medical data. ML algorithms excel in identifying patterns in imaging data, genetic information, and patient records, enabling earlier and more accurate diagnosis of diseases such as cancer and cardiovascular disorders. The ability to predict disease progression and patient outcomes has improved personalized treatment planning. However, ethical considerations around transparency and bias must be addressed to ensure ML models deliver equitable healthcare outcomes. Future advancements in explainable AI will be essential to ensure trust and clinical adoption.
4. Wearable technology has integrated seamlessly into healthcare, allowing for real-time monitoring of vital signs such as heart rate, glucose levels, and physical activity. These devices have proven especially valuable in managing chronic diseases like diabetes and cardiovascular conditions, where continuous monitoring is crucial for early intervention. Wearable devices reduce hospital visits, enhance preventive care, and empower patients to manage their health. Moving forward, integrating wearable data with AI and healthcare systems will enhance their predictive capabilities and broaden their use in personalized healthcare.
5. The convergence of computational engineering and biomedical science has led to numerous breakthroughs in personalized healthcare. Computational models and machine learning algorithms have enabled the design of personalized therapies, especially in fields like oncology, where treatments are tailored to a patient’s genetic profile. Additionally, these technologies have been instrumental in responding to public health challenges, such as during the COVID-19 pandemic, where epidemiological models informed strategies for disease control. Continued interdisciplinary collaboration between computational scientists and biomedical researchers will be key to future innovations.
6. Despite the successes, several challenges persist at the intersection of computational engineering and biomedical science. Data quality, privacy, and security are paramount concerns, particularly with the growing volume of sensitive biomedical data. Additionally, ensuring that computational models and machine learning algorithms are scalable, reliable, and bias-free remains a significant hurdle. Ethical considerations around data use, patient privacy, and transparency in AI-driven healthcare must be prioritized. Policymakers and researchers must work together to establish clear regulations and guidelines that foster innovation while safeguarding ethical standards.
7. Policy and regulation will play a crucial role in shaping the future of this field. Clear data privacy, security, and ethical guidelines are essential for maintaining trust in biomedical research and healthcare. Collaborative efforts between policymakers, researchers, and clinicians will ensure that policies support innovation while safeguarding ethical standards.
8. Emerging technologies such as artificial intelligence, quantum computing, and blockchain hold the potential to revolutionize healthcare further. AI’s integration with multi-omics data will enable more precise diagnostics and treatments, while quantum computing promises to solve complex biological problems that exceed the capabilities of classical computing. Blockchain technology could secure data sharing and collaboration in biomedical research by ensuring transparency and data integrity. Future research should prioritize developing more robust computational models, integrating diverse data sources, and ensuring the ethical application of these advanced technologies in healthcare.
By fostering a culture of interdisciplinary collaboration, the full potential of computational biomedical engineering can be harnessed to improve patient outcomes, enhance healthcare delivery, and deepen our understanding of complex biological systems. The future of healthcare lies at the intersection of computational and biomedical sciences, where innovation and collaboration will continue to drive progress and revolutionize the field.
Acknowledgement: None.
Funding Statement: The authors received no specific funding for this study.
Author Contributions: Study conception and design: Hariharasakthisudhan Ponnarengan; Literature review and data collection: Sivakumar Rajendran, Vikas Khalkar, Gunapriya Devarajan, K. Logesh; Analysis and interpretation of literature: Hariharasakthisudhan Ponnarengan, Sivakumar Rajendran, Vikas Khalkar; Visualization and graphical representation: Vikas Khalkar, Gunapriya Devarajan; Draft manuscript preparation: Sivakumar Rajendran, Vikas Khalkar, Gunapriya Devarajan, Logesh Kamaraj; Critical revision of the manuscript: Hariharasakthisudhan Ponnarengan, Logesh Kamaraj. All authors reviewed the results and approved the final version of the manuscript.
Availability of Data and Materials: Not specific data related to this article as it is a review article.
Ethics Approval: No applicable.
Conflicts of Interest: The authors declare no conflicts of interest to report regarding the present study.
References
1. Hasan A, Saliba J, Pezeshgi Modarres H, Bakhaty A, Nasajpour A, Mofrad MR, et al. Micro and nanotechnologies in heart valve tissue engineering. Biomaterials. 2016;103:278–92. doi:10.1016/j.biomaterials.2016.07.001. [Google Scholar] [PubMed] [CrossRef]
2. Boon M. An engineering paradigm in the biomedical sciences: knowledge as epistemic tool. Prog Biophys Mol Biol. 2017;129:25–39. doi:10.1016/j.pbiomolbio.2017.04.001. [Google Scholar] [PubMed] [CrossRef]
3. Brodland GW. How computational models can help unlock biological systems. Semin Cell Dev Biol. 2015;47–48:62–73. doi:10.1016/j.semcdb.2015.07.001. [Google Scholar] [PubMed] [CrossRef]
4. Seo S, Kim H, Sung JH, Choi N, Lee K, Kim HN. Microphysiological systems for recapitulating physiology and function of blood-brain barrier. Biomaterials. 2020;232:119732. doi:10.1016/j.biomaterials.2019.119732. [Google Scholar] [PubMed] [CrossRef]
5. Miller KS, Khosravi R, Breuer CK, Humphrey JD. A hypothesis-driven parametric study of effects of polymeric scaffold properties on tissue engineered neovessel formation. Acta Biomater. 2014;11:283–94. doi:10.1016/j.actbio.2014.09.046. [Google Scholar] [PubMed] [CrossRef]
6. Stanković T, Ranđelović T, Dragoj M, Stojković Burić S, Fernández L, Ochoa I, et al. In vitro biomimetic models for glioblastoma–a promising tool for drug response studies. Drug Resist Updat. 2021;55:100753. doi:10.1016/j.drup.2021.100753. [Google Scholar] [PubMed] [CrossRef]
7. Jiang H, Li X, Chen T, Liu Y, Wang Q, Wang Z, et al. Bioprinted vascular tissue: assessing functions from cellular, tissue to organ levels. Mater Today Bio. 2023;23:100846. doi:10.1016/j.mtbio.2023.100846. [Google Scholar] [PubMed] [CrossRef]
8. Dankano A, Prather R, Lozinski B, Divo E, Kassab A, DeCampli W. Tailoring left ventricular assist device cannula implantation using coupled multi-scale multi-objective optimization. Med Eng Phys. 2024;125:104124. doi:10.1016/j.medengphy.2024.104124. [Google Scholar] [PubMed] [CrossRef]
9. Afazal M, Afreen S, Chanda A. Computational modelling and analysis of hard tissue behavior around 0.5 mm and 0.85 mm platform switched abutment using 3D finite element analysis. Forces Mech. 2023;13:100243. doi:10.1016/j.finmec.2023.100243. [Google Scholar] [CrossRef]
10. Fillingham P, Romero Bhathal J, Marsh LM, Barbour MC, Kurt M, Ionita CN, et al. Improving the accuracy of computational fluid dynamics simulations of coiled cerebral aneurysms using finite element modeling. J Biomech. 2023;157:111733. doi:10.1016/j.jbiomech.2023.111733. [Google Scholar] [PubMed] [CrossRef]
11. Shim V, Tayebi M, Kwon E, Guild SJ, Scadeng M, Dubowitz D, et al. Combining advanced magnetic resonance imaging (MRI) with finite element (FE) analysis for characterising subject-specific injury patterns in the brain after traumatic brain injury. Eng Comput. 2022;38:3925–37. doi:10.1007/s00366-022-01697-4. [Google Scholar] [CrossRef]
12. Altman MB, Wan W, Hosseini AS, Arabi Nowdeh S, Alizadeh M. Machine learning algorithms for FPGA implementation in biomedical engineering applications: a review. Heliyon. 2024;10(4):e26652. doi:10.1016/j.heliyon.2024.e26652. [Google Scholar] [PubMed] [CrossRef]
13. Kundu P, Beura S, Mondal S, Das AK, Ghosh A. Machine learning for the advancement of genome-scale metabolic modeling. Biotechnol Adv. 2024;74:108400. doi:10.1016/j.biotechadv.2024.108400. [Google Scholar] [PubMed] [CrossRef]
14. Li J, Xu J, Chen Z, Lu Y, Hua X, Jin Z. Computational modelling of articular joints with biphasic cartilage: recent advances, challenges and opportunities. Med Eng Phys. 2024;126:104130. doi:10.1016/j.medengphy.2024.104130. [Google Scholar] [PubMed] [CrossRef]
15. Lawlor A, Lin C, Gómez Rivas J, Ibáñez L, Abad López P, Willemse P, et al. Predictive models for assessing patients’ response to treatment in metastatic prostate cancer: a systematic review. Eur Urol Open Sci. 2024;63:126–35. doi:10.1016/j.euros.2024.03.012. [Google Scholar] [PubMed] [CrossRef]
16. El Barghouti M, Houari F, Akjouj A, Mir A. Engineering and optimization of the SPR device ZnO/Ag/WO3/Ni/2D-nanomaterials highly sensitive for biomedical processing and detection. Opt Mater. 2024;149:115019. doi:10.1016/j.optmat.2024.115019. [Google Scholar] [CrossRef]
17. Vanderlaan RD, Di Nardo A, Amon CH. A multidisciplinary approach to patient-specific surgical planning in congenital heart disease. JACC Adv. 2024;3(7):101058. doi:10.1016/j.jacadv.2024.101058. [Google Scholar] [PubMed] [CrossRef]
18. Zhang Y, Cheng L, Chen G, Alghazzawi D. Evolutionary computation in bioinformatics: a survey. Neurocomputing. 2024;591:127758. doi:10.1016/j.neucom.2024.127758. [Google Scholar] [CrossRef]
19. Uesaka K, Oka H, Kato R, Kanie K, Kojima T, Tsugawa H, et al. Bioinformatics in bioscience and bioengineering: recent advances, applications, and perspectives. J Biosci Bioeng. 2022;134(5):363–73. doi:10.1016/j.jbiosc.2022.08.004. [Google Scholar] [PubMed] [CrossRef]
20. Nakamae K, Bono H. Genome editing and bioinformatics. Gene Genome Ed. 2022;3–4:100018. doi:10.1016/j.ggedit.2022.100018. [Google Scholar] [CrossRef]
21. Swaminathan S, Sundaramurthi JC, Palaniappan AN, Narayanan S. Recent developments in genomics, bioinformatics and drug discovery to combat emerging drug-resistant tuberculosis. Tuberculosis. 2016;101:31–40. doi:10.1016/j.tube.2016.08.002. [Google Scholar] [PubMed] [CrossRef]
22. Kumar Chandra D, Kumar A, Mahapatra C. Machine learning-enforced bioinformatics approaches for drug discovery and development. In: Deep learning applications in translational bioinformatics. Academic Press; 2023. p. 141–75. doi:10.1016/B978-0-443-22299-3.00010-4. [Google Scholar] [CrossRef]
23. Taber DJ, Ward RC, Buchanan CH, Axon RN, Milfred-LaForest S, Rife K, et al. Results of a multicenter cluster-randomized controlled clinical trial testing the effectiveness of a bioinformatics-enabled pharmacist intervention in transplant recipients. Am J Transplant. 2023;23(12):1939–48. doi:10.1016/j.ajt.2023.08.004. [Google Scholar] [PubMed] [CrossRef]
24. García-Moreno PJ, Yesiltas B, Gregersen Echers S, Marcatili P, Overgaard MT, Hansen EB, et al. Recent advances in the production of emulsifying peptides with the aid of proteomics and bioinformatics. Curr Opin Food Sci. 2023;51:101039. doi:10.1016/j.cofs.2023.101039. [Google Scholar] [CrossRef]
25. Garza-Ulloa J. Machine learning models applied to biomedical engineering. In: Applied biomedical engineering using artificial intelligence and cognitive models. Academic Press; 2021. p. 175–334. doi:10.1016/B978-0-12-820718-5.00002-7. [Google Scholar] [CrossRef]
26. Shaikhina T, Lowe D, Daga S, Briggs D, Higgins R, Khovanova N. Machine learning for predictive modelling based on small data in biomedical engineering. IFAC-PapersOnLine. 2014;48(20):469–74. doi:10.1016/j.ifacol.2015.10.185. [Google Scholar] [CrossRef]
27. Rai HM, Yoo J, Atif Moqurrab S, Dashkevych S. Advancements in traditional machine learning techniques for detection and diagnosis of fatal cancer types: comprehensive review of biomedical imaging datasets. Measurement. 2024;225:114059. doi:10.1016/j.measurement.2023.114059. [Google Scholar] [CrossRef]
28. Garg G, Kaur S. Introduction to the special section on biomedical data and image analysis using advanced machine learning methods. Comput Electr Eng. 2024;114:109087. doi:10.1016/j.compeleceng.2024.109087. [Google Scholar] [CrossRef]
29. Guo K, Wu M, Soo Z, Yang Y, Zhang Y, Zhang Q, et al. Artificial intelligence-driven biomedical genomics. Knowl-Based Syst. 2023;279:110937. doi:10.1016/j.knosys.2023.110937. [Google Scholar] [CrossRef]
30. Özdemir V. Genomics, the Internet of Things, artificial intelligence, and society. In: Applied genomics and public health. Academic Press; 2020. p. 275–85. [Google Scholar]
31. Guan S, Wang G. Drug discovery and development in the era of artificial intelligence: from machine learning to large language models. Artif Intell Chem. 2024;2(1):100070. doi:10.1016/j.aichem.2024.100070. [Google Scholar] [CrossRef]
32. Parvatikar PP, Patil S, Khaparkhuntikar K, Patil S, Singh PK, Sahana R, et al. Artificial intelligence: machine learning approach for screening large database and drug discovery. Antiviral Res. 2023;220:105740. doi:10.1016/j.antiviral.2023.105740. [Google Scholar] [PubMed] [CrossRef]
33. Sridhar A, Mawia A, Amutha AL. Mobile application development for disease diagnosis based on symptoms using machine learning techniques. Procedia Comput Sci. 2022;218:2594–603. doi:10.1016/j.procs.2023.01.233. [Google Scholar] [CrossRef]
34. Bakri MH, Özarslan AC, Erarslan A, Basaran Elalmis Y, Ciftci F. Biomedical applications of wearable biosensors. Next Mater. 2024;3:100084. doi:10.1016/j.nxmate.2023.100084. [Google Scholar] [CrossRef]
35. Mahanty B, Kumar Ghosh S, Lee D. Advancements in polymer nanofiber-based piezoelectric nanogenerators: revolutionizing self-powered wearable electronics and biomedical applications. Chem Eng J. 2024;495:153481. doi:10.1016/j.cej.2024.153481. [Google Scholar] [CrossRef]
36. Lee HK, Yang YJ, Koirala GR, Oh S, Kim T. From lab to wearables: innovations in multifunctional hydrogel chemistry for next-generation bioelectronic devices. Biomaterials. 2024;310:122632. doi:10.1016/j.biomaterials.2024.122632. [Google Scholar] [PubMed] [CrossRef]
37. Zhao F, Balthazaar SJ, Hiremath SV, Nightingale TE, Panza GS. Enhancing SCI care: using wearable technologies for physical activity, sleep, and cardiovascular health. Arch Phys Med Rehabil. 2024;105:1997–2007. doi:10.1016/j.apmr.2024.06.014. [Google Scholar] [PubMed] [CrossRef]
38. Raue A, Schilling M, Bachmann J, Matteson A, Schelker M, Kaschek D, et al. Correction: lessons learned from quantitative dynamical modeling in systems biology. PLoS One. 2013;8(12). doi:10.1371/annotation/ea0193d8-1f7f-492a-b0b7-d877629fdcee. [Google Scholar] [CrossRef]
39. White KA, McEntire KD, Buan NR, Robinson L, Barbar E. Charting a new frontier integrating mathematical modeling in complex biological systems from molecules to ecosystems. Integr Comp Biol. 2022;61(6):2255–66. doi:10.1093/icb/icab165. [Google Scholar] [PubMed] [CrossRef]
40. Villaverde AF, Bongard S, Mauch K, Müller D, Balsa-Canto E, Schmid J, et al. A consensus approach for estimating the predictive accuracy of dynamic models in biology. Comput Methods Programs Biomed. 2015;119(1):17–28. doi:10.1016/j.cmpb.2015.02.001. [Google Scholar] [PubMed] [CrossRef]
41. Tiwary BK. Computational medicine: quantitative modeling of complex diseases. Brief Bioinform. 2020;21(2):429–40. doi:10.1093/bib/bbz005. [Google Scholar] [PubMed] [CrossRef]
42. Walpole J, Papin JA, Peirce SM. Multiscale computational models of complex biological systems. Annu Rev Biomed Eng. 2013;15:137–54. doi:10.1146/annurev-bioeng-071811-150104. [Google Scholar] [PubMed] [CrossRef]
43. Bouteiller JMC, Feng Z, Onopa A, Huang M, Hu EY, Somogyi E, et al. Maximizing predictability of a bottom-up complex multi-scale model through systematic validation and multi-objective multi-level optimization. In: 2015 7th International IEEE/EMBS Conference on Neural Engineering (NER2015 Apr 22; IEEE; p. 300–3. doi:10.1109/NER.2015.7146619. [Google Scholar] [CrossRef]
44. Jacop MM, Manigrass M, De Vivo M. Finding the ion in the RNA-stack: can computational models accurately predict key functional elements in large macromolecular complexes? J Chem Inf Model. 2021;61(6):2511. doi:10.1021/acs.jcim.1c00572. [Google Scholar] [PubMed] [CrossRef]
45. Iris F, Gea M, Lampe PH, Santamaria P. Production and implementation of predictive biological models. Med Sci. 2009;25(6–7):608–16. doi:10.1051/medsci/2009256-7608.French. [Google Scholar] [CrossRef]
46. Paton RC. Some computational models at the cellular level. Biosystems. 1992;29(2–3):63–75. doi:10.1016/0303-2647(93)90084-P. [Google Scholar] [PubMed] [CrossRef]
47. Svyetlichnyy D. Frontal cellular automata for modelling microstructure evolution: computational complexity analysis. Comput Mater Sci. 2023;230:112478. doi:10.1016/j.commatsci.2023.112478. [Google Scholar] [CrossRef]
48. Peirce-Cottler SM, Marsden A. Multiscale computational modeling of biomedical systems: current approaches and payoffs. Curr Opin Biomed Eng. 2019;11:A1–3. doi:10.1016/j.cobme.2019.12.001. [Google Scholar] [CrossRef]
49. Guo X, Gong C, Zhai Y, Yu H, Li J, Sun H, et al. Biomechanical characterization of normal and pathological human ascending aortic tissues via biaxial testing experiment, constitutive modeling and finite element analysis. Comput Biol Med. 2023;166:107561. doi:10.1016/j.compbiomed.2023.107561.MRI. [Google Scholar] [CrossRef]
50. Oshin TA, Abhulimen KE. Simulation of flow in an artery under pathological hemodynamic conditions: the use of a diagnostic disease descriptor. Heliyon. 2022;8(7):e09992. doi:10.1016/j.heliyon.2022.e09992. [Google Scholar] [PubMed] [CrossRef]
51. Matin HN, Setayeshi S. A computational tumor growth model experience based on molecular dynamics point of view using deep cellular automata. Artif Intell Med. 2024;148:102752. doi:10.1016/j.artmed.2023.102752. [Google Scholar] [PubMed] [CrossRef]
52. Hollingsworth SA, Dror RO. Molecular dynamics simulation for all. Neuron. 2018;99(6):1129–43. doi:10.1016/j.neuron.2018.08.011. [Google Scholar] [PubMed] [CrossRef]
53. Fischer MM, Blüthgen N. On tumoural growth and treatment under cellular dedifferentiation. J Theor Biol. 2023;557:111327. doi:10.1016/j.jtbi.2022.111327. [Google Scholar] [PubMed] [CrossRef]
54. Kfoury YS, Ji F, Jain E, Mazzola M, Schiroli G, Papazian A, et al. The bone marrow stroma in human myelodysplastic syndrome reveals alterations that regulate disease progression. Blood Adv. 2023;7(21):6608–23. doi:10.1182/bloodadvances.2022008268. [Google Scholar] [PubMed] [CrossRef]
55. Ignacio M, Slater G. A Lattice Kinetic Monte Carlo method to study drug release from swelling porous delivery systems. Physica A. 2022;603:127775. doi:10.1016/j.physa.2022.127775. [Google Scholar] [CrossRef]
56. Huang Y, Mao Z, Zhang Y, Zhao J, Luan X, Wu K, et al. Omics data analysis reveals the system-level constraint on cellular amino acid composition. Synth Syst Biotechnol. 2024;9(2):304–11. doi:10.1016/j.synbio.2024.03.001. [Google Scholar] [PubMed] [CrossRef]
57. Fedele M, Piersanti R, Regazzoni F, Salvador M, Africa PC, Bucelli M, et al. A comprehensive and biophysically detailed computational model of the whole human heart electromechanics. Comput Methods Appl Mech Eng. 2023;410:7825. doi:10.1016/j.cma.2023.115983. [Google Scholar] [CrossRef]
58. Zappon E, Salvador M, Piersanti R, Regazzoni F, Dede’ L, Quarteroni A. An integrated heart-torso electromechanical model for the simulation of electrophysiological outputs accounting for myocardial deformation. Comput Methods Appl Mech Eng. 2024;427:117077. doi:10.1016/j.cma.2024.117077. [Google Scholar] [CrossRef]
59. Mora MT, Zaza A, Trenor B. Insights from an electro-mechanical heart failure cell model: role of SERCA enhancement on arrhythmogenesis and myocyte contraction. Comput Methods Programs Biomed. 2023;230:107350. doi:10.1016/j.cmpb.2023.107350. [Google Scholar] [PubMed] [CrossRef]
60. Djukic T, Topalovic M, Filipovic N. Validation of lattice Boltzmann based software for blood flow simulations in complex patient-specific arteries against traditional CFD methods. Math Comput Simul. 2022;203:957–76. doi:10.1016/j.matcom.2022.07.027. [Google Scholar] [CrossRef]
61. Aramburu J, Antón R, Rodríguez-Fraile M, Sangro B, Bilbao JI. Computational fluid dynamics modeling of liver radioembolization: a review. Cardiovasc Intervent Radiol. 2022 Jan;45(1):12–20. doi:10.1007/s00270-021-02956-5. [Google Scholar] [PubMed] [CrossRef]
62. Aycan O, Topuz A, Kadem L. Evaluating uncertainties in CFD simulations of patient-specific aorta models using grid convergence index method. Mech Res Commun. 2023;133:104188. doi:10.1016/j.mechrescom.2023.104188. [Google Scholar] [CrossRef]
63. Li Z, Tang S, Shi Z, Li B, Feng D, Xie D, et al. Multi-scale cellular PLA-based bionic scaffold to promote bone regrowth and repair. Int J Biol Macromol. 2023;245:125511. doi:10.1016/j.ijbiomac.2023.125511. [Google Scholar] [PubMed] [CrossRef]
64. Reilly CB, Ingber DE. Multi-scale modeling reveals use of hierarchical tensegrity principles at the molecular, multi-molecular, and cellular levels. Extreme Mech Lett. 2018;20:21–8. doi:10.1016/j.eml.2018.01.001. [Google Scholar] [CrossRef]
65. Sabbar Y. Based on epidemiological parameter data, probe into a stochastically perturbed dominant variant of the COVID-19 pandemic model. Gene. 2024;926:148608. doi:10.1016/j.gene.2024.148608. [Google Scholar] [PubMed] [CrossRef]
66. Çakmaklı C, Şimşek Y. Bridging the Covid-19 data and the epidemiological model using the time-varying parameter SIRD model. J Econom. 2024;242(1):105787. doi:10.1016/j.jeconom.2024.105787. [Google Scholar] [CrossRef]
67. Luan J, Qiao Y, Mao L, Fan J, Zhu T, Luo K. The role of aorta distal to stent in the occurrence of distal stent graft-induced new entry tear: a computational fluid dynamics and morphological study. Comput Biol Med. 2023;166:107554. doi:10.1016/j.compbiomed.2023.107554. [Google Scholar] [PubMed] [CrossRef]
68. Zhang X, Xiong J, Ma W, Xue L, Xu Z, Zhang H, et al. Evaluation of embedded modular branched stent graft in treating aortic arch aneurysm using imaging-based computational flow analysis. Med Novel Technol Devices. 2024;21:100285. doi:10.1016/j.medntd.2024.100285. [Google Scholar] [CrossRef]
69. Conti M, Ferrarini A, Finotello A, Salsano G, Auricchio F, Palombo D, et al. Patient-specific computational fluid dynamics of femoro-popliteal stent-graft thrombosis. Med Eng Phys. 2020;86:57–64. doi:10.1016/j.medengphy.2020.10.011. [Google Scholar] [PubMed] [CrossRef]
70. Bonifácio ED, Araújo CA, Guimarães MV, De Souza MP, Lima TP, De Avelar Freitas BA, et al. Computational model of the cancer necrotic core formation in a tumor-on-a-chip device. J Theor Biol. 2024;592:111893. doi:10.1016/j.jtbi.2024.111893. [Google Scholar] [PubMed] [CrossRef]
71. Lampropoulos I, Kavousanakis M. Application of combination chemotherapy in two dimensional tumor growth model with heterogeneous vasculature. Chem Eng Sci. 2023;280:118965. doi:10.1016/j.ces.2023.118965. [Google Scholar] [CrossRef]
72. Beigi OM, Nóbrega LR, Houghten S, Alves Pereira A, De Oliveira Andrade A. Freezing of gait in Parkinson’s disease: classification using computational intelligence. Biosystems. 2023;232:105006. doi:10.1016/j.biosystems.2023.105006. [Google Scholar] [PubMed] [CrossRef]
73. Zeng Q, Zheng Y, Yi D. Bifurcation analysis of a Parkinson’s disease model with two time delays. Math Comput Simul. 2024;219:1–11. doi:10.1016/j.matcom.2023.12.007. [Google Scholar] [CrossRef]
74. Satam H, Joshi K, Mangrolia U, Waghoo S, Zaidi G, Rawool S, et al. Next-generation sequencing technology: current trends and advancements. Biology. 2023;12(7):997. doi:10.3390/biology12070997. [Google Scholar] [PubMed] [CrossRef]
75. De Vries JJ, Brown JR, Couto N, Beer M, Le Mercier P, Sidorov I, et al. Recommendations for the introduction of metagenomic next-generation sequencing in clinical virology, part II: bioinformatic analysis and reporting. J Clin Virol. 2021;138:104812. doi:10.1016/j.jcv.2021.104812. [Google Scholar] [PubMed] [CrossRef]
76. Jia B, Ojima-Kato T, Kojima T, Nakano H. Rapid and cost-effective epitope mapping using PURE ribosome display coupled with next-generation sequencing and bioinformatics. J Biosci Bioeng. 2024;137(4):321–8. doi:10.1016/j.jbiosc.2024.01.008. [Google Scholar] [PubMed] [CrossRef]
77. Oduselu GO, Ajani OO, Ajamma YU, Brors B, Adebiyi E. Homology modelling and molecular docking studies of selected substituted Benzo[d]imidazol-1-yl)methyl) benzimidamide scaffolds on Plasmodium falciparum adenylosuccinate lyase receptor. Bioinform Biol Insights. 2019;13:1. doi:10.1177/1177932219865533. [Google Scholar] [PubMed] [CrossRef]
78. ElHefnawi M, Hegazy E, Elfiky A, Jeon Y, Jeon S, Bhak J, et al. Complete genome sequence and bioinformatics analysis of nine Egyptian females with clinical information from different geographic regions in Egypt. Gene. 2021;769:145237. doi:10.1016/j.gene.2020.145237. [Google Scholar] [PubMed] [CrossRef]
79. Parmin NA, Hashim U, Gopinath SC. Designing probe from E6 genome region of human Papillomavirus 16 for sensing applications. Int J Biol Macromol. 2018;107:1738–46. doi:10.1016/j.ijbiomac.2017.10.051. [Google Scholar] [PubMed] [CrossRef]
80. Ait Lahcen N, Liman W, Oubahmane M, Hdoufane I, Habibi Y, Alanazi AS, et al. Drug design of new anti-EBOV inhibitors: QSAR, homology modeling, molecular docking and molecular dynamics studies. Arab J Chem. 2024;17(9):105870. doi:10.1016/j.arabjc.2024.105870. [Google Scholar] [CrossRef]
81. Guitton Y, Tremblay-Franco M, Le Corguillé G, Martin J, Pétéra M, Roger-Mele P, et al. Create, run, share, publish, and reference your LC-MS, FIA-MS, GC-MS, and NMR data analysis workflows with the Workflow4Metabolomics 3.0 Galaxy online infrastructure for metabolomics. Int J Biochem Cell Biol. 2017;93:89–101. doi:10.1016/j.biocel.2017.07.002. [Google Scholar] [PubMed] [CrossRef]
82. Cafaro M, Epicoco I, Pulimeno M. Techniques for designing bioinformatics algorithms. In: Encyclopedia of bioinformatics and computational biology. Academic Press; 2018. p. 5–14. doi:10.1016/B978-0-12-809633-8.20316-6. [Google Scholar] [CrossRef]
83. Shoaib M, Singh A, Gulati S, Kukreti S. Mapping genomes by using bioinformatics data and tools. In: Chemoinformatics and bioinformatics in the pharmaceutical sciences. Academic Press; 2020. p. 245–78. doi:10.1016/B978-0-12-821748-1.00002-6. [Google Scholar] [CrossRef]
84. Sánchez-Osuna M, Pedrosa M, Bierge P, Gómez-Sánchez I, Alguacil-Guillén M, Espasa M, et al. Genomic analysis of Staphylococcus aureus isolates from bacteremia reveals genetic features associated with the COVID-19 pandemic. iScience. 2024;27:110402. doi:10.1016/j.isci.2024.110402. [Google Scholar] [PubMed] [CrossRef]
85. Manolis AJ, Poulimenos LE, Ambrosio G, Kallistratos MS, Lopez-Sendon J, Dechend R, et al. Medical treatment of stable angina: a tailored therapeutic approach. Int J Cardiol. 2016;220:445–53. doi:10.1016/j.ijcard.2016.06.150. [Google Scholar] [PubMed] [CrossRef]
86. Larder BA, Revell A, Mican JM, Agan BK, Harris M, Torti C, et al. Clinical evaluation of the potential utility of computational modeling as an HIV treatment selection tool by physicians with considerable HIV experience. AIDS Patient Care STDS. 2011 Jan 1;25(1):29–36. doi:10.1089/apc.2010.0254. [Google Scholar] [PubMed] [CrossRef]
87. Drusbosky LM, Cogle CR. Computational modeling and treatment identification in the myelodysplastic syndromes. Curr Hematol Malig Rep. 2017;12:478–83. doi:10.1007/s11899-017-0412-z. [Google Scholar] [PubMed] [CrossRef]
88. Cook L, Araujo A, Pow-Sang J, Budzevich MM, Basanta D, Lynch CC. Predictive computational modeling to define effective treatment strategies for bone metastatic prostate cancer. Sci Rep. 2016 Jul 14;6(1):29384. doi:10.1038/srep29384. [Google Scholar] [PubMed] [CrossRef]
89. Niederer SA, Lumens J, Trayanova NA. Computational models in cardiology. Nat Rev Cardiol. 2019;16(2):100–11. doi:10.1038/s41569-018-0104-y. [Google Scholar] [PubMed] [CrossRef]
90. Winslow RL, Trayanova N, Geman D, Miller MI. Computational medicine: translating models to clinical care. Sci Transl Med. 2012;4(158):1845. doi:10.1126/scitranslmed.3003528. [Google Scholar] [PubMed] [CrossRef]
91. Ogilvie LA, Wierling CK, Kessler T, Lehrach H, Lange BM. Predictive modeling of drug treatment in the area of personalized medicine. Cancer Inform. 2015;14:95–103. [Google Scholar] [PubMed]
92. De Bruijn SE, Fadaie Z, Cremers FPM, Kremer H, Roosing S. The impact of modern technologies on molecular diagnostic success rates, with a focus on inherited retinal dystrophy and hearing loss. Int J Mol Sci. 2021;22(6):2943. doi:10.3390/ijms22062943. [Google Scholar] [PubMed] [CrossRef]
93. Gilissen C, Hoischen A, Brunner HG, Veltman JA. Disease gene identification strategies for exome sequencing. Eur J Hum Genet. 2012;20(5):490–7. doi:10.1038/ejhg.2011.258. [Google Scholar] [PubMed] [CrossRef]
94. Torkamani A, Pham P, Libiger O, Bansal V, Zhang G, Scott-Van Zeeland AA, et al. Clinical implications of human population differences in genome-wide rates of functional genotypes. Front Gene. 2012;3:211. doi:10.3389/fgene.2012.00211. [Google Scholar] [PubMed] [CrossRef]
95. Londin E, Yadav P, Surrey S, Kricka LJ, Fortina P. Use of linkage analysis, genome-wide association studies, and next-generation sequencing in the identification of disease-causing mutations. Methods Mol Biol. 2013;1015:127–46. doi:10.1007/978-1-62703-435-7_8. [Google Scholar] [PubMed] [CrossRef]
96. Javaid M, Haleem A, Singh RP, Suman R, Rab S. Significance of machine learning in healthcare: features, pillars and applications. Int J Intell Netw. 2022;3:58–73. doi:10.1016/j.ijin.2022.05.002. [Google Scholar] [CrossRef]
97. Badrulhisham F, Pogatzki-Zahn E, Segelcke D, Spisak T, Vollert J. Machine learning and artificial intelligence in neuroscience: a primer for researchers. Brain Behav Immun. 2023;115:470–9. doi:10.1016/j.bbi.2023.11.005. [Google Scholar] [PubMed] [CrossRef]
98. Wang J, Qin Z, Hsu J, Zhou B. A fusion of machine learning algorithms and traditional statistical forecasting models for analyzing American healthcare expenditure. Healthc Anal. 2024;5:100312. doi:10.1016/j.health.2024.100312. [Google Scholar] [CrossRef]
99. Baseer K, Sivakumar K, Veeraiah D, Chhabra G, Kumar Lakineni P, Jahir Pasha M, et al. Healthcare diagnostics with an adaptive deep learning model integrated with the Internet of Medical Things (IoMT) for predicting heart disease. Biomed Signal Process Control. 2024;92:105988. doi:10.1016/j.bspc.2024.105988. [Google Scholar] [CrossRef]
100. Jamrat S, Sukasem C, Sratthaphut L, Hongkaew Y, Samanchuen T. A precision medicine approach to personalized prescribing using genetic and nongenetic factors for clinical decision-making. Comput Biol Med. 2023;165:107329. doi:10.1016/j.compbiomed.2023.107329. [Google Scholar] [PubMed] [CrossRef]
101. Lax DN, White S, Manning P, Kabbouche Samaha M. Diagnostic algorithm for pediatric headaches: a clinical improvement initiative. Pediatr Neurol. 2023;149:108–13. doi:10.1016/j.pediatrneurol.2023.09.009. [Google Scholar] [PubMed] [CrossRef]
102. Munquad S, Das AB. Uncovering the subtype-specific disease module and the development of drug response prediction models for glioma. Heliyon. 2024;10(5):e27190. doi:10.1016/j.heliyon.2024.e27190. [Google Scholar] [PubMed] [CrossRef]
103. Gönül S, Namlı T, Coşar A, Toroslu İH. A reinforcement learning based algorithm for personalization of digital, just-in-time, adaptive interventions. Artif Intell Med. 2021;115:102062. doi:10.1016/j.artmed.2021.102062. [Google Scholar] [PubMed] [CrossRef]
104. Castro DC, Walker I, Glocker B. Causality matters in medical imaging. Nat Commun. 2020;11:3673. doi:10.1038/s41467-020-17478-w. [Google Scholar] [PubMed] [CrossRef]
105. Hamal S, Mishra BK, Baldock R, Sayers W, Adhikari TN, Gibson RM. A comparative analysis of machine learning algorithms for detecting COVID-19 using lung X-ray images. Decis Anal J. 2024;11:100460. doi:10.1016/j.dajour.2024.100460. [Google Scholar] [CrossRef]
106. Wong KK, Ayoub M, Cao Z, Chen C, Chen W, Ghista DN, et al. The synergy of cybernetical intelligence with medical image analysis for deep medicine: a methodological perspective. Comput Methods Programs Biomed. 2023;240:107677. doi:10.1016/j.cmpb.2023.107677. [Google Scholar] [PubMed] [CrossRef]
107. Huang S, Jin K, Gao Z, Yang B, Shi X, Zhou J, et al. Automated interpretation of retinal vein occlusion based on fundus fluorescein angiography images using deep learning: a retrospective, multi-center study. Heliyon. 2024;10(13):e33108. doi:10.1016/j.heliyon.2024.e33108. [Google Scholar] [PubMed] [CrossRef]
108. Jiang J, Peng J, Hu C, Jian W, Wang X, Liu W. Breast cancer detection and classification in mammogram using a three-stage deep learning framework based on PAA algorithm. Artif Intell Med. 2022;134:102419. doi:10.1016/j.artmed.2022.102419. [Google Scholar] [PubMed] [CrossRef]
109. García-Gutiérrez F, Hernández-Lorenzo L, Cabrera-Martín MN, Matias-Guiu JA, Ayala JL. Predicting changes in brain metabolism and progression from mild cognitive impairment to dementia using multitask deep learning models and explainable AI. Neuroimage. 2024;297:120695. doi:10.1016/j.neuroimage.2024.120695. [Google Scholar] [PubMed] [CrossRef]
110. Park DJ, Park MW, Lee H, Kim YJ, Kim Y, Park YH. Development of machine learning model for diagnostic disease prediction based on laboratory tests. Sci Rep. 2021 Apr 7;11(1):7567. doi:10.1038/s41598-021-87171-5. [Google Scholar] [PubMed] [CrossRef]
111. Zeleke AJ, Palumbo P, Tubertini P, Miglio R, Chiari L. Comparison of nine machine learning regression models in predicting hospital length of stay for patients admitted to a general medicine department. Inform Med Unlocked. 2023;47:101499. doi:10.1016/j.imu.2024.101499. [Google Scholar] [CrossRef]
112. Zheng Y, Zhang C, Liu Y. Risk prediction models of depression in older adults with chronic diseases. J Affect Disord. 2024;359:182–8. doi:10.1016/j.jad.2024.05.078. [Google Scholar] [PubMed] [CrossRef]
113. Loutati R, Ben-Yehuda A, Rosenberg S, Rottenberg Y. Multimodal machine learning for prediction of 30-day readmission risk in elderly population. Am J Med. 2024;137(7):617–28. doi:10.1016/j.amjmed.2024.04.002. [Google Scholar] [PubMed] [CrossRef]
114. Roster K, Connaughton C, Rodrigues FA. Forecasting new diseases in low-data settings using transfer learning. Chaos Solit Fractals. 2022;161:112306. doi:10.1016/j.chaos.2022.112306. [Google Scholar] [PubMed] [CrossRef]
115. Zhang M, Yan K, Chen Y, Yu R. Anticipating interpersonal sensitivity: a predictive model for early intervention in psychological disorders in college students. Comput Biol Med. 2024;172:108134. doi:10.1016/j.compbiomed.2024.108134. [Google Scholar] [PubMed] [CrossRef]
116. Deep learning for detection of diabetic eye disease. Google Research Blog, USA. Available from: http://research.google/blog/deep-learning-for-detection-of-diabetic-eye-disease/. [Accessed 2024]. [Google Scholar]
117. How Watson for Oncology is advancing personalized patient care. The ASCO Post. The ASCO Post, USA. Available from: https://ascopost.com/issues/june-25-2017/how-watson-for-oncology-is-advancing-personalized-patient-care/. [Accessed 2024]. [Google Scholar]
118. Detecting diabetic eye disease with machine learning. Google Blog. Available from: https://blog.google/technology/ai/detecting-diabetic-eye-disease-machine-learning/. [Accessed 2024]. [Google Scholar]
119. Li Z, Derksen H, Gryak J, Jiang C, Gao Z, Zhang W, et al. Prediction of cardiac arrhythmia using deterministic probabilistic finite-state automata. Biomed Signal Process Control. 2020;63:102200. doi:10.1016/j.bspc.2020.102200. [Google Scholar] [CrossRef]
120. Al Meslamani AZ, Sobrino I. Machine learning in infectious diseases: potential applications and limitations. Ann Med. 2024;56(1). doi:10.1080/07853890.2024.2362869. [Google Scholar] [PubMed] [CrossRef]
121. Hendy LE, Spees LP, Tak C, Carpenter DM, Thomas KC, Roberts MC. An evaluation of the cost-effectiveness of population genetic screening for familial hypercholesterolemia in US patients. Atherosclerosis. 2024;393:117541. doi:10.1016/j.atherosclerosis.2024.117541. [Google Scholar] [PubMed] [CrossRef]
122. Ardila D, Kiraly AP, Bharadwaj S, Choi B, Reicher JJ, Peng L, et al. End-to-end lung cancer screening with three-dimensional deep learning on low-dose chest computed tomography. Nat Med. 2019;25(6):954–61. doi:10.1038/s41591-019-0447-x. [Google Scholar] [PubMed] [CrossRef]
123. Taroni JN, Grayson PC, Hu Q, Eddy S, Kretzler M, Merkel PA, et al. MultiPLIER: a transfer learning framework for transcriptomics reveals systemic features of rare disease. Cell Syst. 2019;8(5):380–94.e4. doi:10.1016/j.cels.2019.04.003. [Google Scholar] [PubMed] [CrossRef]
124. Anitha A, Banerjee M, Thanseem I, Prakash A, Melempatt N, SP S, et al. Rare pathogenic variants identified in whole exome sequencing of monozygotic twins with autism spectrum disorder. Pediatr Neurol. 2024 Sep 1;158:113–23. doi:10.1016/j.pediatrneurol.2024.06.003. [Google Scholar] [PubMed] [CrossRef]
125. Mei J, Yan H, Tang Z, Piao Z, Yuan Y, Dou Y, et al. Deep learning algorithm applied to plain CT images to identify superior mesenteric artery abnormalities. Eur J Radiol. 2024;173:111388. doi:10.1016/j.ejrad.2024.111388. [Google Scholar] [PubMed] [CrossRef]
126. Aljohani M, Bahgat WM, Balaha HM, AbdulAzeem Y, El-Abd M, Badawy M, et al. An automated metaheuristic-optimized approach for diagnosing and classifying brain tumors based on a convolutional neural network. Results Eng. 2024;23:102459. doi:10.1016/j.rineng.2024.102459. [Google Scholar] [CrossRef]
127. Huang AA, Huang SY. Computation of the distribution of model accuracy statistics in machine learning: comparison between analytically derived distributions and simulation-based methods. Health Sci Rep. 2023;6(4):467. doi:10.1002/hsr2.1214. [Google Scholar] [PubMed] [CrossRef]
128. Sidey-Gibbons J, Sidey-Gibbons C. Machine learning in medicine: a practical introduction. BMC Med Res Methodol. 2019;19:64. doi:10.1186/s12874-019-0681-4. [Google Scholar] [PubMed] [CrossRef]
129. Samuelson FW. Inference based on diagnostic measures from studies of new imaging devices. Acad Radiol. 2013;20(7):816–24. doi:10.1016/j.acra.2013.03.002. [Google Scholar] [PubMed] [CrossRef]
130. Erickson BJ, Korfiatis P, Akkus Z, Kline TL. Machine learning for medical imaging. Radiographics. 2017;37(2):505–15. doi:10.1148/rg.2017160130. [Google Scholar] [PubMed] [CrossRef]
131. Müller D, Soto-Rey I, Kramer F. Towards a guideline for evaluation metrics in medical image segmentation. BMC Res Notes. 2022;15:210. doi:10.1186/s13104-022-06096-y. [Google Scholar] [PubMed] [CrossRef]
132. Ahmad R. Reviewing the relationship between machines and radiology: the application of artificial intelligence. Acta Radiol Open. 2021;10(2):124. doi:10.1177/2058460121990296. [Google Scholar] [PubMed] [CrossRef]
133. Balki I, Amirabadi A, Levman J, Martel AL, Emersic Z, Meden B, et al. Sample-size determination methodologies for machine learning in medical imaging research: a systematic review. Can Assoc Radiol J. 2019;70(4):344–53. doi:10.1016/j.carj.2019.06.002. [Google Scholar] [PubMed] [CrossRef]
134. Olyanasab A, Annabestani M. Leveraging machine learning for personalized wearable biomedical devices: a review. J Pers Med. 2024;14(2):203. doi:10.3390/jpm14020203. [Google Scholar] [PubMed] [CrossRef]
135. Yang Y. Application of wearable devices based on artificial intelligence sensors in sports human health monitoring. Meas Sens. 2024;33:101086. doi:10.1016/j.measen.2024.101086. [Google Scholar] [CrossRef]
136. Yang Q, Al Mamun A, Reza MNH, Naznen F. Modelling the significance of value-belief-norm framework to predict mass adoption potentials of internet of things-enabled wearable fitness devices. Heliyon. 2024;10(9):e30179. doi:10.1016/j.heliyon.2024.e30179. [Google Scholar] [PubMed] [CrossRef]
137. Verma RP, Sahu PS, Mishra S, Dabhade A, Saha B. Antibacterial highly sensitive eco-friendly wearable piezoresistive sensor for monitoring multiple physiological parameters. Sens Actuators B: Chem. 2024;405:135288. doi:10.1016/j.snb.2024.135288. [Google Scholar] [CrossRef]
138. Utsha UT, Morshed BI. CardioHelp: a smartphone application for beat-by-beat ECG signal analysis for real-time cardiac disease detection using edge-computing AI classifiers. Smart Health. 2024;31:100446. doi:10.1016/j.smhl.2024.100446. [Google Scholar] [CrossRef]
139. Walter JR, Xu S, Rogers JA. From lab to life: how wearable devices can improve health equity. Nat Commun. 2024;15:123. doi:10.1038/s41467-023-44634-9. [Google Scholar] [PubMed] [CrossRef]
140. Nneka OO, Okafor KC, Nwabueze CA, Mbachu CB, Iloh J, Chinebu TI, et al. A computational fractional order model for optimal control of wearable healthcare monitoring devices for maternal health. Healthc Anal. 2024;5:100308. doi:10.1016/j.health.2024.100308. [Google Scholar] [CrossRef]
141. Riaz MS, Shaukat M, Saeed T, Ijaz A, Qureshi HN, Posokhova I, et al. IPREDICT: AI enabled proactive pandemic prediction using biosensing wearable devices. Inform Med Unlocked. 2023;46:101478. doi:10.1016/j.imu.2024.101478. [Google Scholar] [CrossRef]
142. Almalawi A, Zafar A, Unhelkar B, Hassan S, Alqurashi F, Khan AI, et al. Enhancing security in smart healthcare systems: using intelligent edge computing with a novel Salp Swarm optimization and radial basis neural network algorithm. Heliyon. 2024;10(13):e33792. doi:10.1016/j.heliyon.2024.e33792. [Google Scholar] [PubMed] [CrossRef]
143. Shastry KA, Shastry A. An integrated deep learning and natural language processing approach for continuous remote monitoring in digital health. Decis Anal J. 2023;8:100301. doi:10.1016/j.dajour.2023.100301. [Google Scholar] [CrossRef]
144. Chang V, Doan LMT, Xu QA, Hall K, Wang YA, Kamal MM. Digitalization in omnichannel healthcare supply chain businesses: the role of smart wearable devices. J Bus Res. 2023;156:113369. doi:10.1016/j.jbusres.2022.113369. [Google Scholar] [CrossRef]
145. Hayat N, Salameh AA, Mamun AA, Alam SS, Zainol NR. Exploring the mass adoption potential of wearable fitness devices in Malaysia. Digit Health. 2023;9:1. doi:10.1177/20552076231180728. [Google Scholar] [PubMed] [CrossRef]
146. Lu JK, Wang W, Goh J, Maier AB. A practical guide for selecting continuous monitoring wearable devices for community-dwelling adults. Heliyon. 2024;10(13):e33488. doi:10.1016/j.heliyon.2024.e33488. [Google Scholar] [PubMed] [CrossRef]
147. Deepak G, Sharma P, Jayachitra S, Chepur J, Srihari T, Judgi T. Privacy-preserving deep learning approaches for effective utilization of wearable health data. Meas Sens. 2024;33:101238. doi:10.1016/j.measen.2024.101238. [Google Scholar] [CrossRef]
148. Tanaka M, Ishii S, Matsuoka A, Tanabe S, Matsunaga S, Rahmani A, et al. Perspectives of Japanese elders and their healthcare providers on use of wearable technology to monitor their health at home: a qualitative exploration. Int J Nurs Stud. 2024;152:104691. doi:10.1016/j.ijnurstu.2024.104691. [Google Scholar] [PubMed] [CrossRef]
149. Yin S, Yu Z, Song N, Guo Z, Li W, Ma J, et al. A long lifetime and highly sensitive wearable microneedle sensor for the continuous real-time monitoring of glucose in interstitial fluid. Biosens Bioelectron. 2024;244:115822. doi:10.1016/j.bios.2023.115822. [Google Scholar] [PubMed] [CrossRef]
150. Ma D, Li L, Shi W, Li M, Zhang J, Fan Y, et al. Multimodal coupling and HRV assessment characterize autonomic functional changes in congestive heart failure patients with sinus rhythm or severe arrhythmia. Biomed Signal Process Control. 2024;89:105764. doi:10.1016/j.bspc.2023.105764. [Google Scholar] [CrossRef]
151. Sestino A, D’Angelo A. Elderly patients’ reactions to gamification-based digital therapeutics (DTxthe relevance of socialization tendency seeking. Technol Forecast Soc Change. 2024;205:123526. doi:10.1016/j.techfore.2024.123526. [Google Scholar] [CrossRef]
152. Mohammed ZK, Mohammed MA, Abdulkareem KH, Zebari DA, Lakhan A, Marhoon HA, et al. A metaverse framework for IoT-based remote patient monitoring and virtual consultations using AES-256 encryption. Appl Soft Comput. 2024;158:111588. doi:10.1016/j.asoc.2024.111588. [Google Scholar] [CrossRef]
153. Sonavane A, Khamparia A, Gupta D. A systematic review on the internet of medical things: techniques, open issues, and future directions. Comput Model Eng Sci. 2023;137(2):1525–50. doi:10.32604/cmes.2023.028203. [Google Scholar] [CrossRef]
154. Lee SY, Lee K. Factors that influence an individual’s intention to adopt a wearable healthcare device: the case of a wearable fitness tracker. Technol Forecast Soc Change. 2018;129:154–63. doi:10.1016/j.techfore.2018.01.002. [Google Scholar] [CrossRef]
155. Zovko K, Šerić L, Perković T, Belani H, Šolić P. IoT and health monitoring wearable devices as enabling technologies for sustainable enhancement of life quality in smart environments. J Clean Prod. 2023;413:137506. doi:10.1016/j.jclepro.2023.137506. [Google Scholar] [CrossRef]
156. Hadid A, McDonald EG, Cheng MP, Papenburg J, Libman M, Dixon PC, et al. The WE SENSE study protocol: a controlled, longitudinal clinical trial on the use of wearable sensors for early detection and tracking of viral respiratory tract infections. Contemp Clin Trials. 2023;128:107103. doi:10.1016/j.cct.2023.107103. [Google Scholar] [PubMed] [CrossRef]
157. Ahn J, Yang Y, Park G. Advancing elderly diabetes care: exploring the usability and acceptance of continuous glucose monitoring (CGM). Geriatr Nurs. 2024;59:15–25. doi:10.1016/j.gerinurse.2024.06.041. [Google Scholar] [PubMed] [CrossRef]
158. Bernard L, Valsecchi V, Mura T, Aouinti S, Padern G, Ferreira R, et al. Management of patients with rheumatoid arthritis by telemedicine: connected monitoring. A randomized controlled trial. Joint Bone Spine. 2022;89(5):105368. doi:10.1016/j.jbspin.2022.105368. [Google Scholar] [PubMed] [CrossRef]
159. Triebwasser JE, Hesson A, Langen ES. A randomized-controlled trial to assess the effect of ibuprofen on postpartum blood pressure in women with hypertensive disorders of pregnancy. Pregnancy Hypertens. 2019;18:117–21. doi:10.1016/j.preghy.2019.09.012. [Google Scholar] [PubMed] [CrossRef]
160. Bosnic-Anticevich S, Bender BG, Shuler MT, Hess M, Kocks JW. Recognizing and tackling inhaler technique decay in asthma and chronic obstructive pulmonary disease (COPD) clinical practice. J Allergy Clin Immunol Pract. 2023;11(8):2355–64.e5. doi:10.1016/j.jaip.2023.04.031. [Google Scholar] [PubMed] [CrossRef]
161. Chan M, Estève D, Fourniols J, Escriba C, Campo E. Smart wearable systems: current status and future challenges. Artif Intell Med. 2012;56(3):137–56. doi:10.1016/j.artmed.2012.09.003. [Google Scholar] [PubMed] [CrossRef]
162. Kinny F, Schlottau S, Ali Sherazi B, Obarcanin E, Läer S. Digital health in pharmacy education: elective practical course integrating wearable devices and their generated health data. Explor Res Clin Soc Pharm. 2024;15:100465. doi:10.1016/j.rcsop.2024.100465. [Google Scholar] [PubMed] [CrossRef]
163. Lim JY, Yu H, Kwon YE, Do JG, Hwang JH. Feasibility of digital technology-supported home exercise intervention for health promotion in community-dwelling older adults: a pilot randomized controlled trial. Heliyon. 2024;10(3):e24933. doi:10.1016/j.heliyon.2024.e24933. [Google Scholar] [PubMed] [CrossRef]
164. Esmaeilzadeh P. Challenges and strategies for wide-scale artificial intelligence (AI) deployment in healthcare practices: a perspective for healthcare organizations. Artif Intell Med. 2024;151:102861. doi:10.1016/j.artmed.2024.102861. [Google Scholar] [PubMed] [CrossRef]
165. Teng XF, Zhang YT, Poon CC, Bonato P. Wearable medical systems for p-Health. IEEE Rev Biomed Eng. 2008 Dec 12;1:62–74. doi:10.1109/RBME.2008.2008248. [Google Scholar] [PubMed] [CrossRef]
166. Babu M, Lautman Z, Lin X, Sobota MHB, Snyder MP. Wearable devices: implications for precision medicine and the future of health care. Annu Rev Med. 2024;75:401–15. doi:10.1146/annurev-med-052422-020437. [Google Scholar] [PubMed] [CrossRef]
167. Sharma A, Badea M, Tiwari S, Marty JL. Wearable biosensors: an alternative and practical approach in healthcare and disease monitoring. Molecules. 2021;26(3):748. doi:10.3390/molecules26030748. [Google Scholar] [PubMed] [CrossRef]
168. Yin H, Jha NK. A health decision support system for disease diagnosis based on wearable medical sensors and machine learning ensembles. IEEE Trans Multiscale Comput Syst. 2017 May 31;3(4):228–41. doi:10.1109/TMSCS.2017.2710194. [Google Scholar] [CrossRef]
169. Koydemir HC, Ozcan A. Wearable and implantable sensors for biomedical applications. Annu Rev Anal Chem. 2018;11(1):127–46. doi:10.1146/annurev-anchem-061417-125956. [Google Scholar] [PubMed] [CrossRef]
170. El-Amrawy F, Nounou MI. Are currently available wearable devices for activity tracking and heart rate monitoring accurate, precise, and medically beneficial? Healthc Inform Res. 2015;21(4):315–20. doi:10.4258/hir.2015.21.4.315. [Google Scholar] [PubMed] [CrossRef]
171. Baumgartner R, Arora P, Bath C, Burljaev D, Ciereszko K, Custers B, et al. Fair and equitable AI in biomedical research and healthcare: social science perspectives. Artif Intell Med. 2023;144:102658. doi:10.1016/j.artmed.2023.102658. [Google Scholar] [PubMed] [CrossRef]
172. Yu K, Sang QA, Lung PY, Tan W, Lively T, Sheffield C, et al. Personalized chemotherapy selection for breast cancer using gene expression profiles. Sci Rep. 2017 Mar 3;7:43294. doi:10.1038/srep43294. [Google Scholar] [PubMed] [CrossRef]
173. Abbasi A, Alexandrov LB. Significance and limitations of the use of next-generation sequencing technologies for detecting mutational signatures. DNA Repair. 2021;107:103200. doi:10.1016/j.dnarep.2021.103200. [Google Scholar] [PubMed] [CrossRef]
174. Zhang W, Wang W, Xu Y, Wu K, Shi J, Li M, et al. Prediction of epidermal growth factor receptor mutation subtypes in non-small cell lung cancer from hematoxylin and eosin-stained slides using deep learning. Lab Invest. 2024;104(8):102094. doi:10.1016/j.labinv.2024.102094. [Google Scholar] [PubMed] [CrossRef]
175. Cavallaro C, Cutello V, Pavone M, Zito F. Machine learning and genetic algorithms: a case study on image reconstruction. Knowl-Based Syst. 2024;284:111194. doi:10.1016/j.knosys.2023.111194. [Google Scholar] [CrossRef]
176. Yoo S, Gujrathi I, Haider MA, Khalvati F. Prostate cancer detection using deep convolutional neural networks. Sci Rep. 2019 Dec 20;9(1):19518. doi:10.1038/s41598-019-55972-4. [Google Scholar] [PubMed] [CrossRef]
177. Yim J, Chopra R, Fauw JD, Ledsam J. Using AI to predict retinal disease progression. Google DeepMind Blog, USA. Available from: https://deepmind.google/discover/blog/using-ai-to-predict-retinal-disease-progression/. [Accessed 2024]. [Google Scholar]
178. Rajan R, Kumar S. IoT based optical coherence tomography retinal images classification using OCT Deep Net2. Meas Sens. 2024;25:100652. doi:10.1016/j.measen.2022.100652. [Google Scholar] [CrossRef]
179. Bousquet A, Conrad WH, Sadat SO, Vardanyan N, Hong Y. Deep learning forecasting using time-varying parameters of the SIRD model for COVID-19. Sci Rep. 2022 Feb 22;12(1):3030. doi:10.1038/s41598-022-06992-0. [Google Scholar] [PubMed] [CrossRef]
180. Coskun A, Ertaylan G, Pusparum M, Van Hoof R, Kaya ZZ, Khosravi A, et al. Advancing personalized medicine: integrating statistical algorithms with omics and nano-omics for enhanced diagnostic accuracy and treatment efficacy. Biochim Biophys Acta Mol Basis Dis. 2024;1870(7):167339. doi:10.1016/j.bbadis.2024.167339. [Google Scholar] [PubMed] [CrossRef]
181. Horgan D, Van den Bulcke M, Malapelle U, Troncone G, Normanno N, Capoluongo ED, et al. Tackling the implementation gap for the uptake of NGS and advanced molecular diagnostics into healthcare systems. Heliyon. 2024;10(1):e23914. doi:10.1016/j.heliyon.2023.e23914. [Google Scholar] [PubMed] [CrossRef]
182. Su J, Yang L, Sun Z, Zhan X. Personalized drug therapy: innovative concept guided with proteoformics. Mol Cell Proteomics. 2024;23(3):100737. doi:10.1016/j.mcpro.2024.100737. [Google Scholar] [PubMed] [CrossRef]
183. Johnson KB, Wei W-Q, Weeraratne D, Frisse ME, Misulis K, Rhee K, et al. Precision medicine, AI, and the future of personalized health care. Clin Transl Sci. 2020;14(1):86–93. doi:10.1111/cts.12884. [Google Scholar] [PubMed] [CrossRef]
184. Kabata D, Saeki N, Kajimoto K, Tanaka R, Kakiuchi N, Shintani A, et al. Health guidance intervention mitigates the escalation of predicted comprehensive cardiovascular disease risk: interrupted time series design. Prev Med. 2024;183:107972. doi:10.1016/j.ypmed.2024.107972. [Google Scholar] [PubMed] [CrossRef]
185. Nasr S, Li L, Asad M, Moridi M, Wang M, Zemp FJ, et al. A computational pipeline for identifying gene targets and signaling pathways in cancer cells to improve lymphocyte infiltration and immune checkpoint therapy efficacy. eBioMedicine. 2024;104:105167. doi:10.1016/j.ebiom.2024.105167. [Google Scholar] [PubMed] [CrossRef]
186. Janiga G, Daróczy L, Berg P, Thévenin D, Skalej M, Beuing O. An automatic CFD-based flow diverter optimization principle for patient-specific intracranial aneurysms. J Biomech. 2015;48(14):3846–52. doi:10.1016/j.jbiomech.2015.09.039. [Google Scholar] [PubMed] [CrossRef]
187. Turk PJ, Anderson WE, Burns RJ, Chou S, Dobbs TE, Kearns JT, et al. A regionally tailored epidemiological forecast and monitoring program to guide a healthcare system in the COVID-19 pandemic. J Infect Public Health. 2024;17(6):1125–33. doi:10.1016/j.jiph.2024.04.014. [Google Scholar] [PubMed] [CrossRef]
188. Sarhaddi F, Azimi I, Labbaf S, Niela-Vilén H, Dutt N, Axelin A, et al. Long-term IoT-based maternal monitoring: system design and evaluation. Sensors. 2021;21(7):2281. doi:10.3390/s21072281. [Google Scholar] [PubMed] [CrossRef]
189. Deng Y, Wang C, Qiu T, Ni J, Xuan W, Chen J, et al. Large-area stretchable dynamic 18-lead ECG monitoring patch integrated with deep learning for cardiovascular disease diagnosis. Cell Rep Phys Sci. 2024;5:102077. doi:10.1016/j.xcrp.2024.102077. [Google Scholar] [CrossRef]
190. Rohr-Fukuma M, Stieglitz LH, Bujan B, Jedrysiak P, Oertel MF, Salzmann L, et al. Neurofeedback-enabled beta power control with a fully implanted DBS system in patients with Parkinson’s disease. Clin Neurophysiol. 2024;165:1–15. doi:10.1016/j.clinph.2024.06.001. [Google Scholar] [PubMed] [CrossRef]
191. Heller CA, Michelassi F. Forging successful interdisciplinary research collaborations: a nationwide survey of departments of surgery. Surgery. 2012;151(4):502–9. doi:10.1016/j.surg.2011.09.034. [Google Scholar] [PubMed] [CrossRef]
192. Mike K, Nemirovsky-Rotman S, Hazzan O. Interdisciplinary education—the case of biomedical signal processing. In: 2020 IEEE Global Engineering Education Conference (EDUCONIEEE; 2020 Apr 27; IEEE; p. 339–43. doi:10.1109/EDUCON45650.2020.9125200. [Google Scholar] [CrossRef]
193. Spira A, Coleman D. Impact of an interdisciplinary computational research section in a department of medicine: an 8-year perspective. Am J Med. 2018;131(7):846–51. doi:10.1016/j.amjmed.2018.03.008. [Google Scholar] [PubMed] [CrossRef]
194. Cassel LN, Wolz U. Interdisciplinary computing, successes and challenges (abstract only). In: Proceedings of the 44th ACM Technical Symposium on Computer Science Education (SIGCSE ’132013; Denver, CO, USA, ACM Digital Library; p. 738. doi:10.1145/2445196.2445438. [Google Scholar] [CrossRef]
195. Golecki HM, Amos JR, Bradley J. Designing capstone experiences for interdisciplinarity in biomedical engineering education. In: 2023 ASEE Annual Conference & Exposition, American Society for Engineering Education; 2023. doi:10.18260/1-2—42977. [Google Scholar] [CrossRef]
196. Munshi R, Coalson RD, Ermentrout GB, Madura JD, Meirovitch H, Stiles JR, et al. An introduction to simulation and visualization of biological systems at multiple scales: a summer training program for interdisciplinary research. Biotechnol Progress. 2006;22(1):179–85. doi:10.1021/bp0501773. [Google Scholar] [PubMed] [CrossRef]
197. León A, Pastor Ó. Enhancing precision medicine: a big data-driven approach for the management of genomic data. Big Data Res. 2021;26:100253. doi:10.1016/j.bdr.2021.100253. [Google Scholar] [CrossRef]
198. Neau H, Ansart R, Baudry C, Fournier Y, Mérigoux N, Koren C, et al. HPC challenges and opportunities of industrial-scale reactive fluidized bed simulation using meshes of several billion cells on the route of Exascale. Powder Technol. 2024;444:120018. doi:10.1016/j.powtec.2024.120018. [Google Scholar] [CrossRef]
199. Rebba R, Mahadevan S, Huang S. Validation and error estimation of computational models. Reliab Eng Syst Saf. 2006;91(10–11):1390–7. doi:10.1016/j.ress.2005.11.035. [Google Scholar] [CrossRef]
200. González-Soto M, Díaz-Redondo RP, Fernández-Veiga M, Fernández-Castro B, Fernández-Vilas A. Decentralized and collaborative machine learning framework for IoT. Comput Netw. 2024;239:110137. doi:10.1016/j.comnet.2023.110137. [Google Scholar] [CrossRef]
201. Lee T, Kim S, Lee J, Jun C. HarmoSATE: harmonized embedding-based self-attentive encoder to improve accuracy of privacy-preserving federated predictive analysis. Inf Sci. 2024;662:120265. doi:10.1016/j.ins.2024.120265. [Google Scholar] [CrossRef]
202. Kumar S, Deepika D, Slater K, Kumar V. AOPWIKI-EXPLORER: an interactive graph-based query engine leveraging large language models. Comput Toxicol. 2024;30:100308. doi:10.1016/j.comtox.2024.100308. [Google Scholar] [CrossRef]
203. Maqsood K, Hagras H, Zabet NR. An overview of artificial intelligence in the field of genomics. Discov Artif Intell. 2024;4:9. doi:10.1007/s44163-024-00103-w. [Google Scholar] [CrossRef]
204. Mayo CS, Feng MU, Brock KK, Kudner R, Balter P, Buchsbaum JC, et al. Operational ontology for oncology (O3a professional society-based, multistakeholder, consensus-driven informatics standard supporting clinical and research use of real-world data from patients treated for cancer. Int J Radiat Oncol Biol Phys. 2023;117(3):533–50. doi:10.1016/j.ijrobp.2023.05.033. [Google Scholar] [PubMed] [CrossRef]
205. Nambiar A, Mundra D. An overview of data warehouse and data lake in modern enterprise data management. Big Data Cogn Comput. 2022;6(4):132. doi:10.3390/bdcc6040132. [Google Scholar] [CrossRef]
206. Singhal A, Neveditsin N, Tanveer H, Mago V. Toward fairness, accountability, transparency, and ethics in AI for social media and health care: scoping review. JMIR Med Inform. 2024 Apr 3;12:e50048. doi:10.2196/50048. [Google Scholar] [PubMed] [CrossRef]
207. Kirat T, Tambou O, Do V, Tsoukiàs A. Fairness and explainability in automatic decision-making systems: a challenge for computer science and law. EURO J Decis Process. 2022;11:100036. doi:10.1016/j.ejdp.2023.100036. [Google Scholar] [CrossRef]
208. Galetsi P, Katsaliaki K, Kumar S. Exploring benefits and ethical challenges in the rise of mHealth (mobile healthcare) technology for the common good: an analysis of mobile applications for health specialists. Technovation. 2023;121:102598. doi:10.1016/j.technovation.2022.102598. [Google Scholar] [CrossRef]
209. Fedele A, Punzi C, Tramacere S. The ALTAI checklist as a tool to assess ethical and legal implications for a trustworthy AI development in education. Comput Law Secur Rev. 2024;53:105986. doi:10.1016/j.clsr.2024.105986. [Google Scholar] [CrossRef]
210. Daudén-Esmel C, Castellà-Roca J, Viejo A. Blockchain-based access control system for efficient and GDPR-compliant personal data management. Comput Commun. 2024;214:67–87. doi:10.1016/j.comcom.2023.11.017. [Google Scholar] [CrossRef]
211. da Silva RGL. The advancement of artificial intelligence in biomedical research and health innovation: challenges and opportunities in emerging economies. Global Health. 2024;20:44. doi:10.1186/s12992-024-01049-5. [Google Scholar] [PubMed] [CrossRef]
212. Kassem K, Sperti M, Cavallo A, Vergani AM, Fassino D, Moz M, et al. An innovative artificial intelligence-based method to compress complex models into explainable, model-agnostic and reduced decision support systems with application to healthcare (NEAR). Artif Intell Med. 2024;151:102841. doi:10.1016/j.artmed.2024.102841. [Google Scholar] [PubMed] [CrossRef]
213. Huang Y, Guo J, Chen W, Lin H, Tang H, Wang F, et al. A scoping review of fair machine learning techniques when using real-world data. J Biomed Inform. 2024;151:104622. doi:10.1016/j.jbi.2024.104622. [Google Scholar] [PubMed] [CrossRef]
214. Shi H, Kowalczewski A, Vu D, Liu X, Salekin A, Yang H, et al. Organoid intelligence: integration of organoid technology and artificial intelligence in the new era of in vitro models. Med Nov Technol Devices. 2024;21:100276. doi:10.1016/j.medntd.2023.100276. [Google Scholar] [PubMed] [CrossRef]
215. Algarni AM, Malaiya YK. A consolidated approach for estimation of data security breach costs. In: 2016 2nd International Conference on Information Management (ICIM2016; London, UK; p. 26–39. doi:10.1109/INFOMAN.2016.7477530. [Google Scholar] [CrossRef]
216. Yazijy S, Schölly R, Kellmeyer P. Towards a toolbox for privacy-preserving computation on health data. Stud Health Technol Inform. 2022;290:234–7. doi:10.3233/SHTI220069. [Google Scholar] [PubMed] [CrossRef]
217. Perakslis ED, Stanley M. A cybersecurity primer for translational research. Sci Transl Med. 2016;8(322):229. doi:10.1126/scitranslmed.aaa4493. [Google Scholar] [PubMed] [CrossRef]
218. Azencott CA. Machine learning and genomics: precision medicine versus patient privacy. Philos Trans R Soc A Math Phys Eng Sci. 2018;376(2128):20170350. doi:10.1098/rsta.2017.0350. [Google Scholar] [PubMed] [CrossRef]
219. Niranjana G, Chatterjee D. Security and privacy issues in biomedical AI systems and potential solutions. In: Handbook of artificial intelligence in biomedical engineering. 1st ed. CRC Press; 2021. [Google Scholar]
220. Uttarkar A, Niranjan V. Quantum synergy in peptide folding: a comparative study of CVaR-variational quantum eigensolver and molecular dynamics simulation. Int J Biol Macromol. 2024;273:133033. doi:10.1016/j.ijbiomac.2024.133033. [Google Scholar] [PubMed] [CrossRef]
221. Kapustina O, Burmakina P, Gubina N, Serov N, Vinogradov V. User-friendly and industry-integrated AI for medicinal chemists and pharmaceuticals. Artif Intell Chem. 2024;2:100072. doi:10.1016/j.aichem.2024.100072. [Google Scholar] [CrossRef]
222. Popoola O, Rodrigues M, Marchang J, Shenfield A, Ikpehai A, Popoola J. A critical literature review of security and privacy in smart home healthcare schemes adopting IoT & blockchain: problems, challenges and solutions. Blockchain Res Appl. 2024;5(2):100178. doi:10.1016/j.bcra.2023.100178. [Google Scholar] [CrossRef]
223. Chen Z, Peng B, Zhou Y, Hao Y, Xie X. A data-driven online calibration method for enhancing accuracy in biomedical tactile sensing. Measurement. 2024;236:115048. doi:10.1016/j.measurement.2024.115048. [Google Scholar] [CrossRef]
224. Xiao D, Song C, Nakamura N, Nakayama M. Development of an application concerning fast healthcare interoperability resources based on standardized structured medical information exchange version 2 data. Comput Methods Programs Biomed. 2021;208:106232. doi:10.1016/j.cmpb.2021.106232. [Google Scholar] [CrossRef]
225. Nasarian E, Alizadehsani R, Acharya U, Tsui K. Designing interpretable ML system to enhance trust in healthcare: a systematic review to proposed responsible clinician-AI-collaboration framework. Inf Fusion. 2024;108:102412. doi:10.1016/j.inffus.2024.102412. [Google Scholar] [CrossRef]
226. Ali S, Abuhmed T, El-Sappagh S, Muhammad K, Alonso-Moral JM, Confalonieri R, et al. Explainable Artificial Intelligence (XAIwhat we know and what is left to attain trustworthy Artificial Intelligence. Inf Fusion. 2023;99:101805. doi:10.1016/j.inffus.2023.101805. [Google Scholar] [CrossRef]
227. Sarker IH. Machine learning: algorithms, real-world applications and research directions. SN Comput Sci. 2021;2:160. doi:10.1007/s42979-021-00592-x. [Google Scholar] [PubMed] [CrossRef]
228. Jenkins DA, Martin GP, Sperrin M, Riley RD, Debray TPA, Collins GS, et al. Continual updating and monitoring of clinical prediction models: time for dynamic prediction systems? Diagn Progn Res. 2021 Jan 11;5(1):1. doi:10.1186/s41512-020-00090-3. [Google Scholar] [PubMed] [CrossRef]
229. Ramesh D, Mishra R, Atrey PK, Edla DR, Misra S, Qi L. Blockchain based efficient tamper-proof EHR storage for decentralized cloud-assisted storage. Alex Eng J. 2023;68:205–26. doi:10.1016/j.aej.2023.01.012. [Google Scholar] [CrossRef]
230. Mateus P, Moonen J, Beran M, Jaarsma E, Van der Landen SM, Heuvelink J, et al. Data harmonization and federated learning for multi-cohort dementia research using the OMOP common data model: a Netherlands consortium of dementia cohorts case study. J Biomed Inform. 2024;155:104661. doi:10.1016/j.jbi.2024.104661. [Google Scholar] [PubMed] [CrossRef]
231. Salvi M, Loh HW, Seoni S, Barua PD, García S, Molinari F, et al. Multi-modality approaches for medical support systems: a systematic review of the last decade. Inf Fusion. 2024;103:102134. doi:10.1016/j.inffus.2023.102134. [Google Scholar] [CrossRef]
232. Rigas ES, Lagakis P, Karadimas M, Logaras E, Latsou D, Hatzikou M, et al. Semantic interoperability for an AI-based applications platform for smart hospitals using HL7 FHIR. J Syst Softw. 2024;215:112093. doi:10.1016/j.jss.2024.112093. [Google Scholar] [CrossRef]
233. Vorisek CN, Lehne M, Klopfenstein SAI, Mayer PJ, Bartschke A, Haese T, et al. Fast Healthcare Interoperability Resources (FHIR) for interoperability in health research: systematic review. JMIR Med Inform. 2022 Jul 19;10(7):e35724. doi:10.2196/35724. [Google Scholar] [PubMed] [CrossRef]
234. Quinn P, Ellyne E, Yao C. Will the GDPR restrain health data access bodies under the European Health Data Space (EHDS)? Comput Law Secur Rev. 2024;54:105993. doi:10.1016/j.clsr.2024.105993. [Google Scholar] [CrossRef]
235. Kozel G, Gurses ME, Gecici NN, Gökalp E, Bahadir S, Merenzon MA, et al. Chat-GPT on brain tumors: an examination of artificial intelligence/machine learning’s ability to provide diagnoses and treatment plans for example neuro-oncology cases. Clin Neurol Neurosurg. 2024;239:108238. doi:10.1016/j.clineuro.2024.108238. [Google Scholar] [PubMed] [CrossRef]
236. Li Y, Yang R, Lu Y. A privacy risk identification framework of open government data: a mixed-method study in China. Gov Inf Q. 2024;41(1):101916. doi:10.1016/j.giq.2024.101916. [Google Scholar] [CrossRef]
237. Chen M, Esmaeilzadeh P. Adoption and use of various health information exchange methods for sending inside health information in US hospitals. Int J Med Inform. 2023;177:105156. doi:10.1016/j.ijmedinf.2023.105156. [Google Scholar] [PubMed] [CrossRef]
238. Painter JT, Raciborski RA, Matthieu MM, Oliver CM, Adkins DA, Garner KK. Engaging stakeholders to retrospectively discern implementation strategies to support program evaluation: proposed method and case study. Eval Program Plann. 2024;103:102398. doi:10.1016/j.evalprogplan.2023.102398. [Google Scholar] [PubMed] [CrossRef]
239. Sharp P, Jacks T, Hockfield S. Capitalizing on convergence for health care. Science. 2016;352(6293):1522–3. doi:10.1126/science.aag2350. [Google Scholar] [PubMed] [CrossRef]
240. Weber LM, Saelens W, Cannoodt R, Soneson C, Hapfelmeier A, Gardner PP, et al. Essential guidelines for computational method benchmarking. Genome Biol. 2019 Dec;20:1–2. doi:10.1186/s13059-019-1738-8. [Google Scholar] [PubMed] [CrossRef]
241. Massey JW, Liu C, Yılmaz AE. Benchmarking to close the credibility gap: a computational BioEM benchmark suite. In: 2016 URSI International Symposium on Electromagnetic Theory (EMTS2016; Espoo, Finland; p. 207–10. doi:10.1109/URSI-EMTS.2016.7571354. [Google Scholar] [CrossRef]
242. Mangul S, Martin LS, Hill BL, Lam AK, Distler MG, Zelikovsky A, et al. Systematic benchmarking of omics computational tools. Nat Commun. 2019 Mar 27;10(1):1393. doi:10.1038/s41467-019-09406-4. [Google Scholar] [PubMed] [CrossRef]
243. Ellrott K, Buchanan A, Creason A, Mason M, Schaffter T, Hoff B, et al. Reproducible biomedical benchmarking in the cloud: lessons from crowd-sourced data challenges. Genome Biol. 2019 Dec;20:1–9. doi:10.1186/s13059-019-1794-0. [Google Scholar] [PubMed] [CrossRef]
244. Peters B, Brenner SE, Wang E, Slonim D, Kann MG. Putting benchmarks in their rightful place: the heart of computational biology. PLoS Comput Biol. 2018;14(11):e1006494. doi:10.1371/journal.pcbi.1006494. [Google Scholar] [PubMed] [CrossRef]
245. Abdellatif AA, Mhaisen N, Mohamed A, Erbad A, Guizani M, Dawy Z, et al. Communication-efficient hierarchical federated learning for IoT heterogeneous systems with imbalanced data. Future Gener Comput Syst. 2022;128:406–19. doi:10.1016/j.future.2021.10.016. [Google Scholar] [CrossRef]
246. Yadav R, Pradeepa P, Srinivasan S, Rajora CS, Rajalakshmi R. A novel healthcare framework for ambient assisted living using the internet of medical things (IOMT) and deep neural network. Meas Sens. 2024;33:101111. doi:10.1016/j.measen.2024.101111. [Google Scholar] [CrossRef]
247. Li J, Han D, Weng T, Wu H, Li K, Castiglione A. A secure data storage and sharing scheme for port supply chain based on blockchain and dynamic searchable encryption. Comput Stand Interfaces. 2024;91:103887. doi:10.1016/j.csi.2024.103887. [Google Scholar] [CrossRef]
248. Ali S, Wadho SA, Yichiet A, Gan ML, Lee CK. Advancing cloud security: unveiling the protective potential of homomorphic secret sharing in secure cloud computing. Egypt Inform J. 2024;27:100519. doi:10.1016/j.eij.2024.100519. [Google Scholar] [CrossRef]
249. Sutradhar S, Majumder S, Bose R, Mondal H, Bhattacharyya D. A blockchain privacy-conserving framework for secure medical data transmission in the internet of medical things. Decis Anal J. 2024;10:100419. doi:10.1016/j.dajour.2024.100419. [Google Scholar] [CrossRef]
250. Vats T, Kumar S, Singh SK, Madan U, Preet M, Arya V, et al. Navigating the landscape: safeguarding privacy and security in the era of ambient intelligence within healthcare settings. Cyber Secur Appl. 2023;2:100046. doi:10.1016/j.csa.2024.100046. [Google Scholar] [CrossRef]
251. Kim JW, Moon S-M, Kang S-U, Jang B. Effective privacy-preserving collection of health data from a user’s wearable device. Appl Sci. 2020;10(18):6396. doi:10.3390/app10186396. [Google Scholar] [CrossRef]
252. Thomas L, Gondal I, Oseni T, Firmin S. A framework for data privacy and security accountability in data breach communications. Comput Secur. 2022;116:102657. doi:10.1016/j.cose.2022.102657. [Google Scholar] [CrossRef]
253. Tran A, Luong T, Karnjana J, Huynh V. An efficient approach for privacy-preserving decentralized deep learning models based on secure multi-party computation. Neurocomputing. 2021;422:245–62. doi:10.1016/j.neucom.2020.10.014. [Google Scholar] [CrossRef]
254. Chai H, Huang Y, Xu L, Song X, He M, Wang Q. A decentralized federated learning-based cancer survival prediction method with privacy protection. Heliyon. 2024;10(11):e31873. doi:10.1016/j.heliyon.2024.e31873. [Google Scholar] [PubMed] [CrossRef]
Cite This Article
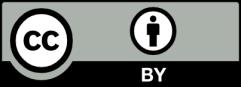
This work is licensed under a Creative Commons Attribution 4.0 International License , which permits unrestricted use, distribution, and reproduction in any medium, provided the original work is properly cited.