Open Access
ARTICLE
Landslide Susceptibility Mapping Using RBFN-Based Ensemble Machine Learning Models
1 Faculty of Civil Engineering, University of Transport Technology, Thanh Xuan, Ha Noi, 100000, Vietnam
2 Faculty of Hydraulic Engineering, Hanoi University of Civil Engineering, Ha Noi, 100000, Vietnam
3 DDG (R) Geological Survey of India, Gandhinagar, 382010, India
4 Research Institute of the University of Bucharest, Bucharest, 050663, Romania
5 National Institute of Hydrology and Water Management, Bucharest, 013686, Romania
6 Department of Civil Engineering, Transilvania University of Brasov, Brasov, 500152, Romania
7 Danube Delta National Institute for Research and Development, Tulcea, 820112, Romania
8 Marwadi University Research Center (MURC), Marwadi University, Rajkot, Gujarat, Bharat, 360003, India
9 Department of Civil Engineering, Faculty of Engineering & Technology, Marwadi University, Rajkot, Gujarat, Bharat, 360003, India
* Corresponding Author: Nguyen Viet Tiep. Email:
(This article belongs to the Special Issue: Computational Intelligent Systems for Solving Complex Engineering Problems: Principles and Applications-II)
Computer Modeling in Engineering & Sciences 2025, 142(1), 467-500. https://doi.org/10.32604/cmes.2024.056576
Received 25 July 2024; Accepted 30 September 2024; Issue published 17 December 2024
Abstract
This study was aimed to prepare landslide susceptibility maps for the Pithoragarh district in Uttarakhand, India, using advanced ensemble models that combined Radial Basis Function Networks (RBFN) with three ensemble learning techniques: DAGGING (DG), MULTIBOOST (MB), and ADABOOST (AB). This combination resulted in three distinct ensemble models: DG-RBFN, MB-RBFN, and AB-RBFN. Additionally, a traditional weighted method, Information Value (IV), and a benchmark machine learning (ML) model, Multilayer Perceptron Neural Network (MLP), were employed for comparison and validation. The models were developed using ten landslide conditioning factors, which included slope, aspect, elevation, curvature, land cover, geomorphology, overburden depth, lithology, distance to rivers and distance to roads. These factors were instrumental in predicting the output variable, which was the probability of landslide occurrence. Statistical analysis of the models’ performance indicated that the DG-RBFN model, with an Area Under ROC Curve (AUC) of 0.931, outperformed the other models. The AB-RBFN model achieved an AUC of 0.929, the MB-RBFN model had an AUC of 0.913, and the MLP model recorded an AUC of 0.926. These results suggest that the advanced ensemble ML model DG-RBFN was more accurate than traditional statistical model, single MLP model, and other ensemble models in preparing trustworthy landslide susceptibility maps, thereby enhancing land use planning and decision-making.Keywords
Cite This Article
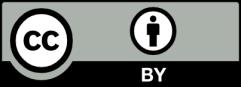
This work is licensed under a Creative Commons Attribution 4.0 International License , which permits unrestricted use, distribution, and reproduction in any medium, provided the original work is properly cited.