Open Access
REVIEW
A Comprehensive Survey on Federated Learning Applications in Computational Mental Healthcare
1 Institute of Informatics and Communication, University of Delhi, Delhi, 110021, India
2 Maharaja Agrasen College, University of Delhi, Delhi, 110096, India
3 Computer Engineering, Ronin Institute, Montclair, NJ 07043, USA
4 Department of Research and Innovation, Insights2Techinfo, Jaipur, 302001, India
5 University Centre for Research and Development (UCRD), Chandigarh University, Chandigarh, 140413, India
6 Management Department, College of Business Administration, Princess Nourah bint Abdulrahman University, Riyadh, 11671, Saudi Arabia
7 CCRI & Department of Computer Science and Information Engineering, Asia University, Taichung, 413, Taiwan
8 Symbiosis Centre for Information Technology (SCIT), Symbiosis International University, Pune, 411057, India
9 Center for Interdisciplinary Research, University of Petroleum and Energy Studies (UPES), Dehradun, 248007, India
* Corresponding Author: Brij B. Gupta. Email:
Computer Modeling in Engineering & Sciences 2025, 142(1), 49-90. https://doi.org/10.32604/cmes.2024.056500
Received 24 July 2024; Accepted 17 October 2024; Issue published 17 December 2024
Abstract
Mental health is a significant issue worldwide, and the utilization of technology to assist mental health has seen a growing trend. This aims to alleviate the workload on healthcare professionals and aid individuals. Numerous applications have been developed to support the challenges in intelligent healthcare systems. However, because mental health data is sensitive, privacy concerns have emerged. Federated learning has gotten some attention. This research reviews the studies on federated learning and mental health related to solving the issue of intelligent healthcare systems. It explores various dimensions of federated learning in mental health, such as datasets (their types and sources), applications categorized based on mental health symptoms, federated mental health frameworks, federated machine learning, federated deep learning, and the benefits of federated learning in mental health applications. This research conducts surveys to evaluate the current state of mental health applications, mainly focusing on the role of Federated Learning (FL) and related privacy and data security concerns. The survey provides valuable insights into how these applications are emerging and evolving, specifically emphasizing FL’s impact.Keywords
Cite This Article
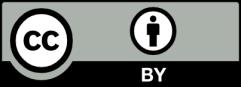
This work is licensed under a Creative Commons Attribution 4.0 International License , which permits unrestricted use, distribution, and reproduction in any medium, provided the original work is properly cited.