Open Access
ARTICLE
Three-Stage Transfer Learning with AlexNet50 for MRI Image Multi-Class Classification with Optimal Learning Rate
1 School of Computing, Sastra Deemed to be University, Thanjavur, 613401, India
2 Department of Computational Intelligence, SRM Institute of Science and Technology, Kattankulathur, 603203, India
3 Department of AI and Software Engineering, Kangwon National University, Samcheok, 25913, Republic of Korea
4 Department of Electronics, Information and Communication Engineering, Kangwon National University, Samcheok, 25913, Republic of Korea
* Corresponding Author: Woong Cho. Email:
Computer Modeling in Engineering & Sciences 2025, 142(1), 155-183. https://doi.org/10.32604/cmes.2024.056129
Received 15 July 2024; Accepted 11 October 2024; Issue published 17 December 2024
Abstract
In radiology, magnetic resonance imaging (MRI) is an essential diagnostic tool that provides detailed images of a patient’s anatomical and physiological structures. MRI is particularly effective for detecting soft tissue anomalies. Traditionally, radiologists manually interpret these images, which can be labor-intensive and time-consuming due to the vast amount of data. To address this challenge, machine learning, and deep learning approaches can be utilized to improve the accuracy and efficiency of anomaly detection in MRI scans. This manuscript presents the use of the Deep AlexNet50 model for MRI classification with discriminative learning methods. There are three stages for learning; in the first stage, the whole dataset is used to learn the features. In the second stage, some layers of AlexNet50 are frozen with an augmented dataset, and in the third stage, AlexNet50 with an augmented dataset with the augmented dataset. This method used three publicly available MRI classification datasets: Harvard whole brain atlas (HWBA-dataset), the School of Biomedical Engineering of Southern Medical University (SMU-dataset), and The National Institute of Neuroscience and Hospitals brain MRI dataset (NINS-dataset) for analysis. Various hyperparameter optimizers like Adam, stochastic gradient descent (SGD), Root mean square propagation (RMS prop), Adamax, and AdamW have been used to compare the performance of the learning process. HWBA-dataset registers maximum classification performance. We evaluated the performance of the proposed classification model using several quantitative metrics, achieving an average accuracy of 98%.Keywords
Cite This Article
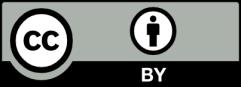
This work is licensed under a Creative Commons Attribution 4.0 International License , which permits unrestricted use, distribution, and reproduction in any medium, provided the original work is properly cited.