Open Access
ARTICLE
Multi-Scale Dilated Convolution Network for SPECT-MPI Cardiovascular Disease Classification with Adaptive Denoising and Attenuation Correction
1 Department of Computational Intelligence, SRM Institute of Science and Technology, Kattankulathur, 603203, Tamil Nadu, India
2 School of Computing, Sastra Deemed to be University, Thanjavur, 613401, Tamil Nadu, India
3 Department of Artificial Intelligence & Software, Kangwon National University, Samcheok, 25913, Republic of Korea
4 Department of Information Convergence System, Graduate School of Smart Convergence, Kwangwoon University, Seoul, 01897, Republic of Korea
* Corresponding Author: Bhanu Shrestha. Email:
Computer Modeling in Engineering & Sciences 2025, 142(1), 299-327. https://doi.org/10.32604/cmes.2024.055599
Received 02 July 2024; Accepted 06 November 2024; Issue published 17 December 2024
Abstract
Myocardial perfusion imaging (MPI), which uses single-photon emission computed tomography (SPECT), is a well-known estimating tool for medical diagnosis, employing the classification of images to show situations in coronary artery disease (CAD). The automatic classification of SPECT images for different techniques has achieved near-optimal accuracy when using convolutional neural networks (CNNs). This paper uses a SPECT classification framework with three steps: 1) Image denoising, 2) Attenuation correction, and 3) Image classification. Image denoising is done by a U-Net architecture that ensures effective image denoising. Attenuation correction is implemented by a convolution neural network model that can remove the attenuation that affects the feature extraction process of classification. Finally, a novel multi-scale diluted convolution (MSDC) network is proposed. It merges the features extracted in different scales and makes the model learn the features more efficiently. Three scales of filters with size are used to extract features. All three steps are compared with state-of-the-art methods. The proposed denoising architecture ensures a high-quality image with the highest peak signal-to-noise ratio (PSNR) value of 39.7. The proposed classification method is compared with the five different CNN models, and the proposed method ensures better classification with an accuracy of 96%, precision of 87%, sensitivity of 87%, specificity of 89%, and F1-score of 87%. To demonstrate the importance of preprocessing, the classification model was analyzed without denoising and attenuation correction.Keywords
Cite This Article
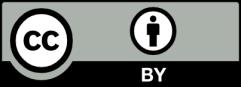
This work is licensed under a Creative Commons Attribution 4.0 International License , which permits unrestricted use, distribution, and reproduction in any medium, provided the original work is properly cited.