Open Access
ARTICLE
Machine Learning Techniques in Predicting Hot Deformation Behavior of Metallic Materials
1 Department of Metallurgical Technologies, Faculty of Materials Science and Technology, VSB–Technical University of Ostrava, Ostrava, 70800, Czech Republic
2 FZU—Institute of Physics of the Czech Academy of Sciences, Prague, 18200, Czech Republic
* Corresponding Authors: Petr Opěla. Email: ; Josef Walek. Email:
(This article belongs to the Special Issue: Computer Aided Simulation in Metallurgy and Material Engineering)
Computer Modeling in Engineering & Sciences 2025, 142(1), 713-732. https://doi.org/10.32604/cmes.2024.055219
Received 20 June 2024; Accepted 23 September 2024; Issue published 17 December 2024
Abstract
In engineering practice, it is often necessary to determine functional relationships between dependent and independent variables. These relationships can be highly nonlinear, and classical regression approaches cannot always provide sufficiently reliable solutions. Nevertheless, Machine Learning (ML) techniques, which offer advanced regression tools to address complicated engineering issues, have been developed and widely explored. This study investigates the selected ML techniques to evaluate their suitability for application in the hot deformation behavior of metallic materials. The ML-based regression methods of Artificial Neural Networks (ANNs), Support Vector Machine (SVM), Decision Tree Regression (DTR), and Gaussian Process Regression (GPR) are applied to mathematically describe hot flow stress curve datasets acquired experimentally for a medium-carbon steel. Although the GPR method has not been used for such a regression task before, the results showed that its performance is the most favorable and practically unrivaled; neither the ANN method nor the other studied ML techniques provide such precise results of the solved regression analysis.Keywords
Cite This Article
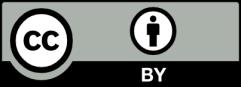
This work is licensed under a Creative Commons Attribution 4.0 International License , which permits unrestricted use, distribution, and reproduction in any medium, provided the original work is properly cited.