Open Access
ARTICLE
Prediction of Shear Bond Strength of Asphalt Concrete Pavement Using Machine Learning Models and Grid Search Optimization Technique
1 Civil Engineering Department, University of Transport Technology, 54 Trieu Khuc, Thanh Xuan, Hanoi, 100000, Vietnam
2 DDG (R) Geological Survey of India, Gandhinagar, 382010, India
* Corresponding Authors: Quynh-Anh Thi Bui. Email: ; Binh Thai Pham. Email:
(This article belongs to the Special Issue: Soft Computing Applications of Civil Engineering including AI-based Optimization and Prediction)
Computer Modeling in Engineering & Sciences 2025, 142(1), 691-712. https://doi.org/10.32604/cmes.2024.054766
Received 06 June 2024; Accepted 17 October 2024; Issue published 17 December 2024
Abstract
Determination of Shear Bond strength (SBS) at interlayer of double-layer asphalt concrete is crucial in flexible pavement structures. The study used three Machine Learning (ML) models, including K-Nearest Neighbors (KNN), Extra Trees (ET), and Light Gradient Boosting Machine (LGBM), to predict SBS based on easily determinable input parameters. Also, the Grid Search technique was employed for hyper-parameter tuning of the ML models, and cross-validation and learning curve analysis were used for training the models. The models were built on a database of 240 experimental results and three input variables: temperature, normal pressure, and tack coat rate. Model validation was performed using three statistical criteria: the coefficient of determination (R2), the Root Mean Square Error (RMSE), and the mean absolute error (MAE). Additionally, SHAP analysis was also used to validate the importance of the input variables in the prediction of the SBS. Results show that these models accurately predict SBS, with LGBM providing outstanding performance. SHAP (Shapley Additive explanation) analysis for LGBM indicates that temperature is the most influential factor on SBS. Consequently, the proposed ML models can quickly and accurately predict SBS between two layers of asphalt concrete, serving practical applications in flexible pavement structure design.Keywords
Cite This Article
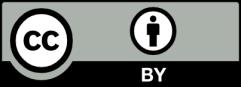
This work is licensed under a Creative Commons Attribution 4.0 International License , which permits unrestricted use, distribution, and reproduction in any medium, provided the original work is properly cited.