Open Access
ARTICLE
Augmenting Internet of Medical Things Security: Deep Ensemble Integration and Methodological Fusion
1 Faculty of Informatics and Management, Center for Basic and Applied Research, University of Hradec Kralove, Hradec Kralove, 50003, Czech Republic
2 Department of Computer Science, College of Computer and Information Sciences, Jouf University, Al Jouf, 72388, Saudi Arabia
3 Department of Computer Engineering & Networks, College of Computer and Information Sciences, Jouf University, Al Jouf, 72388, Saudi Arabia
4 Cybersecurity Center, Prince Mohammad Bin Fahd University, Al Jawharah, Khobar, Dhahran, 34754, Saudi Arabia
* Corresponding Author: Amjad Alsirhani. Email:
Computer Modeling in Engineering & Sciences 2024, 141(3), 2185-2223. https://doi.org/10.32604/cmes.2024.056308
Received 19 July 2024; Accepted 08 October 2024; Issue published 31 October 2024
Abstract
When it comes to smart healthcare business systems, network-based intrusion detection systems are crucial for protecting the system and its networks from malicious network assaults. To protect IoMT devices and networks in healthcare and medical settings, our proposed model serves as a powerful tool for monitoring IoMT networks. This study presents a robust methodology for intrusion detection in Internet of Medical Things (IoMT) environments, integrating data augmentation, feature selection, and ensemble learning to effectively handle IoMT data complexity. Following rigorous preprocessing, including feature extraction, correlation removal, and Recursive Feature Elimination (RFE), selected features are standardized and reshaped for deep learning models. Augmentation using the BAT algorithm enhances dataset variability. Three deep learning models, Transformer-based neural networks, self-attention Deep Convolutional Neural Networks (DCNNs), and Long Short-Term Memory (LSTM) networks, are trained to capture diverse data aspects. Their predictions form a meta-feature set for a subsequent meta-learner, which combines model strengths. Conventional classifiers validate meta-learner features for broad algorithm suitability. This comprehensive method demonstrates high accuracy and robustness in IoMT intrusion detection. Evaluations were conducted using two datasets: the publicly available WUSTL-EHMS-2020 dataset, which contains two distinct categories, and the CICIoMT2024 dataset, encompassing sixteen categories. Experimental results showcase the method’s exceptional performance, achieving optimal scores of 100% on the WUSTL-EHMS-2020 dataset and 99% on the CICIoMT2024.Keywords
Cite This Article
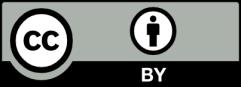
This work is licensed under a Creative Commons Attribution 4.0 International License , which permits unrestricted use, distribution, and reproduction in any medium, provided the original work is properly cited.