Open Access
ARTICLE
Transfer Learning Empowered Skin Diseases Detection in Children
1 Department of Computer Science, College of Computer Science and Information Technology, Imam Abdulrahman Bin Faisal University, P.O. Box 1982, Dammam, 31441, Saudi Arabia
2 Department of Computer Information Systems, College of Computer Science and Information Technology, Imam Abdulrahman Bin Faisal University, P.O. Box 1982, Dammam, 31441, Saudi Arabia
3 Department of Computer Engineering, College of Computer Science and Information Technology, Imam Abdulrahman Bin Faisal University, P.O. Box 1982, Dammam, 31441, Saudi Arabia
4 ICS Department, King Fahd University of Petroleum and Minerals, Dhahran, 31261, Saudi Arabia
* Corresponding Author: Atta Rahman. Email:
(This article belongs to the Special Issue: Intelligent Medical Decision Support Systems: Methods and Applications)
Computer Modeling in Engineering & Sciences 2024, 141(3), 2609-2623. https://doi.org/10.32604/cmes.2024.055303
Received 22 June 2024; Accepted 12 September 2024; Issue published 31 October 2024
Abstract
Human beings are often affected by a wide range of skin diseases, which can be attributed to genetic factors and environmental influences, such as exposure to sunshine with ultraviolet (UV) rays. If left untreated, these diseases can have severe consequences and spread, especially among children. Early detection is crucial to prevent their spread and improve a patient’s chances of recovery. Dermatology, the branch of medicine dealing with skin diseases, faces challenges in accurately diagnosing these conditions due to the difficulty in identifying and distinguishing between different diseases based on their appearance, type of skin, and others. This study presents a method for detecting skin diseases using Deep Learning (DL), focusing on the most common diseases affecting children in Saudi Arabia due to the high UV value in most of the year, especially in the summer. The method utilizes various Convolutional Neural Network (CNN) architectures to classify skin conditions such as eczema, psoriasis, and ringworm. The proposed method demonstrates high accuracy rates of 99.99% and 97% using famous and effective transfer learning models MobileNet and DenseNet121, respectively. This illustrates the potential of DL in automating the detection of skin diseases and offers a promising approach for early diagnosis and treatment.Keywords
Cite This Article
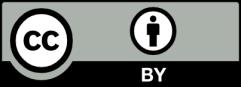
This work is licensed under a Creative Commons Attribution 4.0 International License , which permits unrestricted use, distribution, and reproduction in any medium, provided the original work is properly cited.