Open Access
ARTICLE
Enhancing Septic Shock Detection through Interpretable Machine Learning
1 Department of Information and Computer Science, King Fahd University of Petroleum and Minerals, Dhahran, 31261, Saudi Arabia
2 Department of Electrical and Computer Engineering, North South University, Dhaka, 1229, Bangladesh
3 Department of Computer Science, Taif University, Taif, 21974, Saudi Arabia
4 College of Applied Arts and Technology, Seneca Polytechnic, Toronto, M2J 2X5, Canada
5 Department of Control & Instrumentation Engineering, King Fahd University of Petroleum and Minerals, Dhahran, 31261, Saudi Arabia
* Corresponding Author: Md Mahfuzur Rahman. Email:
(This article belongs to the Special Issue: Exploring the Impact of Artificial Intelligence on Healthcare: Insights into Data Management, Integration, and Ethical Considerations)
Computer Modeling in Engineering & Sciences 2024, 141(3), 2501-2525. https://doi.org/10.32604/cmes.2024.055065
Received 15 June 2024; Accepted 09 September 2024; Issue published 31 October 2024
Abstract
This article presents an innovative approach that leverages interpretable machine learning models and cloud computing to accelerate the detection of septic shock by analyzing electronic health data. Unlike traditional methods, which often lack transparency in decision-making, our approach focuses on early detection, offering a proactive strategy to mitigate the risks of sepsis. By integrating advanced machine learning algorithms with interpretability techniques, our method not only provides accurate predictions but also offers clear insights into the factors influencing the model’s decisions. Moreover, we introduce a preference-based matching algorithm to evaluate disease severity, enabling timely interventions guided by the analysis outcomes. This innovative integration significantly enhances the effectiveness of our approach. We leverage a clinical health dataset comprising 1,552,210 Electronic Health Records (EHR) to train our interpretable machine learning models within a cloud computing framework. Through techniques like feature importance analysis and model-agnostic interpretability tools, we aim to clarify the crucial indicators contributing to septic shock prediction. This transparency not only assists healthcare professionals in comprehending the model’s predictions but also facilitates the integration of our system into existing clinical workflows. We validate the effectiveness of our interpretable models using the same dataset, achieving an impressive accuracy rate exceeding 98% through the application of oversampling techniques. The findings of this study hold significant implications for the advancement of more effective and transparent diagnostic tools in the critical domain of sepsis management.Keywords
Cite This Article
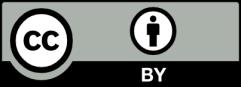
This work is licensed under a Creative Commons Attribution 4.0 International License , which permits unrestricted use, distribution, and reproduction in any medium, provided the original work is properly cited.