Open Access
ARTICLE
Optimizing Bearing Fault Detection: CNN-LSTM with Attentive TabNet for Electric Motor Systems
1 Nautical Science Department, Faculty of Maritime, King Abdulaziz University, Jeddah, 22230, Saudi Arabia
2 Department of Computer Science, COMSATS University Islamabad, Sahiwal Campus, Sahiwal, 57000, Pakistan
3 Marine Engineering Department, Faculty of Maritime, King Abdulaziz University, Jeddah, 22230, Saudi Arabia
4 Artificial Intelligence and Sensing Technologies (AIST) Research Center, University of Tabuk, Tabuk, 71491, Saudi Arabia
5 Electrical Engineering Department, College of Engineering, Najran University, Najran, 61441, Saudi Arabia
* Corresponding Author: Ahmad Shaf. Email:
(This article belongs to the Special Issue: Computational Intelligent Systems for Solving Complex Engineering Problems: Principles and Applications-II)
Computer Modeling in Engineering & Sciences 2024, 141(3), 2399-2420. https://doi.org/10.32604/cmes.2024.054257
Received 23 May 2024; Accepted 31 July 2024; Issue published 31 October 2024
Abstract
Electric motor-driven systems are core components across industries, yet they’re susceptible to bearing faults. Manual fault diagnosis poses safety risks and economic instability, necessitating an automated approach. This study proposes FTCNNLSTM (Fine-Tuned TabNet Convolutional Neural Network Long Short-Term Memory), an algorithm combining Convolutional Neural Networks, Long Short-Term Memory Networks, and Attentive Interpretable Tabular Learning. The model preprocesses the CWRU (Case Western Reserve University) bearing dataset using segmentation, normalization, feature scaling, and label encoding. Its architecture comprises multiple 1D Convolutional layers, batch normalization, max-pooling, and LSTM blocks with dropout, followed by batch normalization, dense layers, and appropriate activation and loss functions. Fine-tuning techniques prevent overfitting. Evaluations were conducted on 10 fault classes from the CWRU dataset. FTCNNLSTM was benchmarked against four approaches: CNN, LSTM, CNN-LSTM with random forest, and CNN-LSTM with gradient boosting, all using 460 instances. The FTCNNLSTM model, augmented with TabNet, achieved 96% accuracy, outperforming other methods. This establishes it as a reliable and effective approach for automating bearing fault detection in electric motor-driven systems.Keywords
Cite This Article
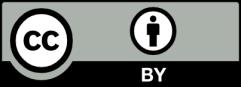
This work is licensed under a Creative Commons Attribution 4.0 International License , which permits unrestricted use, distribution, and reproduction in any medium, provided the original work is properly cited.