Open Access
ARTICLE
Tree-Based Solution Frameworks for Predicting Tunnel Boring Machine Performance Using Rock Mass and Material Properties
1 School of Civil and Environmental Engineering, University of Technology Sydney (UTS), Ultimo, NSW 2007, Australia
2 School of Resources and Safety Engineering, Central South University, Changsha, 410083, China
3 Faculty of Earth Sciences Engineering, Arak University of Technology, Arak, 38181-46763, Iran
4 Department of Mechanical Engineering, New Mexico Institute of Mining and Technology, Socorro, NM 87801, USA
* Corresponding Author: Danial Jahed Armaghani. Email:
(This article belongs to the Special Issue: Computational Intelligent Systems for Solving Complex Engineering Problems: Principles and Applications-II)
Computer Modeling in Engineering & Sciences 2024, 141(3), 2421-2451. https://doi.org/10.32604/cmes.2024.052210
Received 26 March 2024; Accepted 18 September 2024; Issue published 31 October 2024
Abstract
Tunnel Boring Machines (TBMs) are vital for tunnel and underground construction due to their high safety and efficiency. Accurately predicting TBM operational parameters based on the surrounding environment is crucial for planning schedules and managing costs. This study investigates the effectiveness of tree-based machine learning models, including Random Forest, Extremely Randomized Trees, Adaptive Boosting Machine, Gradient Boosting Machine, Extreme Gradient Boosting Machine (XGBoost), Light Gradient Boosting Machine, and CatBoost, in predicting the Penetration Rate (PR) of TBMs by considering rock mass and material characteristics. These techniques are able to provide a good relationship between input(s) and output parameters; hence, obtaining a high level of accuracy. To do that, a comprehensive database comprising various rock mass and material parameters, including Rock Mass Rating, Brazilian Tensile Strength, and Weathering Zone, was utilized for model development. The practical application of these models was assessed with a new dataset representing diverse rock mass and material properties. To evaluate model performance, ranking systems and Taylor diagrams were employed. CatBoost emerged as the most accurate model during training and testing, with R2 scores of 0.927 and 0.861, respectively. However, during validation, XGBoost demonstrated superior performance with an R2 of 0.713. Despite these variations, all tree-based models showed promising accuracy in predicting TBM performance, providing valuable insights for similar projects in the future.Keywords
Cite This Article
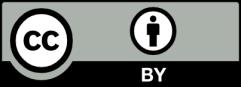
This work is licensed under a Creative Commons Attribution 4.0 International License , which permits unrestricted use, distribution, and reproduction in any medium, provided the original work is properly cited.