Open Access
ARTICLE
Faster AMEDA—A Hybrid Mesoscale Eddy Detection Algorithm
1 Organization Department, Nanjing University of Information Science and Technology, Nanjing, 210044, China
2 School of Computer Science, Nanjing University of Information Science and Technology, Nanjing, 210044, China
3 School of Teacher Education, Nanjing University of Information Science and Technology, Nanjing, 210044, China
4 School of Electronics & Information Engineering, Nanjing University of Information Science and Technology, Nanjing, 210044, China
5 School of Software, Nanjing University of Information Science and Technology, Nanjing, 210044, China
* Corresponding Author: Biao Song. Email:
(This article belongs to the Special Issue: Lightweight Methods and Resource-efficient Computing Solutions)
Computer Modeling in Engineering & Sciences 2024, 141(2), 1827-1846. https://doi.org/10.32604/cmes.2024.054298
Received 24 May 2024; Accepted 23 July 2024; Issue published 27 September 2024
Abstract
Identification of ocean eddies from a large amount of ocean data provided by satellite measurements and numerical simulations is crucial, while the academia has invented many traditional physical methods with accurate detection capability, but their detection computational efficiency is low. In recent years, with the increasing application of deep learning in ocean feature detection, many deep learning-based eddy detection models have been developed for more effective eddy detection from ocean data. But it is difficult for them to precisely fit some physical features implicit in traditional methods, leading to inaccurate identification of ocean eddies. In this study, to address the low efficiency of traditional physical methods and the low detection accuracy of deep learning models, we propose a solution that combines the target detection model Faster Region with CNN feature (Faster R-CNN) with the traditional dynamic algorithm Angular Momentum Eddy Detection and Tracking Algorithm (AMEDA). We use Faster R-CNN to detect and generate bounding boxes for eddies, allowing AMEDA to detect the eddy center within these bounding boxes, thus reducing the complexity of center detection. To demonstrate the detection efficiency and accuracy of this model, this paper compares the experimental results with AMEDA and the deep learning-based eddy detection method eddyNet. The results show that the eddy detection results of this paper are more accurate than eddyNet and have higher execution efficiency than AMEDA.Keywords
Cite This Article
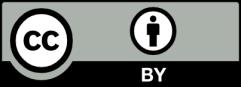
This work is licensed under a Creative Commons Attribution 4.0 International License , which permits unrestricted use, distribution, and reproduction in any medium, provided the original work is properly cited.