Open Access
EDITORIAL
Introduction to the Special Issue on Computer-Aided Uncertainty Modeling and Reliability Evaluation for Complex Engineering Structures
1 School of Mechanical and Electrical Engineering, University of Electronic Science and Technology of China, Chengdu, 611731, China
2INEGI, Faculty of Engineering, University of Porto, Porto, 4200-465, Portugal
3 School of Civil Engineering, Hefei University of Technology, Hefei, 230009, China
* Corresponding Author: Debiao Meng. Email:
(This article belongs to the Special Issue: Computer-Aided Uncertainty Modeling and Reliability Evaluation for Complex Engineering Structures)
Computer Modeling in Engineering & Sciences 2024, 141(1), 1-5. https://doi.org/10.32604/cmes.2024.056319
Received 19 July 2024; Accepted 24 July 2024; Issue published 20 August 2024
Abstract
This article has no abstract.With the advancement of science and technology, complex engineering structures are widely used in extreme environments [1]. In equipment service, many uncertainty factors significantly affect safety and reliability [2–5]. Therefore, ensuring high reliability of structures has become an important research direction in engineering design. At the same time, the importance of equipment health management of complex engineering structures is becoming increasingly prominent [6–8]. Computer-aided uncertainty modeling and reliability assessment have become key tools, and finite element simulation and algorithmic innovation play a key role in the reliability analysis of complex equipment [9,10]. These techniques can accurately simulate stress and damage accumulation under various operating environments, providing engineers with important decision support and optimization solutions.
Reliability analysis is critical in complex engineering structures or specific engineering situations that often involve multiple interactions and complex system relationships, where the failure of any one component can lead to the collapse or loss of functionality of the entire system [11]. Therefore, through in-depth reliability analysis, engineers are able to identify potential risk points and critical failure modes so that preventive measures and design improvements can be made to ensure safe and reliable system operation despite extreme conditions.
In this special issue, several papers about reliability analysis in complex engineering structures or complex working conditions have been accepted. In order to solve the planning problem of UAV swarm combat tests, based on the multi-phase characteristics of UAV swarm missions consisting of three phases: launch, flight and combat, Jiang et al. [12] proposed a methodology to find test scenarios that can maximize the reliability of the mission. Li et al. [13] proposed a random forest model for improving the computational efficiency and accuracy of reliability design in order to solve the problem of poor computational efficiency and accuracy arising from multiple occurrences of reliability calculations and implicit high nonlinear limit state function calls in the fatigue reliability-based structural design optimization of aero-engines. Zhang et al. [14] used a Kriging element model to establish a mathematical relationship between design parameters and contact stresses. A hybrid algorithm that merges a genetic algorithm and a quantum particle swarm optimization algorithm was also used to tune the parameters of the Kriging metamodel to determine the relationship between the computed contact stresses and the studied parameters for a circular-geared pitch cylindrical gear. Besides, some scholars have innovated and summarized the reliability analysis methods. Wang et al. [15] then proposed an efficient decoupled Reliability-Based Design Optimization (RBDO) method combining High-Dimensional Model Representation (HDMR) and Weight Point Estimation Method (WPEM), Xia et al. [16] proposed a reliability analysis method based on T-S Fault Tree Analysis (T-SFTA) and Hyper-Elliptic Bayesian Networks (HE-BN) when dealing with the problem of analyzing the reliability of high systems in the military, aerospace, and railroad fields. Xu et al. [17] introduced the theory of evidence to assess the level of performance conditions in each situation, and the method is able to calculate the weights of each performance condition and modify its multipliers to make the estimation more reasonable. Meng et al. [18] provided a summary review of the methods of saddle-point approximation used in reliability analysis and reliability-based design optimization problems, describing the saddle-point approximation strategies of each order and application. All of these papers explore different analysis methods and strategies around the researched fields to enhance the safety and reliability of engineering structures, and there are also summaries of reliability analysis methods in the researched fields for future generations to make innovations based on them. All these results provide valuable technical support for actual engineering practice, as well as important guidance and insights for future complex engineering design and maintenance management.
Relevant research in the field of health management of complex engineering structures, such as condition monitoring, fault diagnosis and health monitoring, can improve the safety and reliability of engineering structures, achieve timely diagnosis and prediction, and reduce downtime and the cost of maintenance. A number of authors in this special issue have also investigated the health management of some equipment with the assistance of computer modeling. Ren et al. [19] used the CatBoost model in the condition monitoring of stirred reactors, and the validation results showed that the detection accuracy of the model was 100%, and the diagnostic accuracy of the fault types was 98%. Kang et al. [20] coupled a conventional Tuned Mass Damper (TMD) with an inertial device to form a passive dynamic vibration absorber as a way to reduce the cost of the tuned mass damper inertia. Ai et al. [21] proposed an anomaly detection method based on a dynamic prediction model modeled with a rolling time window to achieve dynamic prediction. In order to solve the problem of misclassification and lack of performance of target samples due to the use of migration learning alone in the deep learning-based mechanical fault diagnosis method approach, Guo et al. [22] proposed a Deep Discriminative Adversarial Domain Adaptive Neural Network (DDADAN) for bearing fault diagnostic model, which was able to maintain an accuracy of more than 97.53% under a variety of transmission tasks. Wang et al. [23] proposed a hybrid fault diagnosis method for high-speed wire-finishing machine tools based on expert experience and data-driven. Computer-aided health management of complex engineering structures is an indispensable part of modern engineering practice, and it is an important guarantee for the sustainable operation of complex engineering.
In the reliability and safety analysis of modern complex engineering structures, the cost of realistic reliability analysis and health management prediction is usually higher because of the complexity of the structure so that some scholars will assist the relevant analysis through the study of finite element simulation tools and algorithms. There are also many papers in this special issue that have carried out this kind of research on reliability with the assistance of computer tools. Wu et al. [24] have carried out modular and parametric programming of underground works under complex geological conditions by means of finite element simulation to quickly and accurately simulate and analyze the complex underground works. In order to solve the problem that the traditional optimal preload prediction methods are limited by practical conditions and uncertainties, Jiang et al. [25] proposed a multi-sensor fusion method for rolling bearing preload testing based on the improved D-S evidence theory. Du et al. [26] established a time-frequency matrix to argue that the CSI has a low-rank potential and proposed a distributed decomposition algorithm to effectively separate the stable structural information and contextual fluctuations in the CSI matrix. Diao et al. [27] proposed an adaptive H∞ filtering algorithm with combinatorial constraints in order to solve the problem of data fusion of track information, train state and other a priori information in train localization, which is capable of significantly reducing the train localization error and verified the validity of the algorithm. Wang et al. [28] established an intrinsic model for the interface of sandblasted interface and wine-sprayed basalt aggregate interface with no shear connectors for the connection between ultra-high-strength concrete and steel plates. Li et al. [29] proposed a variable gain and finite area design method to solve the problem of rising control power due to the fixed limiting area of the active mass damper in the collision avoidance device, and the comparison of the results revealed that the proposed method has superior travel limiting performance. Su et al. [30] proposed a new Pearson correlation coefficient-based dependence between PSFs in SPAR-H to solve the problem of inconsistency with the real situation in the classical SPAR-H method due to neglecting the dependence between Performance Shaping Factors (PSFs) and proved the effectiveness of the proposed method through a case study. With the help of modern computer tools and software algorithms, we are able to minimize the cost of reliability analysis when facing some complex engineering structures, which not only improves the accuracy and efficiency of engineering design but also provides engineers with effective decision-making support and risk management tools, thus promoting the continuous innovation and development of the engineering field.
This special issue brings together cutting-edge research results on the reliability analysis of complex engineering structures and working conditions in different research fields, including innovative research on theoretical methods and practical engineering applications. From theoretical discussion to practical application, each paper discusses the key role of modern computer tools and algorithm optimization in enhancing the analysis of complex engineering structures or working conditions. The authors of the papers validate the proposed efficient and accurate methods by means of example verification and finite element simulation, providing new technical perspectives and solutions for engineering practice. All in all, this special issue provides profound thinking and practical guidance for academic innovation and practical application in the field of complex engineering and lays a solid foundation for the reliable and sustainable development of complex engineering equipment in the future.
Funding Statement: The authors gratefully acknowledge the fundings from Project ATE: Agenda para a Transição Energética (02/C05-i01.02/2022.PC644914747-00000023), cofinanced by Plano de Recuperação e Resiliência (PRR), República Portuguesa, through NextGeneration EU; the project entitled Giga-Cycle Fatigue Behaviour of Engineering Metallic Alloys (PTDC/EME-EME/7678/2020); National Natural Science Foundation of China (Grant No. 12372195); the Guangdong Basic and Applied Basic Research Foundation (Grant No. 2022A1515240010); China Scholarship Council (No. 202406070043).
Conflicts of Interest: The authors declare that they have no conflicts of interest to report regarding the present study.
References
1. Meng D, Wang H, Yang S, Lv Z, Hu Z, Wang Z. Fault analysis of wind power rolling bearing based on EMD feature extraction. Comput Model Eng Sci. 2022;130(1):543–58. doi:10.32604/cmes.2022.018123. [Google Scholar] [CrossRef]
2. Meng D, Yang S, de Jesus AM, Zhu SP. A novel Kriging-model-assisted reliability-based multidisciplinary design optimization strategy and its application in the offshore wind turbine tower. Renew Energy. 2023;203:407–20. doi:10.1016/j.renene.2022.12.062. [Google Scholar] [CrossRef]
3. Xu Y, Reniers G, Yang M, Yuan S, Chen C. Uncertainties and their treatment in the quantitative risk assessment of domino effects: classification and review. Process Saf Environ Prot. 2023;172:971–85. doi:10.1016/j.psep.2023.02.082. [Google Scholar] [CrossRef]
4. Meng H, Hu M, Kong Z, Niu Y, Liang J, Nie Z, et al. Risk analysis of lithium-ion battery accidents based on physics-informed data-driven Bayesian networks. Reliab Eng Syst Saf. 2024;251:110294. doi:10.1016/j.ress.2024.110294. [Google Scholar] [CrossRef]
5. Van Huynh T, Tangaramvong S, Do B, Gao W. A novel decoupled approach combining invertible cross-entropy method with Gaussian process modeling for reliability-based design and topology optimization. Comput Methods Appl Mech Eng. 2024;427:117006. doi:10.1016/j.cma.2024.117006. [Google Scholar] [CrossRef]
6. Yang S, Meng D, Wang H, Chen Z, Xu B. A comparative study for adaptive surrogate-model-based reliability evaluation method of automobile components. Int J Struct Integr. 2023;14(3):498–519. doi:10.1108/IJSI-03-2023-0020. [Google Scholar] [CrossRef]
7. Meng D, Yang S, De Jesus AM, Fazeres-Ferradosa T, Zhu SP. A novel hybrid adaptive Kriging and water cycle algorithm for reliability-based design and optimization strategy: application in offshore wind turbine monopile. Comput Methods Appl Mech Eng. 2023;412:116083. doi:10.1016/j.cma.2023.116083. [Google Scholar] [CrossRef]
8. Afshari SS, Enayatollahi F, Xu X, Liang X. Machine learning-based methods in structural reliability analysis: a review. Reliab Eng Syst Safe. 2022;219:108223. doi:10.1016/j.ress.2021.108223. [Google Scholar] [CrossRef]
9. Yang S, Meng D, Wang H, Yang C. A novel learning function for adaptive surrogate-model-based reliability evaluation. Philos Trans Royal Soc A. 2024;382(2264):20220395. doi:10.1098/rsta.2022.0395. [Google Scholar] [PubMed] [CrossRef]
10. Zhang W, Gu X, Hong L, Han L, Wang L. Comprehensive review of machine learning in geotechnical reliability analysis: algorithms, applications and further challenges. Appl Soft Comput. 2023;136:110066. doi:10.1016/j.asoc.2023.110066. [Google Scholar] [CrossRef]
11. Yang S, He Z, Chai J, Meng D, Macek W, Branco R, et al. A novel hybrid adaptive framework for support vector machine-based reliability analysis: a comparative study. Structures. 2023;58:105665. doi:10.1016/j.istruc.2023.105665. [Google Scholar] [CrossRef]
12. Jiang P, Qi J. A planning method for operational test of UAV Swarm based on mission reliability. Comput Model Eng Sci. 2024;140(2):1889–918. doi:10.32604/cmes.2024.049813. [Google Scholar] [CrossRef]
13. Li XQ, Song LK. Random forest-based fatigue reliability-based design optimization for aeroengine structures. Comput Model Eng Sci. 2024;140(1):665–84. doi:10.32604/cmes.2024.048445. [Google Scholar] [CrossRef]
14. Zhang Q, Chen Z, Wu Y, Xiang G, Wen G, Zhang X, et al. Contact stress reliability analysis model for cylindrical gear with circular arc tooth trace based on an improved metamodel. Comput Model Eng Sci. 2024;140(1):593–619. doi:10.32604/cmes.2023.046319. [Google Scholar] [CrossRef]
15. Wang X, Chang X, Wang W, Qiao Z, Zhang F. An efficient reliability-based optimization method utilizing high-dimensional model representation and weight-point estimation method. Comput Model Eng Sci. 2024;139(2):1775–96. doi:10.32604/cmes.2023.043913. [Google Scholar] [CrossRef]
16. Xia Q, Li Y, Zhang D, Wang Y. System reliability analysis method based on TS FTA and HE-BN. Comput Model Eng Sci. 2024;138(2):1769–94. doi:10.32604/cmes.2023.030724. [Google Scholar] [CrossRef]
17. Xu Z, Shang S, Pu Y, Su X, Qian H, Pan X. An improved CREAM model based on DS Evidence Theory and DEMATEL. Comput Model Eng Sci. 2024;138(3):2597–617. doi:10.32604/cmes.2023.031247. [Google Scholar] [CrossRef]
18. Meng D, Guo Y, Xu Y, Yang S, Guo Y, Pan L, et al. Saddlepoint approximation method in reliability analysis: a review. Comput Model Eng Sci. 2024;139(3):2329–59. doi:10.32604/cmes.2024.047507. [Google Scholar] [CrossRef]
19. Ren X, Yu H, Chen X, Tang Y, Wang G, Du X. Application of the cat boost model for stirred reactor state monitoring based on vibration signals. Comput Model Eng Sci. 2024;140(1):647–63. doi:10.32604/cmes.2024.048782. [Google Scholar] [CrossRef]
20. Kang X, Huang Q, Wu Z, Tang J, Jiang X, Lei S. A review of the tuned mass damper inerter (TMDI) in energy harvesting and vibration control: designs, analysis and applications. Comput Model Eng Sci. 2024;139(3):2361–98. doi:10.32604/cmes.2023.043936. [Google Scholar] [CrossRef]
21. Ai Q, Tian H, Wang H, Lang Q, Huang X, Jiang X, et al. Comparative analysis of ARIMA and LSTM model-based anomaly detection for unannotated structural health monitoring data in an immersed tunnel. Comput Model Eng Sci. 2024;139(2):1797–827. doi:10.32604/cmes.2023.045251. [Google Scholar] [CrossRef]
22. Guo J, Chen K, Liu J, Ma Y, Wu J, Wu Y, et al. Bearing fault diagnosis based on deep discriminative adversarial domain adaptation neural networks. Comput Model Eng Sci. 2024;138(3):2619–40. doi:10.32604/cmes.2023.031360. [Google Scholar] [CrossRef]
23. Wang C, Tang N, Zhang Q, Gao L, Yin H, Peng H. Expert experience and data-driven based hybrid fault diagnosis for high-speed wire rod finishing mills. Comput Model Eng Sci. 2024;138(2):1827–47. doi:10.32604/cmes.2023.030970. [Google Scholar] [CrossRef]
24. Wu J, Zhuo L, Pei J, Li Y, Xie H, Wu J, et al. Modularized and parametric modeling technology for finite element simulations of underground engineering under complicated geological conditions. Comput Model Eng Sci. 2024;140(1):621–45. doi:10.32604/cmes.2024.046398. [Google Scholar] [CrossRef]
25. Jiang K, Han C, Chang Y. Research on optimal preload method of controllable rolling bearing based on multisensor fusion. Comput Model Eng Sci. 2024;139(3):3329–52. doi:10.32604/cmes.2024.046729. [Google Scholar] [CrossRef]
26. Du L, Shang S, Zhang L, Li C, Yang J, Tian X. Multidomain correlation-based multidimensional CSI tensor generation for device-free Wi-Fi sensing. Comput Model Eng Sci. 2024;138(2):1749–67. doi:10.32604/cmes.2023.030144. [Google Scholar] [CrossRef]
27. Diao X, Wang P, Li W, Chu X, Wang Y. Adaptive H∞ filtering algorithm for train positioning based on prior combination constraints. Comput Model Eng Sci. 2024;138(2):1795–812. doi:10.32604/cmes.2023.030008. [Google Scholar] [CrossRef]
28. Wang Z, Zhang B, Wang H, Ai Q, Huang X. Constitutive behavior of the interface between UHPC and steel plate without shear connector: from experimental to numerical study. Comput Model Eng Sci. 2024;140(2):1863–88. doi:10.32604/cmes.2024.048217. [Google Scholar] [CrossRef]
29. Li ZH, Wu QG, Teng J, Chen CJ. A Stroke-limitation AMD control system with variable gain and limited area for high-rise buildings. Comput Model Eng Sci. 2024;138(1):865–84. doi:10.32604/cmes.2023.029927. [Google Scholar] [CrossRef]
30. Su X, Shang S, Xu Z, Qian H, Pan X. Assessment of dependent performance shaping factors in SPAR-H based on pearson correlation coefficient. Comput Model Eng Sci. 2024;138(2):1813–26. doi:10.32604/cmes.2023.030957. [Google Scholar] [CrossRef]
Cite This Article
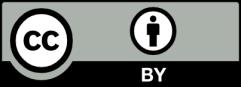
This work is licensed under a Creative Commons Attribution 4.0 International License , which permits unrestricted use, distribution, and reproduction in any medium, provided the original work is properly cited.