Open Access
ARTICLE
Analysis of Progressively Type-II Inverted Generalized Gamma Censored Data and Its Engineering Application
1 Department of Mathematical Sciences, College of Science, Princess Nourah bint Abdulrahman University, P.O. Box 84428, Riyadh, 11671, Saudi Arabia
2 Department of Statistics, St. Anthony’s College, Shillong, 793001, India
3 Faculty of Technology and Development, Zagazig University, Zagazig, 44519, Egypt
* Corresponding Author: Ahmed Elshahhat. Email:
(This article belongs to the Special Issue: Incomplete Data Test, Analysis and Fusion Under Complex Environments)
Computer Modeling in Engineering & Sciences 2024, 141(1), 459-489. https://doi.org/10.32604/cmes.2024.053255
Received 12 February 2024; Accepted 20 June 2024; Issue published 20 August 2024
Abstract
A novel inverted generalized gamma (IGG) distribution, proposed for data modelling with an upside-down bathtub hazard rate, is considered. In many real-world practical situations, when a researcher wants to conduct a comparative study of the life testing of items based on cost and duration of testing, censoring strategies are frequently used. From this point of view, in the presence of censored data compiled from the most well-known progressively Type-II censoring technique, this study examines different parameters of the IGG distribution. From a classical point of view, the likelihood and product of spacing estimation methods are considered. Observed Fisher information and the delta method are used to obtain the approximate confidence intervals for any unknown parametric function of the suggested model. In the Bayesian paradigm, the same traditional inferential approaches are used to estimate all unknown subjects. Markov-Chain with Monte-Carlo steps are considered to approximate all Bayes’ findings. Extensive numerical comparisons are presented to examine the performance of the proposed methodologies using various criteria of accuracy. Further, using several optimality criteria, the optimum progressive censoring design is suggested. To highlight how the proposed estimators can be used in practice and to verify the flexibility of the proposed model, we analyze the failure times of twenty mechanical components of a diesel engine.Keywords
Recently, we have come across several studies on inverse (or reciprocal) distributions of one or two parameters in the literature. Louzada et al. [1] recently emphasized that inverse distributions provide greater flexibility for fitting data and, in many cases, have been found to be better than many other standard distributions. The three parameter inverted generalized gamma (IGG) distribution was introduced by Hoq et al. [2] in the context of life testing experiments. As specific examples, the IGG distribution includes a number of lifespan distributions, including inverted gamma, inverted half-normal, inverted Weibull, inverted exponential, inverted Rayleigh, inverted Maxwell-Boltzmann, and inverted chi-square. The IGG distribution has been found to be a good alternative to other skewed distributions, including inverse gamma, inverse Weibull, and generalized inverse exponential distributions, and can be used to illustrate skewed data.
A random variable x is said to have IGG
and
where
and
where
The hazard rate function (4) has an unimodal shape, while the density function (2) is unimodal and right-skewed with heavy tails. These tails become thicker when the shape parameters are equal, and longer when the shape parameter
Besides Type-I, Type-II, and progressive Type-II censoring (PT2C) plans, several censoring mechanisms are available in the literature. An important advantage of PT2C is that it allows the experimenter to withdraw some live units before the experiment stops. The PT2C can be described as follows: Suppose we have
The maximum product spacing (MPS) was initially presented by Cheng et al. [4] and was utilized by Ranneby [5] as an alternative to the maximum likelihood (ML) estimation approach. In recent times, the MPS method has been employed in different contexts; see Zhu [6], Jeon et al. [7], Nassar et al. [8], Nassar et al. [9], and many others. According to Anatolyev et al. [10], the MPS approach adheres to the invariance property and outperforms likelihood estimators in small-sample scenarios for heavy and skewed distributions.
The limitations and challenges of this work are particularly related to the complexity of parameter estimation, computational intensity, small sample sizes, model power, hypothesis testing, and inference difficulties. Addressing these issues often requires advanced statistical techniques and careful consideration of the specific characteristics of the data and the censoring scheme.
Researchers pay little attention to analyzing new extended gamma lifetime models because of their complex results and expensive numerical evaluations. Aside from the work of Ramos et al. [11], as they studied Bayesian inferences based on non-informative priors using a complete sample, and no attempt has been made, to our knowledge, to estimate IGG parameters based on PT2C since the IGG distribution was introduced in the literature.
Given the usefulness and practicality of the IGG distribution, and with samples collected from the PT2C strategy, we state our main objectives in this paper as follows:
• Obtain the frequentist point estimators of the parameters (including
• Obtain the approximate confidence intervals (ACIs) for all parameters using the acquired ML estimators (MLEs) and MPS estimators (MPSEs).
• As an alternative to the ML function in the Bayesian paradigm, the MPS function is also taken into consideration. Next, both ML and MPS functions are explored in a Bayesian framework for all unknown parameters under squared error loss (SEL) with independent gamma priors.
• Implement Markov chain Monte Carlo (MCMC) methodology with Metropolis–Hastings (M–H) sampler to calculate the offered Bayes point estimates as well as Bayes confidence intervals (BCIs).
• Various optimality metrics are assessed to provide the optimal PT2C plan.
• Extensive Monte Carlo simulations are performed to evaluate the performance of the proposed techniques.
• An engineering data set representing diesel engine failure times is analyzed to show the applicability of the proposed methodologies in a real-life scenario.
The rest of the article is organized as follows: In Sections 2 and 3, we discuss ML- and MPS-based estimation. In Section 4, we discuss Bayesian inferences. Simulation investigations are highlighted in Section 5. In Section 6, the optimal PT2C is discussed. One real data set is analyzed in Section 7. Finally, various observations are made in Section 8.
This section considers the ML estimation to provide the MLEs along with their ACIs of the IGG parameters
Suppose
where
Using (5), without constant terms, the log-likelihood function
where
The MLEs
and
where
and
To prove the convergence of the MLEs
Figure 1: The log-LF (top) and its FPD (bottom) of (a)
In this subsection, the
It is established that
where
To find the
where
Hence, once we obtain the variances
respectively, where
3 Product of Spacings Estimation
This section examines the MPS estimation approach to acquire the MPSEs and ACIs of
Substituting (2) and (1) into the product of spacing (PS) function of PT2C, we get the joint PS function of
where
By maximizing the following logarithmic MPS function (e.g.,
where
Solving the following three normal equations will yield the MPSEs of
and
where
Since the proposed MPSEs from (13)–(15) lack closed form solutions, an iterative method such as Newton-Raphson can be easily used to derive the MPSEs numerically. It is important to note that the MPSEs and MLEs of
Now, to highlight the convergence of the MPSEs
Figure 2: The log-PS (top) and its FPD (bottom) of (a)
To get the two bounds
It follows that
Replacing
respectively, where
In this section, under the PT2C scheme, the Bayes framework for unknown IGG parameters based on LF and PF methods is discussed. Prior information plays an influential role in obtaining Bayes’ estimate. We now consider the independent gamma priors (say,
where
Suppose
where the Bayes estimator
4.1 Posterior Density via LF-Based
Using (5) and (18), the joint posterior PDF via the LF (say,
Using an arbitrary function
All triple integrals in (21) do not have a closed form. Therefore, we will implement the M-H algorithm. From (20), we note that the full conditionals of
4.2 Posterior Density via PS-Based
Using (11) and (18), the joint posterior PDF via PS-based (say,
Certainly, from (22), the Bayes estimator via PS-based (say
The performance of the proposed estimation methodologies is highlighted in this part. According to the algorithm proposed by Balakrishnan et al. [13] for various levels of
Once the simulated PT2C samples are acquired, the classical (point and 95% interval) estimations are offered. Following two independent information requirements called prior-mean and prior-variance, we can easily assign certain values to the proposed prior parameters; see Kundu [14]. Therefore, two different sets for the hyperparameter values of
To conduct the M-H sampling procedure, 12,000 MCMC samples are gathered from the posterior functions (20) and (22). For each simulated Markov chain, the first 2000 variates are removed to ignore the effect of the selection of initial guesses. In all proposed numerical simulations, we assume that all IGG model parameters are unknown. We also assume that the practitioner records the failure time of all test units
The performances of the point theoretical results of
where
All calculations are performed in
• In general, the proposed point (or interval) estimates of
• As
• Comparing the proposed point approaches, it is clear that:
– The MLE (or MCMC-LF) of
– The performance of
• Comparing the proposed interval approaches, it is clear that:
– The ACI-LF estimates of
– The AILs of ACI-PS estimates of
• The CPs of the BCI estimates are (in most cases) greater than the specified nominal level, while the ACI estimates are lower (or closer) to the specified nominal level.
• Due to the availability of gamma priors, Bayes’ MCMC-LF (or MCMC-PS) estimates of all unknown parameters outperform ML (or MPS) estimates. The same comment is also drawn in the context of comparing the BCI-LF (or BCI-PS) with the ACI-LF (or ACI-PS).
• Obviously, the variance of Prior-II is less than that of Prior-I. Therefore, all Bayes estimates of all unknown parameters developed from Prior-II using LF (or PS) data outperform those obtained based on other methods.
• Comparison of the proposed Scheme-1 (first stage) and Scheme-3 (last stage), it is clear that the point estimates of
• Regarding the interval estimates of
• As a tip, to obtain accurate estimates of any unknown life parameter when the proposed censored data are present, the experimenter should record an appropriate effective sample size, taking into account the total cost of the test.
• To sum up, the Bayes M-H procedure is recommended to study the unknown parameters of life of the IGG model in the presence of PT2C data.
The previous sections dealt with the derivation of point and interval estimations, using both frequentist and Bayesian MCMC estimations, for the parameters of life of the IGG distribution when samples are gathered from the PT2C strategy. Following Ng et al. [17], when the design of removal items
Thus, to select the optimal progressive censoring (OPC) plan, Table 11 reports common criteria for this purpose. Regarding the A-and D-optimality criteria, our objective is to reduce the trace and determinant values of estimated variances and covariances developed from the LF and PS methods. Further, the goal of F-optimality is to maximize the observed values of the Fisher matrices in relation to the MLE (or MPSE) (say,
This section analyzes a useful real data set consisting of the failure times (in weeks) of 20 mechanical components of a diesel engine to demonstrate the performance of the proposed methodologies; see Murthy et al. [20]. For computational convenience, we multiply each original data unit by one hundred, and the new transformed data set is: 6.70, 6.80, 7.60, 8.10, 8.40, 8.50, 8.50, 8.60, 8.90, 9.80, 9.80, 11.4, 11.4, 11.5, 12.1, 12.5, 13.1, 14.9, 16.0, and 48.5.
First, the IGG distribution is fitted to the complete diesel engine data with eleven inverted lifetime distributions (for
(1) Generalized inverted Gompertz (GIG(
(2) Alpha-power inverse-Weibull (APIW(
(3) Generalized inverse-Weibull (GIW(
(4) Exponentiated inverted-Weibull (EIW(
(5) Generalized inverted-exponential (GIE(
(6) Generalized inverted half-logistic (GIHL(
(7) Inverted exponentiated Rayleigh (IER(
(8) Inverted Nadarajah–Haghighi (INH(
(9) Inverted Gompertz (IGo(
(10) Inverse-Weibull (IW(
(11) Inverse gamma (IG(
To evaluate the feasibility of the IGG model in comparison with other popular models, several information measures are implemented, namely: (i) Kolmogorov-Smirnov (KS) statistic (with its p-value); (ii) Anderson-Darling (AD); (iii) Cramer von Mises (CvM); (iv) estimated negative log-likelihood (ENL); (v) Akaike information (AI); (vi) consistent Akaike information (CAIC); and (vii) Hannan-Quinn information (HQI).
The MLEs (with their standard-errors (SEs)) of
Figure 3: The Q–Q diagrams of IGG and its competitors from diesel engine data
To capture the behavior of the estimated PDFs and CDFs of the IGG and its competitive distributions using the diesel engine data, we have provided two plots: (a) representing the histogram and the fitted densities and (b) representing the fitted/empirical reliability lines; see Fig. 4. It demonstrates that the IGG distribution reflects the overall structure of the histograms and confirms the numerical results presented here.
Figure 4: Fitted densities and reliability lines of IGG and its competitors from diesel engine data
Using the complete diesel engine data, for different choices of
Tables 14 and 15 indicate that the computed estimates of
Figure 5: Trace plots of
Figure 6: Posterior histograms of
Now, using Table 13, the selection of the OPC plan is explored. So, from (10) and (16), the criteria A, D, and F are evaluated; see Table 16. It shows that the scheme
In this paper, various estimates of the inverted generalized gamma parameters
Acknowledgement: The authors would desire to express their thanks to the editor and the three anonymous referees for useful suggestions and valuable comments. The authors would also like to express their full thanks to the Deanship of Scientific Research and Libraries, Princess Nourah bint Abdulrahman University, through the Program of Research Project Funding after Publication, Grant No. (RPFAP-34-1445) for supporting this project.
Funding Statement: This research project was funded by the Deanship of Scientific Research and Libraries, Princess Nourah bint Abdulrahman University, through the Program of Research Project Funding after Publication, Grant No. (RPFAP-34-1445).
Author Contributions: The authors confirm contribution to the paper as follows: study conception and design: Refah Alotaibi, Sanku Dey, Ahmed Elshahhat; data collection: Refah Alotaibi, Ahmed Elshahhat; analysis and interpretation of results: Ahmed Elshahhat; draft manuscript preparation: Refah Alotaibi, Sanku Dey. All authors reviewed the results and approved the final version of the manuscript.
Availability of Data and Materials: The data that support the findings of this study are available within the paper.
Conflicts of Interest: The authors declare that they have no conflicts of interest to report regarding the present study.
References
1. Louzada F, Ramos PL, Nascimento D. The inverse Nakagami-m distribution: a novel approach in reliability. IEEE Trans Reliab. 2018;67(3):1030–42. [Google Scholar]
2. Hoq AKMS, Ali M. Estimation of parameters of a generalized life testing models. J Stat Res. 1974;9:67–79. [Google Scholar]
3. Balakrishnan N, Aggarwala R. Progressive censoring theory, methods and applications. Boston, MA, USA: Birkhäuser; 2000. [Google Scholar]
4. Cheng RCH, Amin NAK. Estimating parameters in continuous univariate distributions with a shifted origin. J R Stat Soc Series B. 1983;45(3):394–403. [Google Scholar]
5. Ranneby B. The maximum spacing method. An estimation method related to the maximum likelihood method. Scand J Stat. 1984;11(2):93–112. [Google Scholar]
6. Zhu T. Statistical inference of Weibull distribution based on generalized progressively hybrid censored data. J Comput Appl Math. 2020;371:112705. [Google Scholar]
7. Jeon YE, Kang SB, Seo JI. Maximum product of spacings under a generalized Type-II progressive hybrid censoring scheme. Commun Stat Appl Methods. 2022;29(6):665–77. [Google Scholar]
8. Nassar M, Dey S, Wang L, Elshahhat A. Estimation of Lindley constant-stress model via product of spacing with Type-II censored accelerated life data. Commun Stat Simul Comput. 2024;53(1):288–314. [Google Scholar]
9. Nassar M, Alotaibi R, Elshahhat A. E-Bayesian estimation using spacing function for inverse Lindley adaptive Type-I progressively censored samples: comparative study with applications. Appl Bionics Biomech. 2024;2024(1):5567457. [Google Scholar] [PubMed]
10. Anatolyev S, Kosenok G. An alternative to maximum likelihood based on spacings. Econom Theory. 2005;21(2):472–6. [Google Scholar]
11. Ramos PL, Mota AL, Ferreira PH, Ramos E, Tomazella VL, Louzada F. Bayesian analysis of the inverse generalized gamma distribution using objective priors. J Stat Comput Simul. 2021;91(4):786–816. [Google Scholar]
12. Greene WH. Econometric analysis. 7th ed. Upper Saddle River, New Jersey: Pearson Prentice-Hall; 2012. [Google Scholar]
13. Balakrishnan N, Cramer E. The art of progressive censoring. Birkhäuser, New York: Springer; 2014. [Google Scholar]
14. Kundu D. Bayesian inference and life testing plan for the Weibull distribution in presence of progressive censoring. Technometrics. 2008;50(2):144–54. [Google Scholar]
15. Plummer M, Best N, Cowles K, Vines K. Coda: convergence diagnosis and output analysis for MCMC. R News. 2006;6(1):7–11. [Google Scholar]
16. Henningsen A, Toomet O. maxLik: a package for maximum likelihood estimation in R. Comput Stat. 2011;26(3):443–58. [Google Scholar]
17. Ng HKT, Chan PS, Balakrishnan N. Optimal progressive censoring plans for the Weibull distribution. Technometrics. 2004;46:470–81. [Google Scholar]
18. Elshahhat A, Nassar M. Bayesian survival analysis for adaptive Type-II progressive hybrid censored Hjorth data. Comput Stat. 2021;36(3):1965–90. [Google Scholar]
19. Elshahhat A, Abu El Azm WS. Statistical reliability analysis of electronic devices using generalized progressively hybrid censoring plan. Qual Reliab Eng Int. 2022;38(2):1112–30. doi:10.1002/qre.3058. [Google Scholar] [CrossRef]
20. Murthy DNP, Xie M, Jiang R. Weibull models. In: Wiley series in probability and statistics. Hoboken: Wiley; 2004. [Google Scholar]
21. Elshahhat A, Aljohani HM, Afify AZ. Bayesian and classical inference under Type-II censored samples of the extended inverse Gompertz distribution with engineering applications. Entropy. 2021;23(12):1578. doi:10.3390/e23121578. [Google Scholar] [PubMed] [CrossRef]
22. Basheer AM. Alpha power inverse Weibull distribution with reliability application. J Taibah Univ Sci. 2019;13(1):423–32. doi:10.1080/16583655.2019.1588488. [Google Scholar] [CrossRef]
23. De Gusmão FR, Ortega EM, Cordeiro GM. The generalized inverse Weibull distribution. Stat Pap. 2011;52(3):591–619. doi:10.1007/s00362-009-0271-3. [Google Scholar] [CrossRef]
24. Flaih A, Elsalloukh H, Mendi E, Milanova M. The exponentiated inverted Weibull distribution. Appl Math Inf Sci. 2012;6:167–71. [Google Scholar]
25. Abouammoh AM, Alshingiti AM. Reliability estimation of generalized inverted exponential distribution. J Stat Comput Simul. 2009;79(11):1301–15. doi:10.1080/00949650802261095. [Google Scholar] [CrossRef]
26. Potdar KG, Shirke DT. Inference for the parameters of generalized inverted family of distributions. ProbStat Forum. 2013;6:18–28. [Google Scholar]
27. Ghitany ME, Tuan VK, Balakrishnan N. Likelihood estimation for a general class of inverse exponentiated distributions based on complete and progressively censored data. J Stat Comput Simul. 2014;84(1):96–106. doi:10.1080/00949655.2012.696117. [Google Scholar] [CrossRef]
28. Tahir MH, Cordeiro GM, Ali S, Dey S, Manzoor A. The inverted Nadarajah-Haghighi distribution: estimation methods and applications. J Stat Comput Simul. 2018;88(14):2775–98. doi:10.1080/00949655.2018.1487441. [Google Scholar] [CrossRef]
29. Eliwa MS, El-Morshedy M, Ibrahim M. Inverse Gompertz distribution: properties and different estimation methods with application to complete and censored data. Ann Data Sci. 2019;6(2):321–39. doi:10.1007/s40745-018-0173-0. [Google Scholar] [CrossRef]
30. Keller AZ, Goblin MT, Farnworth NR. Reliability analysis of commercial vehicle engine. Reliab Eng. 1985;10(1):15–25. doi:10.1016/0143-8174(85)90039-3. [Google Scholar] [CrossRef]
31. Glen AG. On the inverse gamma as a survival distribution. J Qual Technol. 2011;43(2):158–66. doi:10.1080/00224065.2011.11917853. [Google Scholar] [CrossRef]
Appendix A: Fisher-LF Elements
Differentiating (6) with respect to the unknown parameters
and
where
and
Appendix B: Fisher-PS Elements
Differentiating (12) with respect to the unknown parameters
and
where
Cite This Article
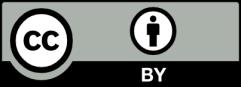
This work is licensed under a Creative Commons Attribution 4.0 International License , which permits unrestricted use, distribution, and reproduction in any medium, provided the original work is properly cited.