Open Access
ARTICLE
Integrating Transformer and Bidirectional Long Short-Term Memory for Intelligent Breast Cancer Detection from Histopathology Biopsy Images
1 Department of Computer Science, College of Computer Science, King Khalid University, Abha, 61451, Saudi Arabia
2 College of Science and Arts in Rijal Alma, King Khalid University, Abha, 61451, Saudi Arabia
3 Department of Information Technology, Muffakham Jah College of Engineering and Technology, Hyderabad, 500034, India
4 Department of Computer Science and Business Systems, Rajalakshmi Engineering College, Thandalam, 602105, India
* Corresponding Author: Prasanalakshmi Balaji. Email:
(This article belongs to the Special Issue: Intelligent Medical Decision Support Systems: Methods and Applications)
Computer Modeling in Engineering & Sciences 2024, 141(1), 443-458. https://doi.org/10.32604/cmes.2024.053158
Received 25 April 2024; Accepted 03 July 2024; Issue published 20 August 2024
Abstract
Breast cancer is a significant threat to the global population, affecting not only women but also a threat to the entire population. With recent advancements in digital pathology, Eosin and hematoxylin images provide enhanced clarity in examining microscopic features of breast tissues based on their staining properties. Early cancer detection facilitates the quickening of the therapeutic process, thereby increasing survival rates. The analysis made by medical professionals, especially pathologists, is time-consuming and challenging, and there arises a need for automated breast cancer detection systems. The upcoming artificial intelligence platforms, especially deep learning models, play an important role in image diagnosis and prediction. Initially, the histopathology biopsy images are taken from standard data sources. Further, the gathered images are given as input to the Multi-Scale Dilated Vision Transformer, where the essential features are acquired. Subsequently, the features are subjected to the Bidirectional Long Short-Term Memory (Bi-LSTM) for classifying the breast cancer disorder. The efficacy of the model is evaluated using divergent metrics. When compared with other methods, the proposed work reveals that it offers impressive results for detection.Keywords
Cite This Article
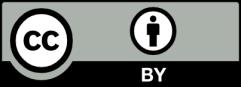
This work is licensed under a Creative Commons Attribution 4.0 International License , which permits unrestricted use, distribution, and reproduction in any medium, provided the original work is properly cited.