Open Access
ARTICLE
Incorporating Lasso Regression to Physics-Informed Neural Network for Inverse PDE Problem
1 National Key Lab of Aerospace Power System and Plasma Technology, Xi’an Jiaotong University, Xi’an, 710049, China
2 School of Mechanical Engineering, Xi’an Jiaotong University, Xi’an, 710049, China
3 Department of Electrical and Computer Engineering, University of Massachusetts Lowell, Lowell, MA 01854, USA
* Corresponding Author: Meng Ma. Email:
(This article belongs to the Special Issue: Machine Learning Based Computational Mechanics)
Computer Modeling in Engineering & Sciences 2024, 141(1), 385-399. https://doi.org/10.32604/cmes.2024.052585
Received 04 April 2024; Accepted 31 May 2024; Issue published 20 August 2024
Abstract
Partial Differential Equation (PDE) is among the most fundamental tools employed to model dynamic systems. Existing PDE modeling methods are typically derived from established knowledge and known phenomena, which are time-consuming and labor-intensive. Recently, discovering governing PDEs from collected actual data via Physics Informed Neural Networks (PINNs) provides a more efficient way to analyze fresh dynamic systems and establish PED models. This study proposes Sequentially Threshold Least Squares-Lasso (STLasso), a module constructed by incorporating Lasso regression into the Sequentially Threshold Least Squares (STLS) algorithm, which can complete sparse regression of PDE coefficients with the constraints of l norm. It further introduces PINN-STLasso, a physics informed neural network combined with Lasso sparse regression, able to find underlying PDEs from data with reduced data requirements and better interpretability. In addition, this research conducts experiments on canonical inverse PDE problems and compares the results to several recent methods. The results demonstrated that the proposed PINN-STLasso outperforms other methods, achieving lower error rates even with less data.Keywords
Cite This Article
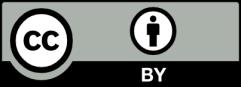
This work is licensed under a Creative Commons Attribution 4.0 International License , which permits unrestricted use, distribution, and reproduction in any medium, provided the original work is properly cited.