Open Access
ARTICLE
Artificial Intelligence Prediction of One-Part Geopolymer Compressive Strength for Sustainable Concrete
1 Department of Civil Engineering, College of Engineering, Jouf University, Sakaka, 72388, Saudi Arabia
2 Department of Civil Engineering, University of Engineering and Technology, Peshawar, 54890, Pakistan
3 Civil Engineering Department, Faculty of Engineering, Al-Azhar University, Cairo, 11884, Egypt
4 Civil Engineering Department, Faculty of Engineering, Aswan University, Aswan, 81528, Egypt
* Corresponding Author: Ahmed. M. Yosri. Email:
(This article belongs to the Special Issue: Recent Advances in Computational Methods for Performance Assessment of Engineering Structures and Materials against Dynamic Loadings)
Computer Modeling in Engineering & Sciences 2024, 141(1), 525-543. https://doi.org/10.32604/cmes.2024.052505
Received 03 April 2024; Accepted 12 June 2024; Issue published 20 August 2024
Abstract
Alkali-activated materials/geopolymer (AAMs), due to their low carbon emission content, have been the focus of recent studies on ecological concrete. In terms of performance, fly ash and slag are preferred materials for precursors for developing a one-part geopolymer. However, determining the optimum content of the input parameters to obtain adequate performance is quite challenging and scarcely reported. Therefore, in this study, machine learning methods such as artificial neural networks (ANN) and gene expression programming (GEP) models were developed using MATLAB and GeneXprotools, respectively, for the prediction of compressive strength under variable input materials and content for fly ash and slag-based one-part geopolymer. The database for this study contains 171 points extracted from literature with input parameters: fly ash concentration, slag content, calcium hydroxide content, sodium oxide dose, water binder ratio, and curing temperature. The performance of the two models was evaluated under various statistical indices, namely correlation coefficient (R), mean absolute error (MAE), and root mean square error (RMSE). In terms of the strength prediction efficacy of a one-part geopolymer, ANN outperformed GEP. Sensitivity and parametric analysis were also performed to identify the significant contributor to strength. According to a sensitivity analysis, the activator and slag contents had the most effects on the compressive strength at 28 days. The water binder ratio was shown to be directly connected to activator percentage, slag percentage, and calcium hydroxide percentage and inversely related to compressive strength at 28 days and curing temperature.Keywords
Cite This Article
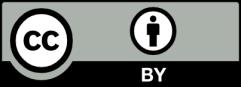
This work is licensed under a Creative Commons Attribution 4.0 International License , which permits unrestricted use, distribution, and reproduction in any medium, provided the original work is properly cited.