Open Access
ARTICLE
Explainable Artificial Intelligence (XAI) Model for Cancer Image Classification
1 Department of Computer Science & Engineering, Raj Kumar Goel Institute of Technology, Ghaziabad, 201017, India
2 Department of School of Computing Science and Engineering, Galgotias University, Greater Noida, 203201, India
3 Tecnológico Nacional de México Campus Tijuana, Baja California, 22414, México
4 Universidad Autónoma de Baja California, Tijuana, Baja California, 22414, México
5 Department of Computer Science and Engineering, G.L, Bajaj Institute of Technology and Management (GLBITM), Affiliated to Dr. A. P. J. Abdul Kalam Technical University, Lucknow, 201306, India
* Corresponding Author: Satya Prakash Yadav. Email:
(This article belongs to the Special Issue: Intelligent Medical Decision Support Systems: Methods and Applications)
Computer Modeling in Engineering & Sciences 2024, 141(1), 401-441. https://doi.org/10.32604/cmes.2024.051363
Received 04 March 2024; Accepted 22 May 2024; Issue published 20 August 2024
Abstract
The use of Explainable Artificial Intelligence (XAI) models becomes increasingly important for making decisions in smart healthcare environments. It is to make sure that decisions are based on trustworthy algorithms and that healthcare workers understand the decisions made by these algorithms. These models can potentially enhance interpretability and explainability in decision-making processes that rely on artificial intelligence. Nevertheless, the intricate nature of the healthcare field necessitates the utilization of sophisticated models to classify cancer images. This research presents an advanced investigation of XAI models to classify cancer images. It describes the different levels of explainability and interpretability associated with XAI models and the challenges faced in deploying them in healthcare applications. In addition, this study proposes a novel framework for cancer image classification that incorporates XAI models with deep learning and advanced medical imaging techniques. The proposed model integrates several techniques, including end-to-end explainable evaluation, rule-based explanation, and user-adaptive explanation. The proposed XAI reaches 97.72% accuracy, 90.72% precision, 93.72% recall, 96.72% F1-score, 9.55% FDR, 9.66% FOR, and 91.18% DOR. It will discuss the potential applications of the proposed XAI models in the smart healthcare environment. It will help ensure trust and accountability in AI-based decisions, which is essential for achieving a safe and reliable smart healthcare environment.Keywords
Cite This Article
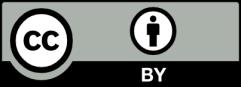
This work is licensed under a Creative Commons Attribution 4.0 International License , which permits unrestricted use, distribution, and reproduction in any medium, provided the original work is properly cited.