Open Access
ARTICLE
Enhancing Communication Accessibility: UrSL-CNN Approach to Urdu Sign Language Translation for Hearing-Impaired Individuals
1 Department of Computer Engineering, Modeling Electronics and Systems Engineering, University of Calabria, Rende Cosenza, 87036, Italy
2 Department of Information Systems, University of Management and Technology, Lahore, 54770, Pakistan
3 Department of Computer Engineering, Istanbul Sabahattin Zaim University, Istanbul, 34303, Turkey
4 Department of Software Engineering, Istanbul Nisantasi University, Istanbul, 34398, Turkey
5 Department of Computer Science, COMSATS University Islamabad, Lahore Campus, Lahore, 54700, Pakistan
6 Faculty of Medicine and Health Technology, Tampere University, Tampere, 33720, Finland
7 Department of Computer Science, College of Computer Engineering and Sciences in Al-Kharj, Prince Sattam Bin Abdulaziz University, P.O. Box 151, Al-Kharj, 11942, Saudi Arabia
8 Second Department of Computer Science, College of Engineering and Computing, George Mason University, Fairfax, VA 4418, USA
* Corresponding Authors: Jawad Rasheed. Email: ; Tunc Asuroglu. Email:
(This article belongs to the Special Issue: Artificial Intelligence Emerging Trends and Sustainable Applications in Image Processing and Computer Vision)
Computer Modeling in Engineering & Sciences 2024, 141(1), 689-711. https://doi.org/10.32604/cmes.2024.051335
Received 02 March 2024; Accepted 03 June 2024; Issue published 20 August 2024
Abstract
Deaf people or people facing hearing issues can communicate using sign language (SL), a visual language. Many works based on rich source language have been proposed; however, the work using poor resource language is still lacking. Unlike other SLs, the visuals of the Urdu Language are different. This study presents a novel approach to translating Urdu sign language (UrSL) using the UrSL-CNN model, a convolutional neural network (CNN) architecture specifically designed for this purpose. Unlike existing works that primarily focus on languages with rich resources, this study addresses the challenge of translating a sign language with limited resources. We conducted experiments using two datasets containing 1500 and 78,000 images, employing a methodology comprising four modules: data collection, pre-processing, categorization, and prediction. To enhance prediction accuracy, each sign image was transformed into a greyscale image and underwent noise filtering. Comparative analysis with machine learning baseline methods (support vector machine, Gaussian Naive Bayes, random forest, and k-nearest neighbors’ algorithm) on the UrSL alphabets dataset demonstrated the superiority of UrSL-CNN, achieving an accuracy of 0.95. Additionally, our model exhibited superior performance in Precision, Recall, and F1-score evaluations. This work not only contributes to advancing sign language translation but also holds promise for improving communication accessibility for individuals with hearing impairments.Keywords
Cite This Article
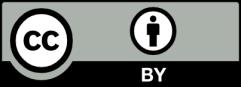
This work is licensed under a Creative Commons Attribution 4.0 International License , which permits unrestricted use, distribution, and reproduction in any medium, provided the original work is properly cited.