Open Access
ARTICLE
CoopAI-Route: DRL Empowered Multi-Agent Cooperative System for Efficient QoS-Aware Routing for Network Slicing in Multi-Domain SDN
College of Engineering Guindy, Anna University, Chennai, 600025, India
* Corresponding Author: Meignanamoorthi Dhandapani. Email:
Computer Modeling in Engineering & Sciences 2024, 140(3), 2449-2486. https://doi.org/10.32604/cmes.2024.050986
Received 24 February 2024; Accepted 29 April 2024; Issue published 08 July 2024
Abstract
The emergence of beyond 5G networks has the potential for seamless and intelligent connectivity on a global scale. Network slicing is crucial in delivering services for different, demanding vertical applications in this context. Next-generation applications have time-sensitive requirements and depend on the most efficient routing path to ensure packets reach their intended destinations. However, the existing IP (Internet Protocol) over a multi-domain network faces challenges in enforcing network slicing due to minimal collaboration and information sharing among network operators. Conventional inter-domain routing methods, like Border Gateway Protocol (BGP), cannot make routing decisions based on performance, which frequently results in traffic flowing across congested paths that are never optimal. To address these issues, we propose CoopAI-Route, a multi-agent cooperative deep reinforcement learning (DRL) system utilizing hierarchical software-defined networks (SDN). This framework enforces network slicing in multi-domain networks and cooperative communication with various administrators to find performance-based routes in intra- and inter-domain. CoopAI-Route employs the Distributed Global Topology (DGT) algorithm to define inter-domain Quality of Service (QoS) paths. CoopAI-Route uses a DRL agent with a message-passing multi-agent Twin-Delayed Deep Deterministic Policy Gradient method to ensure optimal end-to-end routes adapted to the specific requirements of network slicing applications. Our evaluation demonstrates CoopAI-Route’s commendable performance in scalability, link failure handling, and adaptability to evolving topologies compared to state-of-the-art methods.Keywords
Cite This Article
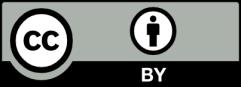
This work is licensed under a Creative Commons Attribution 4.0 International License , which permits unrestricted use, distribution, and reproduction in any medium, provided the original work is properly cited.