Open Access
ARTICLE
An Integrated Bipolar Picture Fuzzy Decision Driven System to Scrutinize Food Waste Treatment Technology through Assorted Factor Analysis
1 Department of Mathematics, Bharathiar University, Coimbatore, 641046, India
2 Department of Mathematics, Sri Ramakrishna Mission Vidyalaya College of Arts and Science, Coimbatore, 641020, India
3 Department of Operations Research and Statistics, Faculty of Organizational Sciences, University of Belgrade, Belgrade, 11000, Serbia
4 College of Engineering, Department of Industrial Engineering and Management, Yuan Ze University, Taoyuan City, 320315, Taiwan
5 Faculty of Transport and Traffic Engineering, University of Belgrade, Vojvode Stepe 305, Belgrade, 11000, Serbia
6 Department of Computer Science and Engineering, College of Informatics, Korea University, Seoul, 02841, Republic of Korea
7 School of Business, Istanbul Medipol University, Istanbul, 34083, Turkey
8 Department of Economics and Management, Khazar University, Baku, Azerbaijan
* Corresponding Author: Dragan Pamucar. Email:
(This article belongs to the Special Issue: Control Systems and Machine Learning for Intelligent Computing)
Computer Modeling in Engineering & Sciences 2024, 140(3), 2665-2687. https://doi.org/10.32604/cmes.2024.050954
Received 23 February 2024; Accepted 16 May 2024; Issue published 08 July 2024
Abstract
Food Waste (FW) is a pressing environmental concern that affects every country globally. About one-third of the food that is produced ends up as waste, contributing to the carbon footprint. Hence, the FW must be properly treated to reduce environmental pollution. This study evaluates a few available Food Waste Treatment (FWT) technologies, such as anaerobic digestion, composting, landfill, and incineration, which are widely used. A Bipolar Picture Fuzzy Set (BPFS) is proposed to deal with the ambiguity and uncertainty that arise when converting a real-world problem to a mathematical model. A novel Criteria Importance Through Intercriteria Correlation-Stable Preference Ordering Towards Ideal Solution (CRITIC-SPOTIS) approach is developed to objectively analyze FWT selection based on thirteen criteria covering the industry’s technical, environmental, and entrepreneurial aspects. The CRITIC method is used for the objective analysis of the importance of each criterion in FWT selection. The SPOTIS method is adopted to rank the alternative hassle-free, following the criteria. The proposed model offers a rank reversal-free model, i.e., the rank of the alternatives remains unaffected even after the addition or removal of an alternative. In addition, comparative and sensitivity analyses are performed to ensure the reliability and robustness of the proposed model and to validate the proposed result.Keywords
Though raw food materials can be preserved and have an extended shelf life, their shelf life becomes substantially shorter once they are processed into consumable food products. When the cooked food is left unconsumed, it soon turns into Food Waste (FW). Since the population density is higher in urban areas owing to the employment opportunities provided by the industries, a higher concentration of population in the cities entails larger volumes of food requirements. Furthermore, global markets promote international culinary trends. A worldwide trend has been sparked by multi-cuisine restaurants, exotic fruits and vegetables imported from other countries, immigration to other countries, etc. According to [1], with increased income rates, the consumption of processed and fast foods has been increasing. Dining in hotels and restaurants has become an inevitable part of the lifestyle of youths in urban areas. More and more restaurants and food chains are opening up every day. The recent COVID-19 outbreak has caused a significant change in the eating habits of people. Youngsters shifted to mindful eating by including fruits and vegetables that are rich in nutrients and having more balanced diets. The work-from-home culture has created opportunities to try diversified cuisine, be it cooking at home or getting it delivered from hotels.
FW is a common source of concern in all countries across the globe. Liu et al. [2] said that globally, approximately 930 million tonnes of food are wasted annually. China contributes about 3.1236 million tonnes of FW per year. According to [3], the FW in China is expected to increase to 100–132.82 million tonnes by 2040. Nearly 81,200 metric tonnes of food are produced in Bristol, one of the major cities in the UK. But one-fifth of the annual production of the food is wasted in Bristol [4]. The EU countries, such as Portugal, Greece, Denmark, Belgium, and Cyprus, produce FW of about 184, 191, 221, 250, and 397 kg/capita, respectively. Other countries like Sweden, Bulgaria, Slovakia, Croatia, and Slovenia have recorded 87, 86, 83, 71, and 68 kg/capita of FW, respectively. India has a Global Hunger Index (GHI) score of 28.7, which is considered serious on the GHI severity scale, placing it 111th out of 125 nations in 2023. The report of the United Nations Environment Programme estimates that Indian households generate FW of about 50 kg/capita. India is said to generate 68.7 million tonnes of FW every year. FW, when left untreated, becomes the source of carbon footprints and mental and health issues. The untreated waste produces harmful and putrid gases due to the breaking down of the food molecules as a result of decomposition.
Hence, it is imperative to treat the FW to ensure a safe and clean environment. Various techniques have been introduced over the years for the treatment of the FW. Each approach has a unique set of advantages as well as shortcomings. An ideal food waste treatment technology minimizes the cost and maximizes the profit. This necessitates the need for a decision-making algorithm for the optimal selection of treatment technology. Real-life problems are more often ambiguous and have different parameters. Hence, to address the ambiguity, fuzzy logic has been incorporated into this study. In this literature, various technologies such as landfills, anaerobic digestion, incineration, and composting have been taken into account. The construction of a mathematical model for the Food Waste Treatment (FWT) selection involves addressing and quantifying certain dilemmas such as acceptance of society. The presence of the FWT plant raises concerns about odor, pollution, and safety. The perception of the public on the plant can take satisfaction, abstinence, or dissatisfaction degrees. Hence the Bipolar Picture Fuzzy Set (BPFS) set is proposed to measure positive and negative aspects of the satisfaction, abstinence, or dissatisfaction degrees. The nomenclature of the acronyms defined in this paper is given in the Table 1.
FW is a major concern in terms of health and environmental issues. Various studies have been made to find the optimal FWT selection. Das et al. [5] have examined the production of biogas, bioethanol, and fertilizer from FW. Paranjpe et al. [6] have analyzed the production of methane in Anaerobic Digestion (AD) and have suggested co-digestion techniques to increase the methane production. Xiong et al. [7] have experimented on the ammonia emission from the food waste composting when the FW is mixed with sulphur. Roy et al. [8] have discussed the environmental, social, and economic aspects of FW valorization. Bhatia et al. [9] have examined the application of anaerobic digestion and composting technologies for the reduction of carbon footprint from FW. Fei et al. [10] have assessed the treatment of FW in small and medium cities of China and have evaluated the technology using Life Cycle Assessment (LCA) and cost-benefit analysis. Garcia et al. [11] have studied extensively the available FWT techniques. Sakcharoen et al. [12] analyzed the factors that are affecting FW management. For instance, Buyuk et al. [13] have analyzed the best FWT selection method using the Spherical Fuzzy Analytic Hierarchy Process. Bernstad et al. [14] have compared three technologies to treat household food waste. Angelo et al. [15] conducted an extensive study on LCA on the FWT selection for Rio de Janeiro. Liu et al. [16] have conducted a study for the site-selection FW treatment plant. Gerassimidou et al. [17] have focused on the FW treatment in the food sector in US. Gao et al. [18] have studied the impact of the FWT technologies on the environment. Rani et al. [19] have introduced a neutrosophic fuzzy set to the FWT technology selection problem to provide neutrality in the situation at hand. Rani et al. [20] have studied the FWT methods using the MEthod based on the REmoval effects of Criteria (MEREC) method to ensure the objective analysis of the problem.
Zadeh introduced fuzzy sets for the first time in 1965 [21]. Subsequently, numerous studies expanded upon Zadeh’s original fuzzy sets. Type-2 fuzzy sets were introduced in 1971, defining the membership function within the unit interval [0, 1] rather than a singular point within the same interval [22]. Set-valued fuzzy Sets (SVFS) were defined in 1976, where the membership degree was expressed as a subset of [0, 1]. Interval-valued Fuzzy Sets (IVFS), initially termed
MCDM approaches are useful for determining the most viable option among a group of prospects. The order of preference of the new set of alternatives changes in traditional ranking techniques when an alternative is added to or deleted from the previous set of alternatives known as Rank Reversal Problem (RRP) [38]. This is not preferable, as the change in the order of preference of alternatives means the loss of credibility of the mathematical model. MCDM methods such as Analytic Hierarchy Process (AHP) [39], Technique for Order of Preference by Similarity to Ideal Solution (TOPSIS) [40,41], ELimination and Choice Translating REality (ELECTRE) [42], VIekriterijumsko KOmpromisno Rangiranje (VIKOR) [43] and Preference Ranking Organization METHod for Enrichment Evaluation (PROMETHEE) [44] are not free from RRP. Hence, the SPOTIS method was developed as a rank-reversal-free (RRF) method that considers the independence of the criteria. Dezert et al. [45] introduced SPOTIS as an alternative to the traditional RRP methods. Characteristic Objects Method (COMET) is another such method that is free from RRP [46]. Since SPOTIS is a recently developed method, there are only a few research papers that have incorporated this method. Shekhovtsov et al. [47] have extended the SPOTIS method and incorporated it into decision-making problems. de Azevdo et al. [48] proposed a hybrid MEREC-SPOTIS method for the ordering of photovoltaic cells. Wieckowski et al. [49] have compared the two existing RRF methods, COMET and SPOTIS, and concluded that SPOTIS is more robust as compared to COMET. Baczkiewicz et al. [50] applied COMET and SPOTIS methods in the analysis of solar panels.
In MCDM, alternatives are evaluated based on their relevance to the criteria. In order to establish significance, it is imperative to assign weights to each criterion [51]. Several weighting methods can be used to assign weights to each criterion. Traditionally, subjective weighting methods like pairwise comparison methods [52], Stepwise Weight Assessment Ratio Analysis (SWARA) [53], AHP [54], Analytic Network Process (ANP) [55], Decision-Making Trial and Evaluation Laboratory (DEMATEL) [56], and Best Worst Method (BWM) [57] were employed to assign weights to each criterion. In these subjective weighting methods, weights were assigned to the criteria based on information from the decision-maker. If the decision-maker either had a biased opinion on the criteria or lacked extensive knowledge about each criterion, the results would be inaccurate [58]. Objective-based weighting techniques were developed to address this issue. They construct weights by evaluating the data included in the decision matrix. The majority of objective-based weighing algorithms use the entropy method [59]. CRITIC, an objective weighting method, was developed by [60] in 1995. This approach was predicated on quantifying the core ideas of contrast intensity and the criteria’s contradictory nature. Contrast intensity refers to the variation of the criteria from one another. Conflicting character refers to the conflicting nature of each criterion. Criterion with higher contrast intensity and conflicting character is given greater weight in the CRITIC method [61]. Some of the other objective-based weighting methods are Integrated Determination of Objective CRIteria Weights (IDOCRIW) [62], MEREC [63], and Criterion Impact Loss (CILOS) [64]. CRITIC has been widely employed to assign weights. A strategy for selecting suppliers that combine the CRITIC approach with spherical fuzzy sets has been presented by [65]. Vujičić et al. [66] have performed a comparative analysis of the objective-based weighting methods: the CRITIC and entropy methods. For the purpose of evaluating the service status groynes in the waterways, Zhang et al. [67] have offered a combined AHP-CRITIC technique that provided both subjective and objective analysis. Parthasarathy et al. [68] employed the CRITIC and EXtended PROMETHEE II (EXPROM-II) methodologies for renewable energy selection under multiple parametric conditions.
Based on the prior studies, we have identified the following concerns:
• Though various studies have been conducted on FWT selection, most of them primarily assessed the various FWT technologies from an environmental perspective. They focused on selecting a technology that causes the least amount of pollution in terms of the environment. Various other aspects, such as social acceptance, viability, and the liquid assets involved, were not taken into account. This results in overlooking the key factors that affect the FWT selection.
• Most of the studies on FWT were based on subjective weighing methods. This poses the risk of biased opinions or partial information from the decision-makers regarding the criteria. These methods depend significantly on the knowledge of the experts causing unreliability in the process of decision making.
• Traditional ranking methods suffered from RRP. This resulted in complex procedures and increased calculation time. Further research was not able to identify the root cause of this phenomenon. This necessitates the need for a ranking method that is free from RRP.
The contribution of the proposed model is as follows:
• The FWT has been evaluated on an environmental, social, economical, and technological basis for better adaptation of the technology in real life.
• This study presents an objective analysis of the criteria using the CRITIC method, ensuring neutrality during the evaluation of FWT.
• This study proposes the use of BPFS for the quantification of the ambiguity present in real-life scenarios. This helps in the handling of bipolarity in terms of belongingness, neutrality, and non-belongingness.
• This study explores the novel SPOTIS rank-reversal-free method to ensure the robustness of the decision-making process.
Definition 3.1. [30,37] Let
where
Definition 3.2. [35,37] Let
where
A basic element
Definition 3.3. [37] A BPFS
where
The PMD
Definition 3.4. [37] Let
1.
2.
The range of SF
4.1 The CRITIC-SPOTIS Approach
The CRITIC technique was first presented in 1995 with the primary goal of calculating the weight of each characteristic. This utilizes an objective-weighted approach where the weights are determined using a decision matrix. The approach allows for the conversion of qualitative qualities into quantitative attributes without requiring the attributes to be independent. The data is obtained by means of an analytical analysis of the decision matrix, which is utilized to meet the evaluation standards in the specified procedure. The SPOTIS approach is a methodology for preventing rank reversals in MCDM situations. The SPOTIS approach sets minimum and maximum boundaries for each of the problem’s criteria. By converting the initially ambiguous MCDM issue into a well-defined one, these constraints aid in avoiding rank reversal. The SPOTIS method was applied to the assessment of the alternatives. The recommended technique entails the following steps:
Step 1: Problem statement: Choose a set of m alternatives
where i = 1, 2, ..., m and j = 1, 2, ..., n represent the BPFS supplied by the decision makers (DMs). As a result,
Step 2: Formation of the linguistic decision matrix under BPFS environment.
Step 3: The score function is applied to the decision matrix to normalize the membership values.
Step 4: Assigning the weights to the chosen criteria.
Step 5: Normalization of the decision matrix.
Step 6: CRITIC method.
Step 6.1: The first stage of the CRITIC method is the normalization of the decision matrix based on the positive and negative attributes given in the formula below:
where
Step 6.2: Calculating the characteristic’s standard deviation with the following formula (2):
where
Step 6.3: The correlation coefficient between criteria is estimated using (3).
where
Step 6.4: The index of the
Step 6.5: Finally, the weight of each criterion is determined by the formula (5).
Step 7: SPOTIS method.
Step 7.1: Establishing the maximum
Step 7.2: The Ideal Solution Point (ISP) is determined by the bounds of the attributes. The optimal alternative is selected as the one with the shortest distance from the ISP.
Step 7.3: To determine the distance between each alternative and the ISP, we initially assess the Euclidean distance for every alternative
where
Step 7.4: Determine the normalized average distance using the following formulae given below:
Step 7.5: Rank the alternatives in ascending order values of
FW is an imminent cause of environmental pollution due to the carbon footprint occurring during the decomposition of the FW. Hence, it is imperative to provide proper disposal and treatment of the FW. Various techniques have been employed for the FW treatment. In this study, we have considered four such techniques: anaerobic digestion (
Anaerobic Digestion: In this procedure, food waste undergoes decomposition in the absence of oxygen, facilitated by microorganisms that convert the organic matter into biogas and a residue known as digestate. Typically, this process occurs within a sealed vessel known as a reactor, which is widely employed due to its effectiveness in treating substrates rich in biodegradable organic matter and high moisture content. Food waste is gathered from various sources including kitchens and restaurants, then subjected to further screening to remove non-organic materials such as plastics and glass. Subsequently, the pre-cleaned food waste is introduced into the reactor for anaerobic digestion. Microorganisms within the reactor convert the food waste into biogas and a nutrient-rich material called digestate, which can be utilized as fertilizer in agriculture. The methane produced serves as a renewable energy source, thereby mitigating global warming by reducing greenhouse gas emissions that would otherwise occur during waste decomposition in landfills. Although the initial setup costs for anaerobic digestion are relatively high, the process involves technical intricacies necessitating regular monitoring of factors such as pH levels and temperature, leading to increased maintenance requirements. Proper management of the digestate is crucial for effective operation.
Composting: Composting, akin to anaerobic digestion, involves the breakdown of food waste by microbes, albeit in the presence of oxygen. Initially, collected food waste undergoes pre-treatment to eliminate harmful substances such as glass and plastics. Subsequently, the food waste is mixed with sawdust to expedite the process. Co-composting, on the other hand, involves combining multiple feedstocks to enhance compost quality. Through co-composting, organic waste is transformed into a valuable organic product known as compost, which serves as a substitute for artificial fertilizers. This transformation is gradual, as it necessitates time for the feedstock to decompose. From a biological perspective, composting involves a controlled aerobic process aimed at breaking down solid waste, rendering it suitable for recycling as plant nutrients, stabilizing soil, aiding in remediation processes, or altering soil composition to enhance soil regeneration. The feasibility of compost production can dictate whether it occurs at a decentralized or centralized level. Both approaches entail significant preprocessing, and composting is typically feasible only with segregated organic matter. Examples of decentralized composting methods include home composting, bin composting, box composting, and vermicomposting, while centralized methods include windrow composting, aerated static pile composting, and in-vessel composting.
Landfill: Landfilling is the process of putting food waste in heaps on land, which produces leachate, a liquid generated when water percolates through the garbage, collecting up different toxins. This leachate contains hazardous substances like organic acids and ammonium, posing risks to human health and environmental pollution. Hydrolysis, aerobic degradation, and anaerobic degradation within a confined environment contribute to leachate formation. Initially, hydrolysis, facilitated by oxygen, yields monosaccharides, fatty acids, glycerol, and amino acids. Over time, as air penetration diminishes, anaerobic degradation predominates. Once decomposition occurs, the land can be repurposed for further decomposition activities. However, landfills pose risks of groundwater contamination and contribute to land pollution. Proper treatment of leachate is crucial due to its high nitrogen and phosphorus content, which can lead to water pollution.
Incineration: Incineration entails the combustion of waste at elevated temperatures within a controlled setting. Prior to incineration, waste undergoes pre-treatment procedures like torrefaction, leaching, and bio-drying to mitigate the release of harmful substances into the environment. Pre-treatment serves to decrease moisture content, facilitating the combustion process. This method effectively diminishes the volume of food waste, converting it into ash. The high temperatures during incineration substantially reduce pests and pathogens. The controlled environment aids in preventing both land and water pollution. However, inadequate maintenance of incineration plants can lead to the emission of pollutants into the atmosphere. The resulting ash, albeit reduced in volume, may contain alkali materials and metals. Proper management of the ash is imperative to avert environmental pollution.
Several internal and external factors significantly impact the selection of FWT methods. Internal factors encompass economic feasibility and technological accessibility, while external factors include environmentally friendly technology and social acceptance. Economic factors involve costs incurred during plant operation, including initial setup expenses, staffing, and operational costs. A nation’s Gross Domestic Product (GDP) also influences FWT selection, particularly in terms of economic viability. For instance, a FWT plant with high operating expenses may struggle to thrive in a developing country. Technological accessibility relates to the maturity and ease of operation of the plant, with complex technologies demanding skilled workers. Social acceptance is crucial as local communities may resist the establishment of a plant due to perceived lifestyle changes. Opposition from the community can lead to significant challenges in operating the facility. Additionally, changes in consumer food waste behavior and supply chain dynamics impact the annual volume of food waste. Locating facilities in areas with high food waste concentration can reduce transportation costs. Environmental impact is another critical consideration, as industries generating significant pollution face resistance from both government and society. Adherence to government regulations and alignment with economic and societal interests are essential for industry acceptance. Thirteen criteria are chosen based on economic, technical, environmental, and social factors to evaluate alternative FWT methods. It is essential to select an alternative that minimizes costs while maximizing profits for successful business operations. Therefore, each criterion is categorized into cost (negative) or benefit (positive) for comprehensive evaluation of alternatives and is given in the Table 2.
5.1 Proposed Methodology Results
In this section, we have incorporated the adapted framework into the fuzzy MCDM approach using the aggregated SPOTIS-CRITIC method (Fig. 1). During the evaluation of the present alternatives, the criteria are graded as benefit (positive) and cost (negative).
Figure 1: Overall proposed algorithm
(i) The decision matrix concerning the criteria and alternatives is formulated based on BPFS, as given in step 1. In this study, four DMs have been formed based on the opinions of four experts. Equal weight is given to each decision expert in the problem, and based on the linguistic scale fixed in Table 3, the decision matrices are given in Table 4.
(ii) Based on the statistical averaging function, the given decision matrices are aggregated into a single DM, which is provided in the Table 5.
(iii) The score function specified in the definition is utilized on the aggregated decision matrix and the normalized decision matrix is provided in the Table 6.
(iv) The weights for the criteria are assigned by the application of CRITIC methods as proposed in step 3 as
(v) The correlation coefficient is found using the formula (3) and is given in the Table 7.
(vi) The standard deviation is calculated based on the formula (3), and the resulting values are:
(vii) To apply the SPOTIS method for ranking the alternatives, the bounds are set according to step 9 as follows: min = [0.10, 0.10, 0.08, 0.15, 0.13, 0.13, 0.12, 0.15, 0.11, 0.08, 0.10, 0.14, 0.13].
max = [0.13, 0.14, 0.14, 0.18, 0.19, 0.19, 0.17, 0.18, 0.14, 0.13, 0.18, 0.19, 0.20].
(viii) The normalized the averaged distance of the alternatives using the formulas (6)–(8). The normalized averaged distances for the alternatives are as follows:
(ix) Using step 13, we conclude that the alternative
Figure 2: Priority of criteria based on CRITIC approach
Figure 3: SPOTIS ranking reversal results
6 Result Validation and Discussion
The CRITIC method is utilized to analyze the influence of each criterion on the alternatives, as illustrated in Table 3. According to the table, benefit to society holds the greatest influence, closely followed by compatibility. Energy consumption also significantly impacts the evaluation of alternatives, while operation cost has the least influence. For the successful operation of a FWT plant, societal acceptance is crucial. Public concerns regarding pollution, odor, and noise arising from the presence of the FWT plant in their vicinity must be addressed before establishment to ensure facility success. Providing employment opportunities for locals can enhance community acceptance and facilitate smooth operation. Minimizing energy consumption is essential as it not only reduces costs but also mitigates environmental impact. Technologies with high operational costs may deter investors and face adoption challenges, potentially leading to failure. Among the alternatives,
Sensitivity analysis: In all MCDM problems, the significance of each criterion is determined based on expert opinion. Since the relative importance of criteria greatly influences alternative evaluation, system reliability is crucial. Assessing system robustness involves changing input data within specified conditions to test its reliability. In this study, out of the identified 13 criteria, six are cost-related and the remaining are benefit-related. Sensitivity analysis involves assigning different weights to cost and benefit criteria and adjusting parameter values. In this model, no parameters are associated with BPFS, CRITIC, and SPOTIS methods. Therefore, sensitivity analysis involves varying the weightage of benefit and cost criteria.
(a) Assuming equal significance for all criteria, each criterion (
(b) Equally weighting benefit and cost criteria at 50% each results in a slight change in alternative distances:
(c) Assigning 70% significance to beneficial criteria leads to alternative distances of:
(d) Assuming 20% and 80% importance for beneficial and non-beneficial attributes, the alternative distances are:
The CRITIC weighting approach heavily influences the alternatives’ ranking illustrated in the Fig. 4. The robustness of the proposed technique is demonstrated by observing changes in the SPOTIS result as weights assigned to each criterion are varied. Despite weight adjustments, the ranking of alternatives remains consistent. Alternative
Figure 4: Sensitivity analysis illustration
Comparative analysis: There are several MCDM methods suitable for evaluating FWT technologies. To examine both the similarities and distinctions among these methods, a comparative analysis has been conducted with various other MCDM methods. This analysis entails inputting the defined decision matrix into different methods to observe potential variations in results. Such comparisons allow us to understand how the outcomes of our proposed model may differ when evaluated using alternative methodologies.
Table 8 presents the ranking of alternatives obtained through Additive Ratio ASsessment (ARAS), Complex Proportional Assessment (COPPRAS), Evaluation based on Distance from Average Solution (EDAS), Multi-Objective Optimization based on Simple Ratio Analysis (MOOSRA), TOPSIS, VIKOR, ELECTRE methods and the proposed model. The application of these MCDM methods does not alter the ranking of alternatives, affirming the accuracy of the proposed algorithm (Fig. 5). Additionally, Spearman’s rank correlation analysis is conducted to assess the consistency between different ranking methods and the proposed model, as illustrated in Fig. 6. A correlation coefficient of 1 signifies a positive monotonic relationship, 0 denotes a neutral monotonic relationship, and −1 indicates a negative relationship. The proposed model exhibits a positive monotonic relationship with ARAS, COPRAS, MOOSRA, and TOPSIS ranking methods. Moreover, the correlation coefficient of 0.8 suggests a stronger monotonic relationship between EDAS, VIKOR, and ELECTRE methods and the proposed model, confirming the consistency of the proposed approach.
Figure 5: Comparative analysis graphical representation
Figure 6: Spearman’s rank correlation analysis graphical representation
7 Conclusion, Limitations and Future Directions
Over-shopping food items on impulse and leaving them in storage for too long is one of the reasons for household FW. Due to high population density and the dining-out culture among the youth, the number of restaurants has grown significantly in urban areas. Based on taste and price, FW occurs in restaurants worldwide [69]. This produces higher concentrations of FW in cities than in rural counterparts. The inefficiency in the supply chain in the agricultural sector is one of the contributors to FW. Huge quantities of agricultural produce are wasted during the post-harvest stage. These FW account for the carbon footprint due to the emission of carbon dioxide and other harmful gases [70]. Hence, various studies have been conducted to evaluate the treatment technologies of the FW. This study considers four different technologies and evaluates them based on the hybrid CRITIC-TOPSIS approach. CRITIC is employed to assign weights to the attributes, while SPOTIS is applied to evaluate and rank the alternatives. Applying the CRITIC method is vital for evaluating the importance of each criterion. In the CRITIC method, the criterion
There are a few limitations that are yet to be overcome. Since the data on FW is extremely fluctuating, only an approximation of the exact data could be found. This study only examined four extensively used technologies for evaluating the optimal technique. In the future, the study could be extended to the new FWT techniques. By focusing on smaller regions, the data accuracy could be guaranteed, and the criteria for the FWT selection could be tailored based on the requirements and preferences in that region. A combination of subjective and objective weighting methods can be employed to analyze the given situation. Due to the reliability, robustness, and rank reversal-free nature of the proposed CRITIC-SPOTIS method, it can be applied to various other technology selections in pharmaceutical, electronics, information technology, Industries, metallurgy, etc. This method could also be extended for site selection to small-scale and large-scale industries. In the future, interpretability analysis can be performed for the proposed model to ensure the transparency of the proposed model. Performing Interpretability analysis can help to facilitate the adoption of the proposed method in real-world scenarios. Statistical averaging methods can accommodate various types of uncertainty beyond fuzzy logic, such as probabilistic uncertainty, measurement error, or variability. It provides simple calculations and transparent results. It significantly reduces the calculation time while the fuzzy aggregation operators increase the complexity of the proposed model. Hence, the statistical averaging model is preferred to fuzzy averaging operators. In the future, the same study could be extended by implementing the aggregation operator instead of the averaging operator.
Acknowledgement: This work was supported by the Department of Mathematics, Bharathiar University, Coimbatore, India.
Funding Statement: The authors received no specific funding for this study.
Author Contributions: Navaneethakrishnan Suganthi Keerthana Devi: Conceptualization, Data curation, Methodology, Writing-original draft, Writing, Review and Editing. Samayan Narayanamoorthy: Conceptualization, Data curation, Formal analysis, Supervision, Investigation, Methodology, Validation, Visualization, Writing-original draft, Writing and Editing. Thirumalai Nallasivan Parthasarathy: Resources, Software, Validation, Visualization, Writing and Editing. Chakkarapani Sumathi Thilagashree: Validation, Resources, Writing and Editing. Dragan Pamucar: Validation, Writing and Editing. Vladimir Simic: Validation, Visualization, and Editing. Ali Hasan Dinçer: Validation, Visualization, and Editing. Serhat Yüksel: Validation, Visualization, and Editing.
Availability of Data and Materials: The study utilizes secondary data, which is available with the authors and can be made available upon public request.
Conflicts of Interest: The authors declare that they have no conflicts of interest to report regarding the present study.
References
1. d’Amour CB, Pandey B, Reba M, Ahmad S, Creutzig F, Seto KC. Urbanization, processed foods, and eating out in India. Glob Food Secur. 2020;25:100361. doi:10.1016/j.gfs.2020.100361. [Google Scholar] [CrossRef]
2. Liu C, Shang J, Liu C, Wang H, Wang S. Policy recommendations for reducing food waste: an analysis based on a survey of urban and rural household food waste in Harbin, China. Sustainability. 2023;15(14):11122. doi:10.3390/su151411122. [Google Scholar] [CrossRef]
3. Ogunmoroti A, Liu M, Li M, Liu W. Unraveling the environmental impact of current and future food waste and its management in Chinese provinces. Resour Environ Sustain. 2022;9:100064. [Google Scholar]
4. Parsa A, van de Wiel M, Schmutz U, Fried J, Black D, Roderick I. Challenging the food waste hierarchy. J Environ Manag. 2023;344:118554. doi:10.1016/j.jenvman.2023.118554. [Google Scholar] [PubMed] [CrossRef]
5. Das A, Verma M, Mishra V. Food waste to resource recovery: a way of green advocacy. Environ Sci Pollut Res. 2024;31(12):17874–86. [Google Scholar]
6. Paranjpe A, Saxena S, Jain P. A review on performance improvement of anaerobic digestion using co-digestion of food waste and sewage sludge. J Environ Manag. 2023;338:117733. doi:10.1016/j.jenvman.2023.117733. [Google Scholar] [PubMed] [CrossRef]
7. Xiong S, Liu Y, Zhang H, Xu S, Li S, Fan X, et al. Effects of chemical additives and mature compost on reducing nitrogen loss during food waste composting. Environ Sci Pollut Res. 2023;30(13):39000–11. doi:10.1007/s11356-022-24752-5. [Google Scholar] [PubMed] [CrossRef]
8. Roy P, Mohanty AK, Dick P, Misra M. A review on the challenges and choices for food waste valorization: environmental and economic impacts. ACS Environ Au. 2023;3(2):58–75. doi:10.1021/acsenvironau.2c00050. [Google Scholar] [PubMed] [CrossRef]
9. Bhatia L, Jha H, Sarkar T, Sarangi PK. Food waste utilization for reducing carbon footprints towards sustainable and cleaner environment: a review. Int J Environ Res Public Health. 2023;20(3):2318. doi:10.3390/ijerph20032318. [Google Scholar] [PubMed] [CrossRef]
10. Fei F, Wen Z, Zhang J, Xing Y, Zhang H, Li Y. New multi-source waste co-incineration and clustering park operating model for small-and medium-sized city: a case study in China. J Environ Manag. 2024;355:120514. doi:10.1016/j.jenvman.2024.120514. [Google Scholar] [PubMed] [CrossRef]
11. Garcia-Garcia G, Woolley E, Rahimifard S, Colwill J, White R, Needham L. A methodology for sustainable management of food waste. Waste Biomass Valorization. 2017;8(6):2209–27. doi:10.1007/s12649-016-9720-0. [Google Scholar] [PubMed] [CrossRef]
12. Sakcharoen T, Ratanatamskul C, Chandrachai A. Factors affecting technology selection, techno-economic and environmental sustainability assessment of a novel zero-waste system for food waste and wastewater management. J Clean Prod. 2021;314:128103. doi:10.1016/j.jclepro.2021.128103. [Google Scholar] [CrossRef]
13. Buyuk AM, Temur GT. Food waste treatment option selection through spherical fuzzy AHP. J Intell Fuzzy Syst. 2022;42(1):97–107. [Google Scholar]
14. Bernstad A, la Cour Jansen J. A life cycle approach to the management of household food wasteaa Swedish full-scale case study. Waste Manag. 2011;31(8):1879–96. doi:10.1016/j.wasman.2011.02.026. [Google Scholar] [PubMed] [CrossRef]
15. Angelo AC, Saraiva AB, Clímaco JC, Infante CE, Valle R. Life cycle assessment and multi-criteria decision analysis: selection of a strategy for domestic food waste management in Rio de Janeiro. J Clean Prod. 2017;143:744–56. doi:10.1016/j.jclepro.2016.12.049. [Google Scholar] [CrossRef]
16. Liu KM, Lin SH, Hsieh JC, Tzeng GH. Improving the food waste composting facilities site selection for sustainable development using a hybrid modified MADM model. Waste Manag. 2018;75:44–59. doi:10.1016/j.wasman.2018.02.017. [Google Scholar] [PubMed] [CrossRef]
17. Gerassimidou S, Dora M, Iacovidou E. A tool for the selection of food waste management approaches for the hospitality and food service sector in the UK. Resources. 2022;11(10):80. doi:10.3390/resources11100080. [Google Scholar] [CrossRef]
18. Gao A, Tian Z, Wang Z, Wennersten R, Sun Q. Comparison between the technologies for food waste treatment. Energy Proc. 2017;105:3915–21. doi:10.1016/j.egypro.2017.03.811. [Google Scholar] [CrossRef]
19. Rani P, Mishra AR, Krishankumar R, Ravichandran KS, Kar S. Multi-criteria food waste treatment method selection using single-valued neutrosophic-CRITIC-MULTIMOORA framework. Appl Soft Comput. 2021;111:107657. doi:10.1016/j.asoc.2021.107657. [Google Scholar] [CrossRef]
20. Rani P, Mishra AR, Saha A, Hezam IM, Pamucar D. Fermatean fuzzy Heronian mean operators and MEREC-based additive ratio assessment method: an application to food waste treatment technology selection. Int J Intell Syst. 2022;37(3):2612–47. doi:10.1002/int.v37.3. [Google Scholar] [CrossRef]
21. Zadeh LA. Fuzzy sets. Inf Control. 1965;8(3):338–53. doi:10.1016/S0019-9958(65)90241-X. [Google Scholar] [CrossRef]
22. Mendel JM. Type-2 fuzzy sets and systems: an overview. IEEE Comput Intell Mag. 2007;2(1):20–9. doi:10.1109/MCI.2007.380672. [Google Scholar] [CrossRef]
23. Ali S, Naveed H, Siddique I, Zulqarnain RM. Extension of Interaction geometric aggregation operator for material selection using interval-valued intuitionistic fuzzy hypersoft set. J Oper Intell. 2024;2(1):14–35. doi:10.31181/jopi21202410. [Google Scholar] [CrossRef]
24. Gorzałczany MB. An interval-valued fuzzy inference methodasome basic properties. Fuzzy Sets Syst. 1989;31(2):243–51. doi:10.1016/0165-0114(89)90006-7. [Google Scholar] [CrossRef]
25. Ali A, Ullah K, Hussain A. An approach to multi-attribute decision-making based on intuitionistic fuzzy soft information and Aczel-Alsina operational laws. J Decis Anal Intell Comput. 2023;3(1):80–9. doi:10.31181/jdaic180601p. [Google Scholar] [CrossRef]
26. De SK, Biswas R, Roy AR. Some operations on intuitionistic fuzzy sets. Fuzzy Sets Syst. 2000;114(3):477–84. doi:10.1016/S0165-0114(98)00191-2. [Google Scholar] [CrossRef]
27. Wang P, Zhu B, Yu Y, Ali Z, Almohsen B. Complex intuitionistic fuzzy DOMBI prioritized aggregation operators and their application for resilient green supplier selection. Facta Univ Sers: Mech Eng. 2023;21(3):339–57. doi:10.22190/FUME230805029W. [Google Scholar] [CrossRef]
28. Farooq D. Application of pythagorean fuzzy analytic hierarchy process for assessing driver behavior criteria associated to road safety. J Soft Comput Decis Anal. 2024;2(1):144–58. doi:10.31181/jscda21202439. [Google Scholar] [CrossRef]
29. Julong D. Introduction to grey system theory. J Grey Syst. 1989;1(1):1–24. [Google Scholar]
30. Akram M, Shumaiza, Arshad M. Bipolar fuzzy TOPSIS and bipolar fuzzy ELECTRE-I methods to diagnosis. Comput Appl Math. 2020;39(1):1–21. doi:10.1007/s40314-019-0980-8. [Google Scholar] [CrossRef]
31. Wang H, Smarandache F, Zhang Y, Sunderraman R. Single valued neutrosophic sets. Inf Study. 2010;12:20110. [Google Scholar]
32. Barman H, Roy SK, Sakalauskas L, Weber GW. Inventory model involving reworking of faulty products with three carbon policies under neutrosophic environment. Adv Eng Inform. 2023;57(3):102081. doi:10.1016/j.aei.2023.102081. [Google Scholar] [CrossRef]
33. Fatima A, Ashraf S, Jana C. Approach to multi-attribute decision making based on spherical fuzzy einstein Z-number aggregation information. J Oper Intell. 2024;2(1):179–201. doi:10.31181/jopi21202411. [Google Scholar] [CrossRef]
34. Sarfraz M. Multi-attribute decision-making for T-spherical fuzzy information utilizing schweizer-sklar prioritized aggregation operators for recycled water. Decis Mak Adv. 2024;2(1):105–28. doi:10.31181/dma21202425. [Google Scholar] [CrossRef]
35. Cuong BC, Kreinovich V. Picture fuzzy sets. J Comput Sci Cybern. 2014;30(4):409–20. [Google Scholar]
36. Parthasarathy TN, Narayanamoorthy S, Devi NS, Pamucar D, Simic V, Kang D. An idiosyncratic interval valued picture q-rung orthopair fuzzy decision-making model for electric vehicle battery charging technology selection. Int J Fuzzy Syst. 2024;21:1–6. [Google Scholar]
37. Riaz M, Garg H, Athar Farid HM, Chinram R. Multi-criteria decision making based on bipolar picture fuzzy operators and new distance measures. Comput Model Eng Sci. 2021;127(2):771–800. doi:10.32604/cmes.2021.014174. [Google Scholar] [CrossRef]
38. García-Cascales MS, Lamata MT. On rank reversal and TOPSIS method. Math Comput Model. 2012;56(5–6):123–32. [Google Scholar]
39. Asadabadi MR, Chang E, Saberi M. Are MCDM methods useful? A critical review of analytic hierarchy process (AHP) and analytic network process (ANP). Cogent Eng. 2019;6(1):1623153. doi:10.1080/23311916.2019.1623153. [Google Scholar] [CrossRef]
40. Opricovic S, Tzeng GH. Compromise solution by MCDM methods: a comparative analysis of VIKOR and TOPSIS. Eur J Oper Res. 2004;156(2):445–55. doi:10.1016/S0377-2217(03)00020-1. [Google Scholar] [CrossRef]
41. Ghosh S, Roy SK. Closed-loop multi-objective waste management through vehicle routing problem in neutrosophic hesitant fuzzy environment. Appl Soft Comput. 2023;148:110854. doi:10.1016/j.asoc.2023.110854. [Google Scholar] [CrossRef]
42. Çalı S, Balaman Ş.Y. A novel outranking based multi criteria group decision making methodology integrating ELECTRE and VIKOR under intuitionistic fuzzy environment. Expert Syst Appl. 2019;119:36–50. doi:10.1016/j.eswa.2018.10.039. [Google Scholar] [CrossRef]
43. Dağıstanlı HA. An interval-valued intuitionistic fuzzy VIKOR approach for R&D project selection in defense industry investment decisions. J Soft Comput Decis Anal. 2024;2(1):1–3. [Google Scholar]
44. Brans JP, Vincke P, Mareschal B. How to select and how to rank projects: the PROMETHEE method. Eur J Oper Res. 1986;24(2):228–38. doi:10.1016/0377-2217(86)90044-5. [Google Scholar] [CrossRef]
45. Dezert J, Tchamova A, Han D, Tacnet JM. The SPOTIS rank reversal free method for multi-criteria decision-making support. In: 2020 IEEE 23rd International Conference on Information Fusion (FUSION); 2020 Jul 6; Rustenburg, South Africa, IEEE. p. 1–8. [Google Scholar]
46. Paradowski B, BAczkiewicz A, WatrAbski J. Towards proper consumer choices-MCDM based product selection. Procedia Comput Sci. 2021;192:1347–58. doi:10.1016/j.procs.2021.08.138. [Google Scholar] [CrossRef]
47. Shekhovtsov A, Paradowski B, Wieckowski J, Kizielewicz B, Sałabun W. Extension of the SPOTIS method for the rank reversal free decision-making under fuzzy environment. In: 2022 IEEE 61st Conference on Decision and Control (CDC); 2022 Dec 6; Cancun, Mexico, IEEE. p. 5595–600. [Google Scholar]
48. de Azevêdo Junior CM, Júnior EL, de Castro Araujo TM, dos Santos M, Gomes CF, de Moura Pereira DA. Ordering of solar photovoltaic panels using the MEREC-SPOTIS hybrid analytical model. Procedia Comput Sci. 2023;230:808–18. doi:10.1016/j.procs.2023.12.052. [Google Scholar] [CrossRef]
49. Wieckowski J, Król R, Watróbski J. Towards robust results in multi-criteria decision analysis: ranking reversal free methods case study. Procedia Comput Sci. 2022;207:4584–92. doi:10.1016/j.procs.2022.09.522. [Google Scholar] [CrossRef]
50. Baczkiewicz A, Kizielewicz B, Shekhovtsov A, Yelmikheiev M, Kozlov V, Sałabun W. Comparative analysis of solar panels with determination of local significance levels of criteria using the MCDM methods resistant to the rank reversal phenomenon. Energies. 2021;14(18):5727. doi:10.3390/en14185727. [Google Scholar] [CrossRef]
51. Ayan B, Abacıoğlu S, Basilio MP. A comprehensive review of the novel weighting methods for multi-criteria decision-making. Information. 2023;14(5):285. doi:10.3390/info14050285. [Google Scholar] [CrossRef]
52. Hovanov NV, Kolari JW, Sokolov MV. Deriving weights from general pairwise comparison matrices. Math Soc Sci. 2008;55(2):205–20. doi:10.1016/j.mathsocsci.2007.07.006. [Google Scholar] [CrossRef]
53. Karabašević D, Stanujkić D, Urošević S. The MCDM model for personnel selection based on SWARA and ARAS methods. Management. 2015;20(77):43–52. [Google Scholar]
54. Saaty TL. How to make a decision: the analytic hierarchy process. Eur J Oper Res. 1990;48(1):9–26. doi:10.1016/0377-2217(90)90057-I. [Google Scholar] [CrossRef]
55. Yang JL, Tzeng GH. An integrated MCDM technique combined with DEMATEL for a novel cluster-weighted with ANP method. Expert Syst Appl. 2011;38(3):1417–24. doi:10.1016/j.eswa.2010.07.048. [Google Scholar] [CrossRef]
56. Baykasoğlu A, Kaplanoğlu V, Durmuşoğlu ZD, Şahin C. Integrating fuzzy DEMATEL and fuzzy hierarchical TOPSIS methods for truck selection. Expert Syst Appl. 2013;40(3):899–907. doi:10.1016/j.eswa.2012.05.046. [Google Scholar] [CrossRef]
57. Rezaei J. Best-worst multi-criteria decision-making method. Omega. 2015;53:49–57. doi:10.1016/j.omega.2014.11.009. [Google Scholar] [CrossRef]
58. Krishnan AR, Kasim MM, Hamid R, Ghazali MF. A modified CRITIC method to estimate the objective weights of decision criteria. Symmetry. 2021;13(6):973. doi:10.3390/sym13060973. [Google Scholar] [CrossRef]
59. Garg H, Agarwal N, Tripathi A. Entropy based multi-criteria decision making method under fuzzy environment and unknown attribute weights. Glob J Technol Optim. 2015;6(3):13–20. [Google Scholar]
60. Diakoulaki D, Mavrotas G, Papayannakis L. Determining objective weights in multiple criteria problems: the critic method. Comput Oper Res. 1995;22(7):763–70. doi:10.1016/0305-0548(94)00059-H. [Google Scholar] [CrossRef]
61. Hashemkhani Zolfani S, Yazdani M, Ebadi Torkayesh A, Derakhti A. Application of a gray-based decision support framework for location selection of a temporary hospital during COVID-19 pandemic. Symmetry. 2020;12(6):886. doi:10.3390/sym12060886. [Google Scholar] [CrossRef]
62. Zavadskas EK, Podvezko V. Integrated determination of objective criteria weights in MCDM. Int J Inf Technol Decis Making. 2016;15(02):267–83. doi:10.1142/S0219622016500036. [Google Scholar] [CrossRef]
63. Narayanamoorthy S, Parthasarathy TN, Pragathi S, Shanmugam P, Baleanu D, Ahmadian A, et al. The novel augmented fermatean MCDM perspectives for identifying the optimal renewable energy power plant location. Sustain Energy Technol Assess. 2022;53(2):102488. doi:10.1016/j.seta.2022.102488. [Google Scholar] [CrossRef]
64. Naz S, Shafiq A, Butt SA. CILOS-WASPAS approach based on Schweizer-Sklar power operators for evaluating cosmetic brands in a group decision-making environment. Granul Comput. 2024;9(3):1–37. doi:10.1007/s41066-024-00481-7. [Google Scholar] [CrossRef]
65. Kahraman C, Onar SC, Öztayşi B. A novel spherical fuzzy CRITIC method and its application to prioritization of supplier selection criteria. J Intell Fuzzy Syst. 2022;42(1):29–36. doi:10.3233/JIFS-219172. [Google Scholar] [CrossRef]
66. Vujičić MD, Papić MZ, Blagojević MD. Comparative analysis of objective techniques for criteria weighing in two MCDM methods on example of an air conditioner selection. Tehnika. 2017;72(3):422–9. doi:10.5937/tehnika1703422V. [Google Scholar] [CrossRef]
67. Zhang F, Wang P, Mu P, Wang M, Han L, Sun J. A comprehensive evaluation method for the service status of groins in waterways based on an AHP-improved CRITIC combination weighting optimization model. Sustainability. 2022;14(17):10709. doi:10.3390/su141710709. [Google Scholar] [CrossRef]
68. Parthasarathy TN, Narayanamoorthy S, Sulaiman R, Elamir AM, Ahmadian A, Kang D. An end-to-end categorizing strategy for green energy sources: picture q-rung orthopair fuzzy EXPROM-II: mADA approach. Sustain Energy Technol Assess. 2024;63:103658. [Google Scholar]
69. Coşkun A, Özbük RM. What influences consumer food waste behavior in restaurants? An application of the extended theory of planned behavior. Waste Manag. 2020;117:170–8. doi:10.1016/j.wasman.2020.08.011. [Google Scholar] [PubMed] [CrossRef]
70. Chen C, Chaudhary A, Mathys A. Nutritional and environmental losses embedded in global food waste. Resour Conservat Recycl. 2020;160:104912. doi:10.1016/j.resconrec.2020.104912. [Google Scholar] [CrossRef]
Cite This Article
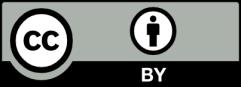