Open Access
ARTICLE
AFBNet: A Lightweight Adaptive Feature Fusion Module for Super-Resolution Algorithms
1 Department of Geography and Anthropology, Louisiana State University, Baton Rouge, LA, 70803, USA
2 School of Automation, University of Electronic Science and Technology of China, Chengdu, 610054, China
3 College of Computer and Information Sciences, King Saud University, Riyadh, 11574, Saudi Arabia
4 College of Resource and Environment Engineering, Guizhou University, Guiyang, 550025, China
5 School of Geographical Sciences, Southwest University, Chongqing, 400715, China
* Corresponding Authors: Siyu Lu. Email: ; Wenfeng Zheng. Email:
Computer Modeling in Engineering & Sciences 2024, 140(3), 2315-2347. https://doi.org/10.32604/cmes.2024.050853
Received 20 February 2024; Accepted 03 April 2024; Issue published 08 July 2024
Abstract
At present, super-resolution algorithms are employed to tackle the challenge of low image resolution, but it is difficult to extract differentiated feature details based on various inputs, resulting in poor generalization ability. Given this situation, this study first analyzes the features of some feature extraction modules of the current super-resolution algorithm and then proposes an adaptive feature fusion block (AFB) for feature extraction. This module mainly comprises dynamic convolution, attention mechanism, and pixel-based gating mechanism. Combined with dynamic convolution with scale information, the network can extract more differentiated feature information. The introduction of a channel spatial attention mechanism combined with multi-feature fusion further enables the network to retain more important feature information. Dynamic convolution and pixel-based gating mechanisms enhance the module’s adaptability. Finally, a comparative experiment of a super-resolution algorithm based on the AFB module is designed to substantiate the efficiency of the AFB module. The results revealed that the network combined with the AFB module has stronger generalization ability and expression ability.Keywords
Cite This Article
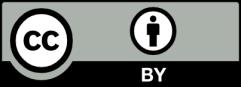
This work is licensed under a Creative Commons Attribution 4.0 International License , which permits unrestricted use, distribution, and reproduction in any medium, provided the original work is properly cited.