Open Access
ARTICLE
LSTM Based Neural Network Model for Anomaly Event Detection in Care-Independent Smart Homes
1 Department of Computer Science and Information Engineering, Asia University, Taichung, 413, Taiwan
2 Symbiosis Centre for Information Technology (SCIT), Symbiosis International University, Pune, 411057, India
3 Center for Interdisciplinary Research, University of Petroleum and Energy Studies (UPES), Dehradun, 248007, India
4 Computer Engineering, Ronin Institute, Montclair, NJ 07043, USA
5 Management Department, College of Business Administration, Princess Nourah bint Abdulrahman University, P.O. Box 84428, Riyadh, 11671, Saudi Arabia
6 Department of Business Administration, Asia University, Taichung, 413, Taiwan
7 Department of Electrical and Computer Engineering, Lebanese American University, Beirut, 1102, Lebanon
8 Department of Computer Sciences, Faculty of Computing and Information Technology, Northern Border University, Rafha, 91911, Saudi Arabia
9 School of Science and Technology, Hong Kong Metropolitan University (HKMU), Hong Kong, China
* Corresponding Author: Brij B. Gupta. Email:
(This article belongs to the Special Issue: Control Systems and Machine Learning for Intelligent Computing)
Computer Modeling in Engineering & Sciences 2024, 140(3), 2689-2706. https://doi.org/10.32604/cmes.2024.050825
Received 19 February 2024; Accepted 16 May 2024; Issue published 08 July 2024
Abstract
This study introduces a long-short-term memory (LSTM)-based neural network model developed for detecting anomaly events in care-independent smart homes, focusing on the critical application of elderly fall detection. It balances the dataset using the Synthetic Minority Over-sampling Technique (SMOTE), effectively neutralizing bias to address the challenge of unbalanced datasets prevalent in time-series classification tasks. The proposed LSTM model is trained on the enriched dataset, capturing the temporal dependencies essential for anomaly recognition. The model demonstrated a significant improvement in anomaly detection, with an accuracy of 84%. The results, detailed in the comprehensive classification and confusion matrices, showed the model’s proficiency in distinguishing between normal activities and falls. This study contributes to the advancement of smart home safety, presenting a robust framework for real-time anomaly monitoring.Keywords
Cite This Article
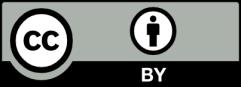
This work is licensed under a Creative Commons Attribution 4.0 International License , which permits unrestricted use, distribution, and reproduction in any medium, provided the original work is properly cited.