Open Access
ARTICLE
FDSC-YOLOv8: Advancements in Automated Crack Identification for Enhanced Safety in Underground Engineering
1 Key Laboratory of Computing Power Network and Information Security, Ministry of Education, Shandong Computer Science Center (National Supercomputer Center in Jinan), Qilu University of Technology (Shandong Academy of Sciences), Jinan, 250353, China
2 School of Qilu Transportation, Shandong University, Jinan, 250100, China
3 Geotechnical and Structural Engineering Center, Shandong University, Jinan, 250061, China
4 Shandong Lairong High-Speed Railway Co., Ltd., Weihai, 264200, China
5 Shandong High-Speed Group Co., Ltd., Jinan, 250014, China
* Corresponding Author: Zhihui Liu. Email:
(This article belongs to the Special Issue: Multiscale, Multifield, and Continuum-Discontinuum Analysis in Geomechanics )
Computer Modeling in Engineering & Sciences 2024, 140(3), 3035-3049. https://doi.org/10.32604/cmes.2024.050806
Received 19 February 2024; Accepted 24 April 2024; Issue published 08 July 2024
Abstract
In underground engineering, the detection of structural cracks on tunnel surfaces stands as a pivotal task in ensuring the health and reliability of tunnel structures. However, the dim and dusty environment inherent to underground engineering poses considerable challenges to crack segmentation. This paper proposes a crack segmentation algorithm termed as Focused Detection for Subsurface Cracks YOLOv8 (FDSC-YOLOv8) specifically designed for underground engineering structural surfaces. Firstly, to improve the extraction of multi-layer convolutional features, the fixed convolutional module is replaced with a deformable convolutional module. Secondly, the model’s receptive field is enhanced by introducing a multi-branch convolutional module, improving the extraction of shallow features for small targets. Next, the Dynamic Snake Convolution module is incorporated to enhance the extraction capability for slender and weak cracks. Finally, the Convolutional Block Attention Module (CBAM) module is employed to achieve better target determination. The FDSC-YOLOv8s algorithm’s mAP50 and mAP50-95 reach 96.5% and 66.4%, according to the testing data.Keywords
Cite This Article
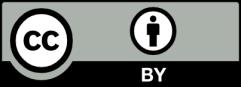