Open Access
ARTICLE
GliomaCNN: An Effective Lightweight CNN Model in Assessment of Classifying Brain Tumor from Magnetic Resonance Images Using Explainable AI
1 Department of Computer Science and Engineering, Ahsanullah University of Science and Technology, Dhaka, 1208, Bangladesh
2 Department of Computer Science and Software Engineering, The University of Western Australia, Perth, WA 6009, Australia
3 Department of Computer Science, American International University-Bangladesh, Dhaka, 1229, Bangladesh
4 Department of Computer Science, College of Computer and Information Sciences, King Saud University, P.O.Box 51178, Riyadh, 11543, Saudi Arabia
5 School of Computing, Southern Illinois University, Carbondale, 62901, USA
* Corresponding Authors: M. F. Mridha. Email: ; Mejdl Safran. Email:
Computer Modeling in Engineering & Sciences 2024, 140(3), 2425-2448. https://doi.org/10.32604/cmes.2024.050760
Received 16 February 2024; Accepted 23 April 2024; Issue published 08 July 2024
Abstract
Brain tumors pose a significant threat to human lives and have gained increasing attention as the tenth leading cause of global mortality. This study addresses the pressing issue of brain tumor classification using Magnetic resonance imaging (MRI). It focuses on distinguishing between Low-Grade Gliomas (LGG) and High-Grade Gliomas (HGG). LGGs are benign and typically manageable with surgical resection, while HGGs are malignant and more aggressive. The research introduces an innovative custom convolutional neural network (CNN) model, Glioma-CNN. GliomaCNN stands out as a lightweight CNN model compared to its predecessors. The research utilized the BraTS 2020 dataset for its experiments. Integrated with the gradient-boosting algorithm, GliomaCNN has achieved an impressive accuracy of 99.1569%. The model’s interpretability is ensured through SHapley Additive exPlanations (SHAP) and Gradient-weighted Class Activation Mapping (Grad-CAM++). They provide insights into critical decision-making regions for classification outcomes. Despite challenges in identifying tumors in images without visible signs, the model demonstrates remarkable performance in this critical medical application, offering a promising tool for accurate brain tumor diagnosis which paves the way for enhanced early detection and treatment of brain tumors.Keywords
Cite This Article
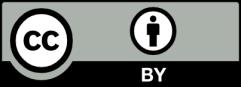