Open Access
ARTICLE
Evaluations of Chris-Jerry Data Using Generalized Progressive Hybrid Strategy and Its Engineering Applications
1 Department of Mathematical Sciences, College of Science, Princess Nourah bint Abdulrahman University, P.O. Box 84428, Riyadh, 11671, Saudi Arabia
2 Department of Statistics, Al-Azhar University, Cairo, 11884, Egypt
3 Faculty of Technology and Development, Zagazig University, Zagazig, 44519, Egypt
* Corresponding Author: Ahmed Elshahhat. Email:
(This article belongs to the Special Issue: Incomplete Data Test, Analysis and Fusion Under Complex Environments)
Computer Modeling in Engineering & Sciences 2024, 140(3), 3073-3103. https://doi.org/10.32604/cmes.2024.050606
Received 12 February 2024; Accepted 28 March 2024; Issue published 08 July 2024
Abstract
A new one-parameter Chris-Jerry distribution, created by mixing exponential and gamma distributions, is discussed in this article in the presence of incomplete lifetime data. We examine a novel generalized progressively hybrid censoring technique that ensures the experiment ends at a predefined period when the model of the test participants has a Chris-Jerry (CJ) distribution. When the indicated censored data is present, Bayes and likelihood estimations are used to explore the CJ parameter and reliability indices, including the hazard rate and reliability functions. We acquire the estimated asymptotic and credible confidence intervals of each unknown quantity. Additionally, via the squared-error loss, the Bayes’ estimators are obtained using gamma prior. The Bayes estimators cannot be expressed theoretically since the likelihood density is created in a complex manner; nonetheless, Markov-chain Monte Carlo techniques can be used to evaluate them. The effectiveness of the investigated estimations is assessed, and some recommendations are given using Monte Carlo results. Ultimately, an analysis of two engineering applications, such as mechanical equipment and ball bearing data sets, shows the applicability of the proposed approaches that may be used in real-world settings.Keywords
Cite This Article
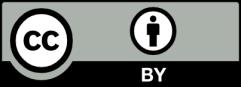