Open Access
ARTICLE
Integrating Neighborhood Geographic Distribution and Social Structure Influence for Social Media User Geolocation
1 Key Laboratory of Cyberspace Situation Awareness of Henan Province, Zhengzhou, 450001, China
2 State Key Laboratory of Mathematical Engineering and Advanced Computing, Zhengzhou, 450001, China
* Corresponding Author: Xiangyang Luo. Email:
Computer Modeling in Engineering & Sciences 2024, 140(3), 2513-2532. https://doi.org/10.32604/cmes.2024.050517
Received 08 February 2024; Accepted 30 April 2024; Issue published 08 July 2024
Abstract
Geolocating social media users aims to discover the real geographical locations of users from their publicly available data, which can support online location-based applications such as disaster alerts and local content recommendations. Social relationship-based methods represent a classical approach for geolocating social media. However, geographically proximate relationships are sparse and challenging to discern within social networks, thereby affecting the accuracy of user geolocation. To address this challenge, we propose user geolocation methods that integrate neighborhood geographical distribution and social structure influence (NGSI) to improve geolocation accuracy. Firstly, we propose a method for evaluating the homophily of locations based on the -order neighborhood geographic distribution (-NGD) similarity among users. There are notable differences in the distribution of -NGD similarity between location-proximate and non-location-proximate users. Exploiting this distinction, we filter out non-location-proximate social relationships to enhance location homophily in the social network. To better utilize the location-proximate relationships in social networks, we propose a graph neural network algorithm based on the social structure influence. The algorithm enables us to perform a weighted aggregation of the information of users’ multi-hop neighborhood, thereby mitigating the over-smoothing problem of user features and improving user geolocation performance. Experimental results on real social media dataset demonstrate that the neighborhood geographical distribution similarity metric can effectively filter out non-location-proximate social relationships. Moreover, compared with 7 existing social relationship-based user positioning methods, our proposed method can achieve multi-granularity user geolocation and improve the accuracy by 4.84% to 13.28%.Keywords
Cite This Article
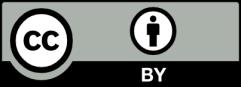
This work is licensed under a Creative Commons Attribution 4.0 International License , which permits unrestricted use, distribution, and reproduction in any medium, provided the original work is properly cited.