Open Access
REVIEW
A Comprehensive Systematic Review: Advancements in Skin Cancer Classification and Segmentation Using the ISIC Dataset
1 Department of Computer Sciences, Pakistan Institute of Engineering and Applied Sciences, Nilore, 50700, Islamabad
2 Future Technology Research Center, National Yunlin University of Science and Technology, Douliou, 64002, Taiwan
3 Directorate of IT Services, University of Gujrat, Gujrat, 50700, Pakistan
* Corresponding Author: Aneela Zameer. Email:
Computer Modeling in Engineering & Sciences 2024, 140(3), 2131-2164. https://doi.org/10.32604/cmes.2024.050124
Received 28 January 2024; Accepted 26 April 2024; Issue published 08 July 2024
Abstract
The International Skin Imaging Collaboration (ISIC) datasets are pivotal resources for researchers in machine learning for medical image analysis, especially in skin cancer detection. These datasets contain tens of thousands of dermoscopic photographs, each accompanied by gold-standard lesion diagnosis metadata. Annual challenges associated with ISIC datasets have spurred significant advancements, with research papers reporting metrics surpassing those of human experts. Skin cancers are categorized into melanoma and non-melanoma types, with melanoma posing a greater threat due to its rapid potential for metastasis if left untreated. This paper aims to address challenges in skin cancer detection via visual inspection and manual examination of skin lesion images, processes historically known for their laboriousness. Despite notable advancements in machine learning and deep learning models, persistent challenges remain, largely due to the intricate nature of skin lesion images. We review research on convolutional neural networks (CNNs) in skin cancer classification and segmentation, identifying issues like data duplication and augmentation problems. We explore the efficacy of Vision Transformers (ViTs) in overcoming these challenges within ISIC dataset processing. ViTs leverage their capabilities to capture both global and local relationships within images, reducing data duplication and enhancing model generalization. Additionally, ViTs alleviate augmentation issues by effectively leveraging original data. Through a thorough examination of ViT-based methodologies, we illustrate their pivotal role in enhancing ISIC image classification and segmentation. This study offers valuable insights for researchers and practitioners looking to utilize ViTs for improved analysis of dermatological images. Furthermore, this paper emphasizes the crucial role of mathematical and computational modeling processes in advancing skin cancer detection methodologies, highlighting their significance in improving algorithmic performance and interpretability.Keywords
Cite This Article
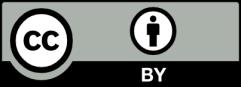
This work is licensed under a Creative Commons Attribution 4.0 International License , which permits unrestricted use, distribution, and reproduction in any medium, provided the original work is properly cited.