Open Access
ARTICLE
Advancements in Remote Sensing Image Dehazing: Introducing URA-Net with Multi-Scale Dense Feature Fusion Clusters and Gated Jump Connection
1 School of Computer, Jiangsu University of Science and Technology, Zhenjiang, 212003, China
2 Department of Electrical and Computer Engineering, University of Nevada, Las Vegas, 89119, USA
* Corresponding Author: Xing Deng. Email:
Computer Modeling in Engineering & Sciences 2024, 140(3), 2397-2424. https://doi.org/10.32604/cmes.2024.049737
Received 16 January 2024; Accepted 16 April 2024; Issue published 08 July 2024
Abstract
The degradation of optical remote sensing images due to atmospheric haze poses a significant obstacle, profoundly impeding their effective utilization across various domains. Dehazing methodologies have emerged as pivotal components of image preprocessing, fostering an improvement in the quality of remote sensing imagery. This enhancement renders remote sensing data more indispensable, thereby enhancing the accuracy of target identification. Conventional defogging techniques based on simplistic atmospheric degradation models have proven inadequate for mitigating non-uniform haze within remotely sensed images. In response to this challenge, a novel UNet Residual Attention Network (URA-Net) is proposed. This paradigmatic approach materializes as an end-to-end convolutional neural network distinguished by its utilization of multi-scale dense feature fusion clusters and gated jump connections. The essence of our methodology lies in local feature fusion within dense residual clusters, enabling the extraction of pertinent features from both preceding and current local data, depending on contextual demands. The intelligently orchestrated gated structures facilitate the propagation of these features to the decoder, resulting in superior outcomes in haze removal. Empirical validation through a plethora of experiments substantiates the efficacy of URA-Net, demonstrating its superior performance compared to existing methods when applied to established datasets for remote sensing image defogging. On the RICE-1 dataset, URA-Net achieves a Peak Signal-to-Noise Ratio (PSNR) of 29.07 dB, surpassing the Dark Channel Prior (DCP) by 11.17 dB, the All-in-One Network for Dehazing (AOD) by 7.82 dB, the Optimal Transmission Map and Adaptive Atmospheric Light For Dehazing (OTM-AAL) by 5.37 dB, the Unsupervised Single Image Dehazing (USID) by 8.0 dB, and the Superpixel-based Remote Sensing Image Dehazing (SRD) by 8.5 dB. Particularly noteworthy, on the SateHaze1k dataset, URA-Net attains preeminence in overall performance, yielding defogged images characterized by consistent visual quality. This underscores the contribution of the research to the advancement of remote sensing technology, providing a robust and efficient solution for alleviating the adverse effects of haze on image quality.Graphical Abstract
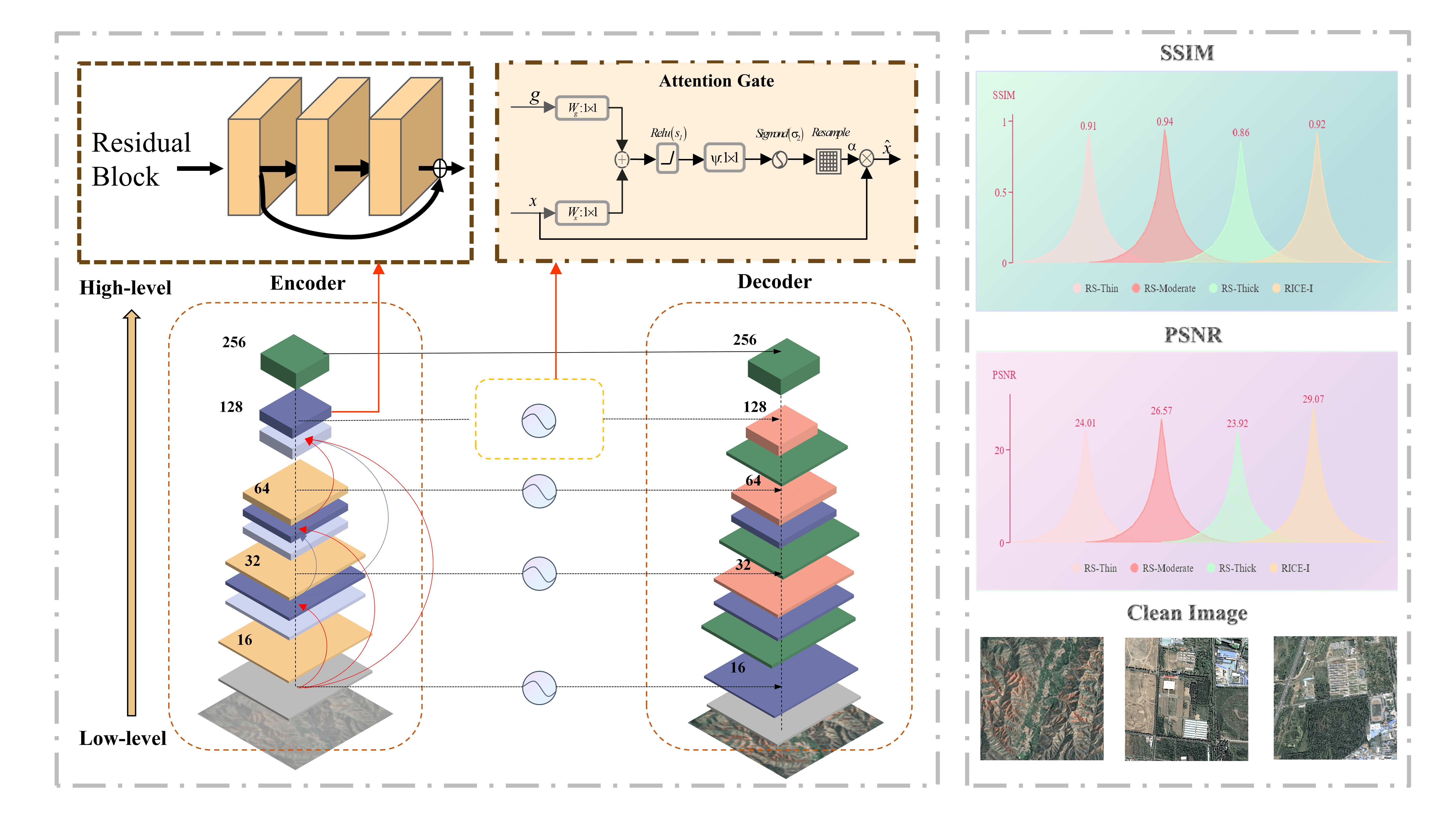
Keywords
Cite This Article
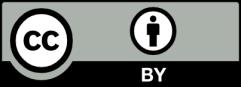