Open Access
ARTICLE
Comparing Fine-Tuning, Zero and Few-Shot Strategies with Large Language Models in Hate Speech Detection in English
Departamento de Informática y Sistemas, Universidad de Murcia, Campus de Espinardo, Murcia, 30100, Spain
* Corresponding Author: José Antonio García-Díaz. Email:
(This article belongs to the Special Issue: Emerging Artificial Intelligence Technologies and Applications)
Computer Modeling in Engineering & Sciences 2024, 140(3), 2849-2868. https://doi.org/10.32604/cmes.2024.049631
Received 12 January 2024; Accepted 02 April 2024; Issue published 08 July 2024
Abstract
Large Language Models (LLMs) are increasingly demonstrating their ability to understand natural language and solve complex tasks, especially through text generation. One of the relevant capabilities is contextual learning, which involves the ability to receive instructions in natural language or task demonstrations to generate expected outputs for test instances without the need for additional training or gradient updates. In recent years, the popularity of social networking has provided a medium through which some users can engage in offensive and harmful online behavior. In this study, we investigate the ability of different LLMs, ranging from zero-shot and few-shot learning to fine-tuning. Our experiments show that LLMs can identify sexist and hateful online texts using zero-shot and few-shot approaches through information retrieval. Furthermore, it is found that the encoder-decoder model called Zephyr achieves the best results with the fine-tuning approach, scoring 86.811% on the Explainable Detection of Online Sexism (EDOS) test-set and 57.453% on the Multilingual Detection of Hate Speech Against Immigrants and Women in Twitter (HatEval) test-set. Finally, it is confirmed that the evaluated models perform well in hate text detection, as they beat the best result in the HatEval task leaderboard. The error analysis shows that contextual learning had difficulty distinguishing between types of hate speech and figurative language. However, the fine-tuned approach tends to produce many false positives.Keywords
Cite This Article
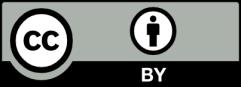