Open Access
ARTICLE
A Novel ISSA–DELM Model for Predicting Rock Mass Permeability
1 School of Engineering and Technology, China University of Geosciences (Beijing), Beijing, 100083, China
2 Beijing Engineering Corporation Limited, Beijing, 100024, China
* Corresponding Author: Leihua Yao. Email:
(This article belongs to the Special Issue: Intelligent Analysis of Imperfect Data in Complex Scenes: Modeling, Learning, and Optimization)
Computer Modeling in Engineering & Sciences 2024, 140(3), 2825-2848. https://doi.org/10.32604/cmes.2024.049330
Received 03 January 2024; Accepted 10 May 2024; Issue published 08 July 2024
Abstract
In pumped storage projects, the permeability of rock masses is a crucial parameter in engineering design and construction. The rock mass permeability coefficient (K) is influenced by various geological parameters, and previous studies aimed to establish an accurate relationship between K and geological parameters. This study uses the improved sparrow search algorithm (ISSA) to optimize the parameter settings of the deep extreme learning machine (DELM), constructing a prediction model with flexible parameter selection and high accuracy. First, the Spearman method is applied to analyze the correlation between geological parameters. A sample database is built by comprehensively selecting four geological indexes: burial depth, rock quality designation (RQD), fracture density characteristic index (FD), and rock mass integrity designation (RID). Hence, the defects of the sparrow search algorithm (SSA) are enhanced using the improved strategy, and the initial input weights of the DELM are optimized. Finally, the proposed ISSA–DELM model is employed to predict the permeability coefficient of rock mass in the entire study area. The results showed that the predictive performance of the model is superior to that of the DELM and SSA–DELM. Therefore, this model successfully provides insights into the distribution characteristics of rock mass permeability at engineering sites.Keywords
Cite This Article
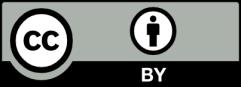
This work is licensed under a Creative Commons Attribution 4.0 International License , which permits unrestricted use, distribution, and reproduction in any medium, provided the original work is properly cited.