Open Access
ARTICLE
A Novel Graph Structure Learning Based Semi-Supervised Framework for Anomaly Identification in Fluctuating IoT Environment
1 School of Computer and Software Engineering, Xihua University, Chengdu, 610039, China
2 West China Second University Hospital, Sichuan University, Chengdu, 610065, China
3 School of Computer Science, Chongqing University, Chongqing, 400044, China
* Corresponding Authors: Peng Chen. Email: ; Yunni Xia. Email:
(This article belongs to the Special Issue: Machine Learning Empowered Distributed Computing: Advance in Architecture, Theory and Practice)
Computer Modeling in Engineering & Sciences 2024, 140(3), 3001-3016. https://doi.org/10.32604/cmes.2024.048563
Received 12 December 2023; Accepted 11 April 2024; Issue published 08 July 2024
Abstract
With the rapid development of Internet of Things (IoT) technology, IoT systems have been widely applied in healthcare, transportation, home, and other fields. However, with the continuous expansion of the scale and increasing complexity of IoT systems, the stability and security issues of IoT systems have become increasingly prominent. Thus, it is crucial to detect anomalies in the collected IoT time series from various sensors. Recently, deep learning models have been leveraged for IoT anomaly detection. However, owing to the challenges associated with data labeling, most IoT anomaly detection methods resort to unsupervised learning techniques. Nevertheless, the absence of accurate abnormal information in unsupervised learning methods limits their performance. To address these problems, we propose AS-GCN-MTM, an adaptive structural Graph Convolutional Networks (GCN)-based framework using a mean-teacher mechanism (AS-GCN-MTM) for anomaly identification. It performs better than unsupervised methods using only a small amount of labeled data. Mean Teachers is an effective semi-supervised learning method that utilizes unlabeled data for training to improve the generalization ability and performance of the model. However, the dependencies between data are often unknown in time series data. To solve this problem, we designed a graph structure adaptive learning layer based on neural networks, which can automatically learn the graph structure from time series data. It not only better captures the relationships between nodes but also enhances the model’s performance by augmenting key data. Experiments have demonstrated that our method improves the baseline model with the highest F1 value by 10.4%, 36.1%, and 5.6%, respectively, on three real datasets with a 10% data labeling rate.Keywords
Cite This Article
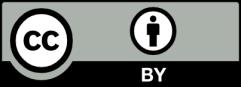
This work is licensed under a Creative Commons Attribution 4.0 International License , which permits unrestricted use, distribution, and reproduction in any medium, provided the original work is properly cited.