Open Access
ARTICLE
Efficient Penetration Testing Path Planning Based on Reinforcement Learning with Episodic Memory
1 Henan Key Laboratory of Information Security, National Engineering Technology Research Center of the Digital Switching System, Zhengzhou, 450000, China
2 School of Cryptographic Engineering, Information Engineering University, Zhengzhou, 450000, China
* Corresponding Author: Tianyang Zhou. Email:
(This article belongs to the Special Issue: Cyberspace Intelligent Mapping and Situational Awareness)
Computer Modeling in Engineering & Sciences 2024, 140(3), 2613-2634. https://doi.org/10.32604/cmes.2023.028553
Received 24 December 2022; Accepted 17 March 2023; Issue published 08 July 2024
Abstract
Intelligent penetration testing is of great significance for the improvement of the security of information systems, and the critical issue is the planning of penetration test paths. In view of the difficulty for attackers to obtain complete network information in realistic network scenarios, Reinforcement Learning (RL) is a promising solution to discover the optimal penetration path under incomplete information about the target network. Existing RL-based methods are challenged by the sizeable discrete action space, which leads to difficulties in the convergence. Moreover, most methods still rely on experts’ knowledge. To address these issues, this paper proposes a penetration path planning method based on reinforcement learning with episodic memory. First, the penetration testing problem is formally described in terms of reinforcement learning. To speed up the training process without specific prior knowledge, the proposed algorithm introduces episodic memory to store experienced advantageous strategies for the first time. Furthermore, the method offers an exploration strategy based on episodic memory to guide the agents in learning. The design makes full use of historical experience to achieve the purpose of reducing blind exploration and improving planning efficiency. Ultimately, comparison experiments are carried out with the existing RL-based methods. The results reveal that the proposed method has better convergence performance. The running time is reduced by more than 20%.Keywords
Cite This Article
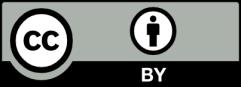
This work is licensed under a Creative Commons Attribution 4.0 International License , which permits unrestricted use, distribution, and reproduction in any medium, provided the original work is properly cited.