Open Access
REVIEW
A Review and Bibliometric Analysis of the Current Studies for the 6G Networks
1 Faculty of Computing, Universiti Malaysia Pahang Al-Sultan Abdullah (UMPSA), Pekan, Pahang, 26600, Malaysia
2 Department of Medical Equipment Technology Engineering, Al Salam University College, Baghdad, Iraq
3 School of Mathematics and Computer Science, University of Wolverhampton, Wolverhampton, 01902, UK
4 Department of Production Engineering and Metallurgy, University of Technology, Baghdad, Iraq
5 Cardiff School of Technologies, Cardiff Metropolitan University, Cardiff, CF5 2YB, UK
6 Institute for Big Data Analytics and Artificial Intelligence (IBDAAI), Kompleks Al-Khawarizmi, Universiti Teknologi MARA, Shah Alam, Selangor, 40450, Malaysia
7 Department Computer Science, Al-Salam University College, Baghdad, Iraq
* Corresponding Author: Qusay M. Salih. Email:
Computer Modeling in Engineering & Sciences 2024, 140(3), 2165-2206. https://doi.org/10.32604/cmes.2024.028132
Received 01 December 2022; Accepted 20 July 2023; Issue published 08 July 2024
Abstract
The race to develop the next generation of wireless networks, known as Sixth Generation (6G) wireless, which will be operational in 2030, has already begun. To realize its full potential over the next decade, 6G will undoubtedly necessitate additional improvements that integrate existing solutions with cutting-edge ones. However, the studies about 6G are mainly limited and scattered, whereas no bibliometric study covers the 6G field. Thus, this study aims to review, examine, and summarize existing studies and research activities in 6G. This study has examined the Scopus database through a bibliometric analysis of more than 1,000 papers published between 2017 and 2021. Then, we applied the bibliometric analysis methods by including (1) document type, (2) subject area, (3) author, and (4) country of publication. The study’s results reflect the research 6G community’s trends, highlight important research challenges, and elucidate potential directions for future research in this interesting area.Graphical Abstract
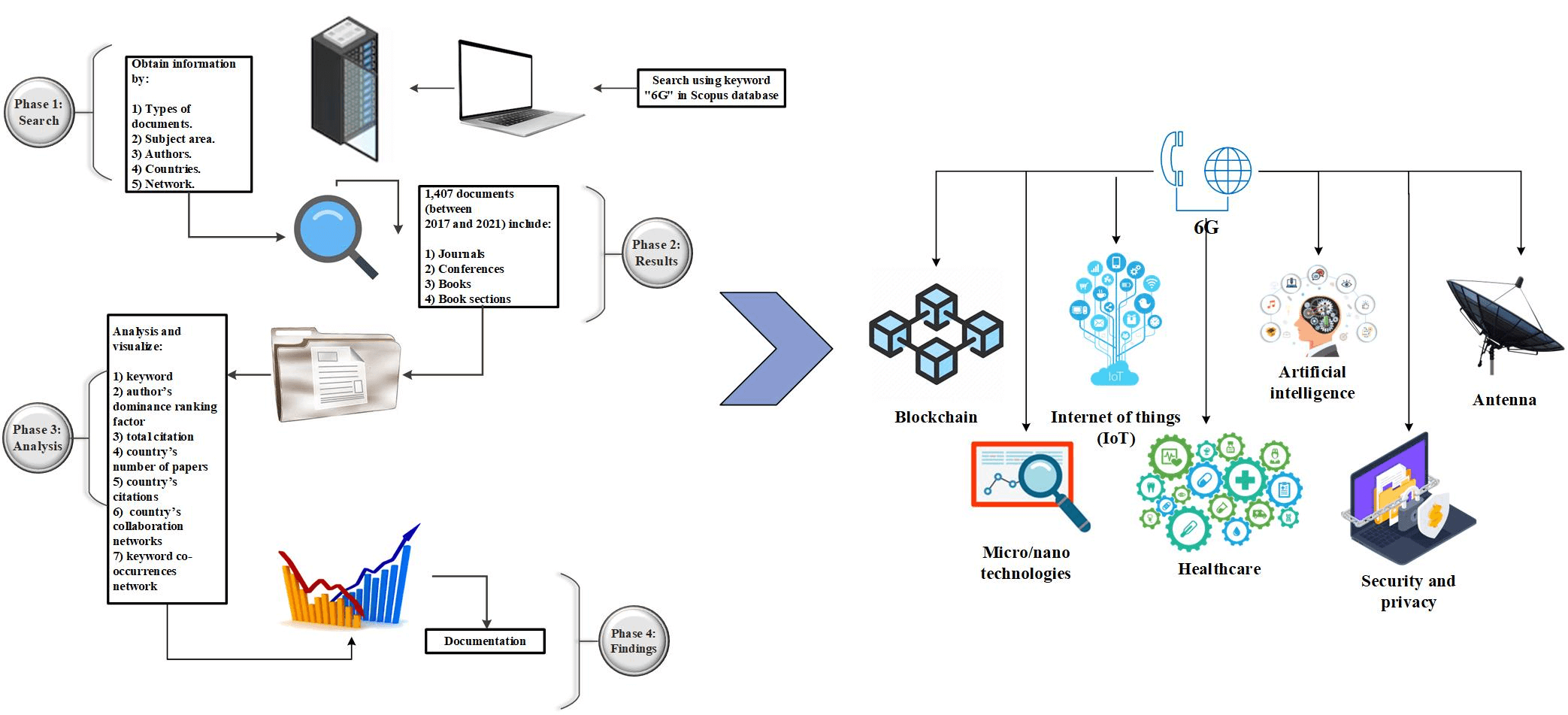
Keywords
Since the introduction of Second Generation Networks (2G), every future mobile service has been planned several years ahead of its commercial debut [1]. After almost ten years of preparation, 5G networks commenced commercial implementation in 2019. Similarly, the competition for 6G wireless networks, operational in 2030, is already underway. For 6G to reach its full potential in the next ten years, it must be built on current solutions and new technologies [1,2]. Thus, 6G technology is a future mobile communication technology critical to technological advancement [3,4].
Scholars utilize bibliometric analysis for several purposes, including identifying emerging publication and journal performance trends, cooperation patterns, research elements, and investigating the intellectual structure of a specific area in the current study. There seem to be plenty of interesting publications on the 6G topic, so it seems appropriate to conduct a Bibliometrics study based on the empirical Scopus database to determine who the leading research pioneers are interested in understanding the topic of 6G. Also, to identify gaps and redefine the boundaries of new studies. The motivation for this study is to explore and discuss topics related to 6G. Also, this study focuses on identifying the relationship between document type, subject area, author, and country.
To indicate the maturation of the 6G domain, this paper aims to instruct an examination of the 6G domain by furnishing bibliometric information on more than 1000 scientific studies dragged from the Scopus database using R software with Bibliometrics. The number of publications published, citations, research field, keywords, institutions, phrases, and authors were all examined in depth in this study. Hence, this work investigated the 6G by offering a complete examination of 6G research methodologies published on Scopus between 2017 and 2022. The method assesses 6G research, publishing trends, and research subjects.
The contributions of this study are manifold:
▪ Bibliometric study includes 10407 research retrieved from the Scopus database, which consists of all types of articles, including ISI-indexed articles.
▪ Explore the research 6G community’s trends.
▪ Investigate the 6G technologies and applications.
▪ Highlighting the challenges and potential research directions in the 6G field.
This study’s methodology is divided into four sections: (1) search, (2) result, (3) analysis, and (4) findings. The process and its phases are depicted in Fig. 1. This research begins with using as the primary keyword in the Scopus database. This process defines the research direction of 6G technologies and highlights the database they are interested in [1]. In more, in the phase of search that the Scopus database was chosen for this study since it includes both ISI and Scopus-indexed rank papers. Going to follow that, the outcome phase successfully regained 1407 papers with a limit the publication time of the paper between 2017–2021 years, and the subject areas are the communication field and computer field. The following phase of this study concentrated on revealing the information, respectively. This study focused on different domains, including scrutinizing different document types, subject areas, authors, countries, and network.
Figure 1: Methodology stages
The second phase, “result,” typically involves compiling a list of all articles that met the inclusion criteria in the search process. This list should include relevant details such as the article title, author(s), publication date, journal or conference proceedings, and other pertinent information. In the third section, “analysis”, the researcher interprets the results and draws conclusions based on the result phase. This may involve using statistical or other analytical methods to identify patterns, trends, or relationships among the data. The researcher should also consider the study’s limitations and potential avenues for future research. Finally, in the fourth phase, “findings”, the researcher presents the key results of the bibliometrics study based on the selected articles. This may include data on the number of articles published in a particular field, the most cited articles or authors, the distribution of research by geographic region, and other significant findings. By providing clear and detailed descriptions of each section of the methodology, the researcher can better understand the research process and the study results.
To create the 6G bibliometric analysis this study used R, a free source statistical tool, to make the 6G bibliometric analysis. The bibliometric package was installed and used in the R desktop application [2]. Many studies have utilized this bibliometrics instrument for their respective study fields [1]. Based on our information, this study uses bibliometric analysis in 6G for the first time. The R application can provide a variety of 6G findings and then visualizations and analysis further below.
Table 1 presents an overview of the output and a bibliometric analysis examining the 6G technology research landscape. It highlights the methodology’s essential steps and the bibliometric analysis’s corresponding outcomes.
The methodology comprises three primary stages. Firstly, the Scopus database was chosen for sourcing relevant research publications, ensuring a comprehensive and reliable search process. Secondly, the study incorporated articles, conference papers, book chapters, and books published up to 2021, enabling an extensive analysis of the existing research on 6G technology. Lastly, the analysis aimed to discover new pertinent topics, patterns, trends, and relationships within the data, providing insights for future research directions.
On the other hand, the bibliometric analysis concentrated on six major categories. The type of documents category supplies the information needed to analyze various document types, ensuring a well-rounded understanding of the research landscape. By examining subject areas, the analysis aids in better comprehending past and current research trends in 6G technology. The author category pinpoints the most active authors in 6G research based on factors such as keywords, dominance ranking, and total citations.
Additionally, the country category highlights the author’s country and offers information on the country’s total number of papers, publications, citations, and collaboration networks. The network metric is employed to analyze the 6G research landscape regarding network configuration, presenting results based on country collaboration and keyword co-occurrences network.
The bibliometric investigation’s key findings are outlined based on the chosen articles. These insights provide valuable information about the 6G research landscape and help guide further exploration and innovation in the field.
Bibliometric Analysis, usually called Bibliometrics, is a statistical technique for assessing state-of-the-art or identifying research opportunities black study [3,5]. The major search parameters utilized in the bibliometric analysis include field-specific publication years, document type, language, and area of knowledge. The bibliometric analysis of this study is broken down into five main categories, as shown in Fig. 2. On the other side, Fig. 2 also indicates that there are groups of sub-categories developed under the Authors, Country, and Network categories.
Figure 2: Bibliometric analysis categories
These sub-categories recreate an essential role in research interpretation and scientific mapping by provoking the bibliometric information used to reveal the impact of the high 6G study that contributes to creating new knowledge. These categories are considered the most commonly used in the bibliometric analysis [5–7], and they were chosen based on the research unit and the study’s objectives. We developed numerous research questions to address this study, as shown in Fig. 3.
Figure 3: Research questions
The bibliometric analysis outcomes will be discussed in the following subsections below.
Table 2 tabulates the main information about the data and document types from the Scopus database, with 1407 articles from 2017 to 2021 related to 6G. Author’s keywords are a shortlist of keywords writers in a publication have used in the complete text to characterize the subject matter of their work [1], where the keywords mentioned in the articles were three times double the articles totaling 4308. The papers had 4245 writers, with 43 single authors per article and 4202 articles having multiple authors per article. According to the findings, articles increased rapidly from 2013 to 2021 and are predicted to climb gradually by the end of 2022. Table 2 is represented visually in Fig. 4 in the form of a graph. In more detail, Fig. 4a presents the authors’ frequency distribution and the number of author appearances in a publication. There were a total of 4245 authors and 6872 author appearances, indicating a high level of participation in the publication. 43 of these author appearances were from single-authored articles, showcasing the work of individual authors. On the other hand, 4202 author appearances were from multi-authored articles, highlighting the collaborative nature of research. In summary, Fig. 4a reveals a high level of participation by authors in a publication, with a mixture of both individual and collaborative efforts being represented.
Figure 4: Overview of authors, articles, and years in the 6G field including additional information
Meanwhile, Fig. 4b shows the authors’ contributions to a publication, including the total number of author keywords and articles and the frequency of sources. There were 4308 author keywords, 1407 articles, and 319 sources, which shows that the authors wrote about many different things and used many different sources. This highlights the publication’s comprehensiveness and inclusiveness, showcasing the authors’ interdisciplinary approach to their research. The information shows that the authors covered a wide range of topics because it comes from many different fields. Fig. 4c suggests a trend of growth in the publication, with a steadily increasing number of articles being published each year. The sharp increase in 2021 shows a notable acceleration in the publication’s growth. In conclusion, the information presents a picture of growth and expansion for the publication, with a steadily increasing number of articles being published each year, particularly in 2021.
In the same fashion, the data in Fig. 4d indicate high-quality and relevant research being published, with a steady flow of citations and moderate collaboration among authors. The average number of authors per article is 3.02, and the articles per author are 0.331, showing a moderate level of productivity. The articles receive citations soon after publication.
The coupling map generated four clusters of articles identified by references based on the effect of the global citation score and labelled by authors’ keywords using 250 units, a minimal group frequency per 1,000 units of 5, three labels per cluster, and a label size of 0.3, as shown in Fig. 5. On the other hand, four cluster groups refer to the relations between the topics. This classification of clustering also explains the degree of relationship between the topics. Meanwhile, as shown in Fig. 5, it is divided into four quadrants, where every quadrant is discussed as the following:
• First quadrant (I): These are well-developed topics that play an important role in the organization of the study area. Consequently, they are characterized by high centrality as well as a high density of discussion. For example, 6G and deep learning play as an attractive topic.
• Second quadrant (II): These are highly developed and specialized subjects, yet they are minor compared to the rest of the field, namely “Niche themes”. This quadrant appears to be dominated by the concept of “knowledge translation”. Knowledge translation involves the cooperation between researchers that aimed to curtailment the gap between research and applied practice. The 6G with the Internet of Things (IoT) is the potential to be a new line of future research.
• Third quadrant (III): It comprises the emerging and sloping themes represented by low density and centrality, namely “Peripheral themes”. The 6G networks and related topics are growing up. On the other side, cybertwin and 28 GHz are slop down.
• Four quadrants (IV): It denotes a high degree of centralization and low degree of density, which is currently in its early stages of development but is crucial for the co-production domain. The most obvious finding from the analysis is that the researchers are paying more attention to the blockchain.
Figure 5: Clustering by documents coupling
The open-access type found that the gold open was more famous, referring to publishing in a fully open-access scholarly journal, as shown in Fig. 6. Conversely, IEEE Access is the first choice for 6G researchers to publish their articles, as seen in Table 3 and Fig. 7. In the same context, the IEEE Internet of Things journal also is considered an attractive journal for researchers to publish their research. On the other side, Table 3 reveals that the other journals listed a lower range in the number of published research. Meanwhile, there was a considerable increase in the number of articles published between 2018 and 2019. Then as well, the graph depicts an increased progression from 2020 to 2021. On the other hand, Fig. 7 contributes to a superior visual outcome by highlighting the period of publishing.
Figure 6: Open access types
Figure 7: Source growth
It is evident that there are many other sources with a significant number of articles published, indicating a diverse range of topics and research interests within the technology and science field. The table can be useful for researchers, students, and professionals in the field to identify the most prominent sources for their work and to stay up-to-date with the latest research trends. Overall, the table provides a valuable snapshot of the current state of scientific and technical publications and can aid in navigating the vast array of sources available in the field.
To better understand the research trend from the past to the present, this part focuses on identifying the most popular topics in the subject area of 6G based on the frequency with which keywords are employed, as illustrated in Fig. 8. The black line goes if the keyword is used with a particular frequency during the given year.
Figure 8: Trend topics
The flats’ dimensions show the year’s total usage. This figure shows the topics for the last two years to help the researcher discover the research compass direction in 6G. The 6G, blockchain, and deep learning are the most popular topic from 2020 to 2021. The significant analysis and discussion in 2021 revealed that 6G mobile communication is becoming more aware. Meanwhile, the IoT rose a hot topic combined with 6G mobile communication. Fig. 8 also explores how Artificial Intelligence (AI) and wireless communication become a starting topic, whereas AI can utilize the 6G wireless communication area. As a result, the trend topics analysis discovered many new hot topics for researchers who want to work on a new research direction in the 6G area.
The authors who were most engaged in the 6G research are listed in this section. This part used the author’s keywords, dominance ranking factor, and total citations. The authors and publications comprising the top twenty rankings are listed in Table 4. As seen in the table, with thirty-eight publications each, the WANG J has the most publications. In the same context, the authors LI J, WANG Y, and ZHANG Y also have listed twenty-nine publications. On the other hand, this section quantified each author’s contribution by examining the dominance ranking factor regarding the number of elements, as shown below.
3.3.1 Authors’ Dominance Ranking
The Dominance Factor (DF) is a ratio that calculates the proportion of publications with multiple authors in which one author is the first author [6,8,9]. Numerous bibliometric studies have utilized the DF factor in their research [4,7,10]. The DF ranking determines the supremacy of an author in article production. The DF feature is computed by dividing the number of multi-authored publications where the author has been the first author by the total number of publications (Nmf) by the total number of multi-authored publications where the author is the first author (Nmt). This is ignored in the case of a single author, as its value is always “one” for single-authored papers. The math formula for the DF factor is as described in the following:
Table 5 summarises the list of the top 20 DF authors, led by CHEN Y, who appeared as a first author in seven articles and as one of many authors in nineteen multi-authored articles. This list includes ZHANG J and LIU Y next. The result suggests that CHEN Y, ZHANG J, and LIU Y are the leaders of their research team, as they were the first author of all of their papers (seven for CHEN Y, nine for ZHANG J, and nine for LIU Y, respectively).
However, by comparing Table 5 (Dominance factor) and Table 4 (authors with a number of articles), the situation is different. Although Wang H and LI J are in the top two in the number of articles, they are ranked 12th and 16th in the Dominance factor ranking. This demonstrates that CEHN Y, ZHANG J AND LIU Y are the most dominant in 6G research.
This section explains the keywords relate to 6G technology. In their research articles, the researchers associated multiple keywords with the 6G. This is vital because it requires us to examine research trends and identify gaps in the 6G field.
Table 6 displays the total number of the author’s keywords within the top twenty positions. The top three in the ranking are 6G, 5G, and 6G mobile communication. The IoT, Blockchain, and AI can be discovered by concentrating on the following keywords. The keyword analysis identifies the study’s other major components. The IoT is a popular topic that appears through numerous studies under the IoT.
On the other side, Fig. 9 depicts the word TreeMap of terms that drew 6G researchers’ attention. Meanwhile, it demonstrates that the IoT, blockchain, AI, energy efficiency, energy harvesting, and security all contribute to 6G topics. This demonstrates that researchers can focus on combining these domains with 6G to follow new research directions.
Figure 9: Word TreeMap
The topic dendrogram depicted in Fig. 10 illustrates the hierarchical relationship and order of the keywords that hierarchical clustering generates. The cutting and vertical lines in the figure make things simpler to examine and interpret the various clusters. In addition, Fig. 10 does not seek to identify the exact relationships between sets. Instead, it estimates the approximate number of clusters to facilitate further discussion.
Figure 10: Topic dendrogram
The diagram shows that 6G areas experiment with IoT. This highlights that the researchers focus on cooperating 6G with IoT in their research field. On the other hand, 6G also combines Artificial Intelligence (AI), machine learning and deep learning. To summarize overall terms, the following picture shows a word cloud that identifies the most popular study topics worldwide.
A word cloud of terms found in 6G article research publications is shown in Fig. 11. General terms such as 6G mobile communication, wireless communication, blockchain, AI, and 5G are emphasized in the diagram. Apart from these broad concepts, the IoT, blockchain, security, deep learning, edge computing, and machine learning are some of the most appealing keywords. The 6G topic has much potential in these sectors and could lead to an increase in 6G articles in the future. In addition, the word cloud covers a variety of energy-related themes, including energy efficiency and energy harvesting. It demonstrates that energy experts believe in 6G and have implemented it in their respective research fields. According to the cloud, millimeter-wave networks are an attractive topic for 6G networks. In addition to the word cloud, the following part includes a multi-field plot between affiliations and keywords in the top 20 rankings, which can determine which nations are interested in the terms.
Figure 11: Word cloud of 6G keywords
The publications that received multiple citations from other papers and were ranked among the top 20 are listed in Table 7. This information revealed that specific articles received numerous citations in particular years, demonstrating that certain topics are only noteworthy in particular years. Several authors combined 6G technology with other fields; consequently, they only cited relevant works in their research.
Basar is the most-cited article in 2019, having received the most citations (506) of any article in any year. Saad is the second article published in 2020 and has received 450 citations. Various aspects have been cited in more articles. This shows that the papers are important since they provide information and highlight 6G and wireless applications as common study areas. When 6G results are associated with concepts such as machine learning and the IoT, the number of citations changes dramatically.
The data demonstrate that the citations come from various journals, with the IEEE ACCESS Journal being the most referenced and having the highest average TC per year (73.4444). IEEE NETWORK published an average of (52.1111) TC each year. The former journal is primarily concerned with network protocols and architectures, protocol design and validation, network control and signaling, as well as actual network implementations such as Local Area Networks (LANs), metropolitan area networks (MANs), and wide area networks (WANs). The top five IEEE sources, as shown in the table, have excellent citations totaling more than 50 citations. As seen in the table, these IEEE journals are attracting more researchers who should publicize their 6G research, with the IEEE being the most cited source. In addition to citations, the section below discusses which countries are active in 6G research.
This section discussed the countries that are interested in 6G research. It draws attention to the author’s country, mentioned in the writings. It includes the country’s total number of papers, publications, citations, and collaboration networks. The following sub-section starts with the overall number of articles by country.
3.4.1 Country Total of Articles
Fig. 12 depicts the nations conducting 6G research. China ranks first with 2,568 total articles, followed by the United States (428) and Germany (103). Notable is the fact that these three nations are located on different continents:
1. China represents the best-ranking country in Asia.
2. The United States represents North and South America.
3. Germany marks the highest publication among other countries in Europe.
Figure 12: Total of articles in the country
This information indicates that each continent has a leading nation in 6G research. The figure also shows that the red-yellow hue is more prevalent in multiple European nations. This indicates that European nations embraced 6G in their research papers more than nations from other continents. Similarly, more European nations are attempting to participate in 6G research, as numerous small red dots surround the continents. This evidence indicates that the number of 6G researchers in Europe is increasing, and we anticipate an increase in publications in the near future.
Asia, like Europe, has a strong interest in 6G research. China dominates the Asian continent, as evidenced by the actual green hues and the total number of articles. This number indicates that, compared to other countries on the continent, these nations have recognized the potential of 6G and contributed their ideas to publications.
This section analyzes the 6G articles in terms of single and multiple publications for each country. It also intends to track the networks of collaboration that emerge when 6G publications are published in different nations. Table 8 shows that some countries have published only a few shared articles, such as Japan, Iran, Iraq, Australia, France, Brazil, Pakistan, Egypt, and Spain. On the other hand, other countries prefer to publish single articles. Table 8 shows that China, India, and the USA are the top three leading countries.
Meanwhile, Fig. 13 demonstrates that China has the highest MCP and SCP, indicating that it actively publishes in both single and many publications, followed by India and the United States.
Figure 13: Country collaboration
However, the figure reveals that certain countries are less interested in publishing with others from the MCP standpoint. They were more interested in publishing in the SCP range, where the publication values were more significant than one. It is worth noting that the MCP’s highest values were lower than the SCP’s. This trend demonstrates that most countries prefer collaborating and publishing 6G research in a single country rather than across numerous countries.
3.4.3 Country Collaboration Map
Fig. 14 shows country collaboration worldwide, with black indicating the presence of cooperation in that country. Collaboration with foreign countries is more common in dark black.
Figure 14: Country collaboration map
It is worth noting that the countries with the most 6G publications also have the highest cooperation rate, implying that they have shared knowledge and aided one another in obtaining scientifically valuable findings. Because of legislation and practices, the United States, the United Kingdom, Australia, China, and a few countries in Europe had the highest networking rates among other countries. Thus, partnerships between countries will boost the number of publications.
This section lists the 6G publications that received citations from other researchers in the top 20 global rankings. Table 9 displays the total and average number of article citations. It sorts by the total number of citations, from most to least. China is ranked first, followed by the United States and India.
Table 9 shows that some countries had a low total number of citations but a high average number of article citations. Finland (70–35), Australia (115–8.85), and Italy (75–2.78) are the countries concerned. This evidence reveals that these three countries published a small number of publications, yet each piece earned a large number of citations from throughout the world. It also demonstrates that these countries wrote high-quality blockchain articles rather than a large number of them.
On the other hand, Fig. 15 resembles both the total and average number of citations. It highlights the total number of citations with a spectrum of colours (grey, yellow, and black) and the average number as a circle. The total number of citations is a measure of the frequency with which a specific article has been cited, and it reflects the significance and recognition of an article in the scientific community. The average article citations represent the average number of citations received by an article. It indicates how well an article performs relative to other articles covering the same subject. The greater the average article citations, the greater the article’s impact on the field of study. A higher total number of citations indicates that the article has a greater impact, and a higher average number of citations indicates that the article is more successful than others in the same field. Together, the two metrics help researchers evaluate the success of an article and 6G network researchers. In contrast to the preceding subsection (country publications), the United States only published 67 articles but received an exceptional 906 citations. It surpassed India, which published a greater number of publications but fewer citations than the United States. In the same context, the United States has higher average citations than China, but China published more articles overall.
Figure 15: Country with total citations on the world map
The data reveals that the United States has published in high-quality journals, attracting citations and references from other scholars. The following part presents network collaboration between nations, keywords, and affiliations in the purpose of discovering the relationship between these countries.
Scientific knowledge is a complex system, and as a result, it requires a network structure to define the relationship between its primary constituents. This section presents a network-based bibliometric analysis of the 6G. It comprises a country’s collaboration network and a network of keyword co-occurrences. This network was built so that the movement of the different nodes connected might be seen.
Scientific knowledge is a complex system. As a result, it requires a network structure to define the relationship between its primary constituents. This section presents a network-based bibliometric analysis of the 6G. It comprises a country collaboration network and a network of keyword co-occurrences. His network was developed to understand the activity of the different nodes connected.
3.5.1 Country Collaboration Network
Fig. 16 demonstrates a track of collaboration networking circles to identify actively collaborating countries. The collaboration network illustrates how the authors’ co-authorships connect them to the network. The country collaboration network is extraordinarily beneficial for fostering collaboration, sharing resources, and combining knowledge. By accessing international research, researchers can stay abreast of the most recent trends and discoveries in their field while exchanging ideas and techniques with professionals from around the globe. This helps to foster a global collaborative environment and increases research potential. The collaboration network also provides an online platform for connecting peers from various countries and regions, thereby creating invaluable information, data, and ideas resource. The network is useful for increasing awareness of various research topics, making discoveries more accessible and widespread, and fostering collaboration between international research organisations. In addition, we were able to determine which nations actively collaborate on 6G research within multiple nations and which nations prefer to conduct 6G research independently without collaboration.
Figure 16: Country collaboration layout network
The total number of articles is represented by the colored circle in each network node, as shown in the picture. According to the graph, China is the country that collaborates the most in the publication of 6G articles, followed by the United States, India, and the United Kingdom. As shown in the diagram, several lines are connected in the upper part of the collaboration network. Compared to countries in the lower portion, this indicates that the countries in the top part were actively interacting.
Italy, Denmark, Pakistan, Canada, Australia, India, Hong Kong, Japan, Nigeria, and Korea comprised the majority of the countries in the upper section. This means that Asian countries chose to work with others rather than publish in a single country regarding publishing publications in 6G research activities.
3.5.2 Keyword Co-Occurrences Network
This study also looked at the keyword co-occurrence network. The keywords co-occurrence network analysis shows the relationship between terms in the literature, revealing the field’s knowledge structure [28]. Hence, Fig. 17 shows the data needed to determine which fields were associated with 6G research, and it establishes the network’s keyword co-occurrences.
Figure 17: Keyword co-occurrences network
The authors include “6G” as a keyword rather than “6g” mobile communication, as shown in the picture. The reason for this is that 6G is a novel term that was previously excluded from the global language. As a result, there are variations in the keyword. Despite this, both phrases relate to the same word, 6G. The researchers integrate 6G with numerous keywords in the figure, showing that they combine 6G with multiple research topics. Machine learning, mmwave, IoT, energy efficiency, uncrewed aerial vehicles, and blockchain are some sectors involved in 6G research efforts.
3.5.3 Affiliation Collaboration Network
As illustrated in Fig. 18, the colored node in the network indicates the participation of universities and colleagues from various nations.
Figure 18: Affiliation collaboration network
China’s Beijing University of Posts and Telecommunications collaborates with the Chinese University of Electronic Science and Technology, Shanghai Jiao Tong University, Nanyang Technical University, and Nanjing University of Aeronautics and Astronautics. While the purple color shows that the king saud university of Saudi Arabia collaborates with fujian normal university from China. In the same context, king saud university has a partnership with the University of electronic science and technology of china. On the other side, Princeton university in the USA has a partnership with the peking university from the chain. Meanwhile, the Peking university has cooperated with Tsinghua university in china. From Bangladesh, Southeast University has collaborated with Shandong regular university and Shandong university in china. Furthermore, the xidian university and northwestern polytechnical university in China have cooperated with Tohoku university in Japan.
As a result, this figure shows that Chinese institutions prefer to collaborate with their own countries, and scientific collaboration with colleges in various countries is hesitant. As a result, more cooperation between institutions in other countries that deal with 6G technology is required.
4 Review of 6G and Recent Studies
In 6G, the 5G paradigm will be further enhanced and expanded. Moreover, the 6G system will improve user QoS (Quality of Service) and increase performance by multiples above 5G. Theoretically, the 6G network could serve as a worldwide communications hub. The per-user bit rate in 6G is expected to be around 1 Tbps (terabits per second). The simultaneous wireless connectivity provided by 6G is estimated to be 1,000 times greater than 5G. It is expected that, in addition to the KPIs of 5G communication systems, 6G will necessitate the development of new key performance indicator (KPI) drivers. Many KPIs from the 5G system will be applicable to the 6G system. However, the 5G KPIs must be revisited, and new KPIs for 6G must be considered. Several KPI classes are currently difficult to define for 6G and are expected to be finalised by future research.
Fig. 19 visualizes the comparison between 4G, 5G and 6G [29–31]. Some academic and industry researchers set the following KPIs for 6G communication: peak data rate of 1 Tbps, radio latency of 0.1 ms, battery lifetime of 20 years, device connectivity of 100/m3, traffic increase of 10,000 times, energy efficiency of ten times, maximum outage of one out of one million, and 10 cm indoor and 1 m outdoor positioning precision.
Figure 19: Comparison between 4G, 5G and 6G
The ongoing network traffic explosion and emerging applications and devices (Internet of everything or machine-to-machine (M2M) connections) are unlikely to be sustained by the current generation of wireless communication. The next generation of wireless communication systems (sixth generation (6G) networks) is being introduced to support such massive internet connections with diverse requirements regarding data rates, reliability, latency, intelligence, security, and energy efficiency. However, 6G wireless communications networks will necessitate advanced scientific research.
As depicted in Fig. 20, the 6G scientific community has been very active in elaborating visions and perspectives around enabling technologies and specifications for a few years. They have recently investigated many other critical aspects of 6G related to the blockchain, the IoT, AI, antenna, micro/nanotechnologies, healthcare, security, and privacy.
Figure 20: Recent studies in 6G
As 6G mobile networks become virtualized and cloud-based, they will serve as the foundation for the Internet of Everything. However, as communication technologies have advanced, malicious parties’ threats have become more sophisticated, making security a major concern for the future 6G era. Despite massive efforts by researchers to improve security and authentication protocols, systems continue to be vulnerable to new intrusions and attacks. As a result, blockchain technology is expected to be one of the leading supporting technologies for providing a secure and unique network to 6G-enabled devices, transactions, and applications [32–34].
The Autonomous Vehicle (AV), also known as Vehicle-to-Everything (6G-V2X) communication, is a recent research topic in the IoT with 6G [35–37]. With the ever-increasing mobile internet traffic and services, the rapid growth of Autonomous Vehicle (AV) technology and the integration of edge computing pose new challenges [38–40]. Tackling such challenges through customized edge computing services is critical research in 6G Vehicle-to-Everything (6G-V2X) communication [41–43].
On the other hand, research on 6G networks and AI has already begun in industry and academia (AI) [44,45]. AI has thus been used as a new paradigm for designing and optimising highly intelligent 6G networks. The 6G scientific community has adopted an AI-enabled intelligent architecture for 6G networks that are divided into four layers (intelligent sensing layer, data mining and analytics layer, intelligent control layer, and smart application layer) [25,46,47].
The 6G security communities also combine AI to empower health solutions for efficient, cost-effective, and technology-based strategies [48–50]. For instance, the combination of 6G and AI has the potential to manage dementia successfully (a general term for the impaired ability to remember, think, or make decisions that interfere with doing everyday activities) in patients until a cure becomes available [51–53].
Furthermore, making 6G visions a reality will necessitate using previously unseen strategies in developing Hardware-Software (HW-SW) systems, leveraging increased functional abstraction of HW, leading to its partial reconceptualization [54–57]. In the 6G scenario, microtechnologies and nanotechnologies are expected to play pivotal roles as devices, systems such as Micro/NanoElectroMechanical Systems (MEMS/NEMS), and materials, with a particularly significant estimated impact on network edge architecture [58–60].
With the deployment of more and more 5G networks, the limitations of 5G networks have been found, which undoubtedly promotes the exploratory research of 6G networks as the next-generation solutions. These investigations include the fundamental security and privacy problems associated with 6G technologies [48,58,61,62]. For instance, the SDN controllers used to manage and control UAV networks are easy targets. Furthermore, eavesdropping attacks, hijacking attacks, spoofing attacks, and DDoS attacks can also occur in many 6G applications [63,64].
Additionally, 6G researchers were also working on 6G antenna specifications (Terahertz (THz) frequency band (0.1–10 THz), that will be used in the 6G wireless communication system. It is crucial to support the user demand for higher data rates and ultra-high-speed communication for many future applications [65–67].
5 Bibliometric Discoveries in 6G Filed for Challenges and Future Research
The main goal of this bibliometric analysis is to determine how studies have evolved in terms of the type of documents, subject areas, authors, country, and network in the field of 6G. The number of articles expanded fast from 2013 to 2021, according to the type of document results. Furthermore, by the end of 2022, it is expected to raise the research number to about 6G. Blockchain, millimeter-wave, intelligent surfaces, and edge computing are predicted to have the most research impact with 6G. On the other hand, the open-access type discovered that the gold open, which refers to publishing in a completely open-access scientific publication, was more well-known. For the subject area, and according to a 2021 study, 6G mobile communication is becoming a more popular topic.
In the same vein, the IoT became a hot topic when integrated with a 6G mobile connection [67–69]. AI and wireless communication have become the newest trend topic, with artificial intelligence taking advantage of the 6g wireless communication region [70–72]. As a result of the trend topics study, researchers who want to work on a new research direction in the 6G field will find many new seductive themes. China, the USA, and Germany are the countries that have the most publications. In the same context, China, India, and the USA cooperate most with other countries and have the highest total citations in 6G publications. We could identify more particular study topics and read the most cited publications using the primary keywords, allowing us to assess the most active areas.
The scope of 6G research is intimidating to those already involved or considering becoming involved. We have already started looking into new topics and concepts to address these issues. There is an awareness that there is a limit to the number of Massive MIMO antennas that can be crammed into a small area to provide high bandwidth gain and network coverage in a compact manner. Holographic Radio Frequency (RF) systems will also be investigated in the future to boost spectrum efficiency and gain [73,74]. Cyber risks should not be overlooked regarding security, particularly in large-scale interconnected situations like the IoT, autonomous cars, etc.
Alternative approaches to increasing security without slowing down data transmission are being investigated. Advanced wireless blockchain authentication and Quantum Key Distribution will be researched to secure the end-to-end communications channel. The likelihood that 6G will benefit from OWC communications research is high. It will create a reliable system resistant to interference while providing a fast and secure wireless channel. The 6G design recognizes the risk of introducing overhead to data traffic and jeopardizing QoE and QoS [74–76]. Because of the potential added overhead, quantum computing and quantum machine learning may be developed enough to provide the proper balance of processing power [75,77,78].
In addition, researchers in the field of 6G are also attempting to improve energy efficiency and energy harvesting. As a result, designing a long-term 6G network is challenging as it needs to simultaneously offer significantly more data at higher speeds while also meeting extremely strict energy efficiency goals. This means that the amount of energy used to transmit a bit must be significantly lowered simultaneously as the transmission speed increases.
Sixth Generation (6G) wireless technology will deliver a foundation for linked devices and automation systems, from self-driving vehicles to unmanned aerial vehicles (UAVs) [39,79,80]. According to our bibliometric analysis, 6G researchers are also engaged in UAVs, also known as drones. UAVs play many important parts in our current area [79,81,82]. Examples of UAVs applications are package delivery, media production, real-time surveillance, and remote building. Hence, 6G researchers need to investigate and involve 6G with UAVs in their experiments [81,83].
Based on the tremendous bibliometric analysis, 6G is a fertile environment for many new topics for researchers wishing to begin researching 6G technology. Fig. 21 shows many new research directions, where every topic is considered ambiguous when connected with the 6G networks. Based on this figure, the researchers need extra effort to make 6G applicable to these topics and challenges as follows:
Figure 21: Research directions
5.1 Higher Data Sending Rates in Terabits/Second over Higher High-Frequency THz Bands
Terahertz is required for intercommunication between nodes and applications, which can assist in increased data rates and low-delay. This is possible with a 6G communication system [17]. However, the issue with THz frequency generation is that its value is pretty low for photonics-based devices that create optical signals and quite high for electronic units that generate microwave signals. Caused by graphene’s electrical and optical characteristics, the creation of THz frequency signals becomes feasible with the advent of new graphene-based technologies [84–86]. In the same context, the movement suffers from spreading loss due to the growth of electromagnetic waves, which is a concern. This loss increases by a factor of four based on the distance between two nodes and the operating frequency, which causes a low data rate latency [87–89].
6G networks must be more adaptable in today’s environment. One of the most important features of 6G is the capability of the network’s transceiver algorithms to be decoupled from the hardware. The software-defined method can contribute to the intelligent 6G interface [28,90,91]. Smart antennas can boost system performance by automatically modifying wave patterns based on CSI. Software Machine learning algorithms can remotely control future antennas and metamaterial [92–94]. Through intelligent operations, software-defined cognitive radios can deliver reliable wireless communications based on environmental information. Machine learning may be incorporated into cognitive radios’ spectrum detection, interference analysis, dynamic bandwidth resource management, and power control [95–97]. Machine learning approaches can optimize the end-to-end physical layer functionality and self-optimization [98–100].
The 6G network’s physical layer can provide a solid basis for high-speed data exchange. As a result, 6G will necessitate implementing effective physical layer services that can coexist with confidential and broadcast services [84,101,102]. Thus, shifting to higher radio frequencies is thus one of the challenges that must be examined. In the same context, the physical layer is also required to handle data rates in mobility scenarios that necessitate coping with far lower coherence times [103–105]. Transmission intervals will be significantly compressed due to ultra-low latency requirements. Meanwhile, the hardware architectures for the physical layer in 6G are a challenge [92,106,107].
5.4 Artificial Intelligence for 6G
According to the bibliometric data presented in Table 6, Figs. 10 and 11, a large number of 6G experimentations involve artificial intelligence, machine learning, and deep learning. This demonstrates that artificial intelligence is anticipated to power 6G networks. 6G networks offer faster, more reliable, adaptable, and more efficient connections. 6G networks will also use artificial intelligence to process high-level data faster than current 4G and 5G networks. AI-assisted networks will be able to recognize patterns that current networks cannot, enhancing the efficiency and security of data transmission [108,109]. In addition, 6G networks will use machine learning to self-heal and continuously adapt data flow management to enhance the user experience [108,110,111]. Lastly, 6G networks will continue to employ AI-assisted virtual (or augmented) realities Country collaboration network, a crucial enabler of AI in the physical world [112–114].
6G will go beyond existing mobile internet designs by supporting omnipresent AI services for devices between the network core and edge infrastructure [3,115,116]. Furthermore, AI approaches such as machine learning and deep learning will be used to build and optimize 6G infrastructures, protocols, and processes [27,24,117]. More precisely, 6G will use AI approaches to do its role while also enabling apps that run on computationally complex and data-hungry AI models via low-latency and high-bandwidth channel communication channels to specialized hardware [24,118,119].
Security has been a prominent concern to secure persons, and data from the first generation of mobile communications has been a prominent concern [25,26,120]. The most prominent security concerns during the start of cellular communications were intruders, denial of service, freeloaders, rough access points, eavesdroppers, and a few others [27,28,121,122]. However, as time passed, the security risks grew increasingly complex. A security breach can result in financial, social, and psychological damage, depending on who or what in the network gets targeted [92,123–125]. Hence, with the support of diversified system-level security against distributed denial of service, a more secure network architecture should be designed to handle the identification of billions of connected devices and 6G users [115,126,127]. A new trust model is needed to provide a confidence layer between the 6G user, 6G network, and 6G services [13,123,128].
Therefore, 6G researchers utilized blockchain. The words ”blockchain” appear in Table 6, Figs. 10 and 11 of our bibliometric analysis. This indicates that 6G researchers intend to utilize blockchain technology to enhance internet security, privacy, and trust. 6G networks can decentralize data storage and transmission via blockchain, allowing for more secure data sharing and communication without the need for centralized authorities or third parties. Distributed ledger technology (DLT) can be implemented in 6G networks to achieve end-to-end (E2E) encryption and data integrity, ensuring that only authorised parties with the proper credentials can access data. This strengthens network security against data manipulation and malicious attacks. Additionally, 6G researchers employ blockchain-based authentication, authorization, and other security protocols to ensure that only authorized entities and processes have access to data and services. This enhances the security of both network data and communications between user devices and the 6G infrastructure.
Regarding the internet of things (IoT), Table 5 lists IoT three times among the top 20 words. This demonstrates that 6G network researchers must adopt the Internet of Things (IoT) in order to realise its full potential of the IoT [81]. 6G’s ultra-high speeds and low latency will enable massive data transfers and allow IoT devices to communicate much more quickly than in the past [81]. The distributed network architecture of 6G will allow for more efficient distribution and management of system resources, making it ideal for enabling billions of IoT devices across a large geographical area [129]. By employing shorter wavelengths, 6G will enable higher data transfer rates, allowing IoT applications to process data faster than ever. In addition, 6G will improve end-to-end security and privacy, particularly when IoT devices communicate. This will ensure that only authorized parties have access to sensitive information. In addition, 6G will support a large number of simultaneous connections, which will be advantageous for the Internet of Things as the number of connected devices continues to increase [130–132].
6G will be a key enabler for future IoT networks and applications since it will offer wireless coverage and connect all applications, sensors, etc. [107,118]. Compared to the 5G mobile network, the 6G mobile network of the next generation is anticipated to provide more coverage and greater flexibility to facilitate IoT connections and service delivery [130,133,134]. However, 6G must offer real-time transmission in a dynamic environment with less latency for real-time applications [89,123,126]. Also, energy harvesting strategies that use renewable energy sources will be beneficial for developing green environmental solutions for the IoT-6G system. The integration of IoT-6G faces many challenges, which are also related to security, hardware, quality of service, and others [29,80,135]. Thus, this topic needs to get more attention and spend more effort to build a thriving environment for connecting IoT with 6G.
5.7 Connected Vehicles and Transportation
One potential research issue with using the 6G network in future transportation is ensuring secure and reliable connectivity for autonomous vehicles and other connected devices [87]. The large amounts of data transmitted by these devices will require high bandwidth and low latency to prevent accidents and ensure safe operations [126,136,137]. Additionally, the network must be able to handle interference from other devices and physical obstructions to maintain a stable connection [126]. Regarding utilization, the 6G network can be leveraged in various ways to enhance the capabilities of future transportation systems. For example, it can provide real-time traffic updates and navigation assistance to autonomous vehicles, enabling them to make smarter driving decisions [43,60,138]. The high bandwidth and low latency offered by 6G can also enable real-time communication between vehicles and other road users, helping to prevent accidents and improve overall traffic flow [129,130]. The 6G network can also provide high-definition mapping and real-time weather updates, helping autonomous vehicles navigate safely in adverse weather conditions [136].
5.8 Software-Defined Networking (SDN)
SDN is a key technology in developing 6G networks. In SDN, the network’s control plane is separated from the data plane, allowing for centralized control and management of network resources [81]. This results in more efficient use of network resources and improved network performance. One of the main benefits of SDN in 6G networks is the ability to create virtual networks within a shared physical network infrastructure through network slicing [139–141]. This allows for dedicated resources for specific use cases or applications, improving network efficiency and supporting new and innovative use cases [14,142,143]. SDN technology aims to make 6G networks more flexible, scalable, and efficient while supporting new use cases and applications [144]. By centralizing control and management of network resources, SDN can improve network performance and provide users with a more efficient and reliable network experience [145]. Moreover, SDN in 6G networks can also support the creation of new and innovative use cases and applications, such as developing smart cities, autonomous vehicles, and IoT [146]. The combination of SDN and 6G technologies is expected to bring about a new era of highly efficient, flexible, and secure communication and transportation networks.
The most pertinent limitation of this evaluation is the number and type of studies included and the identification of the source databases, even though the selected sources are dependable and representative on a broad scale. Second, a summary of research on various 6G applications does not always represent how they are used or what effects they have. In conclusion, this study looks at where 6G research is now and where it could go in the future.
The bibliometric technique used in this research was quite helpful in examining and presenting a complete picture of the 6G field. On the other side, since the data for this study is gathered from the Scopus database, there is a probability of losing out on important information. This constraint should motivate academics to seek methods to get data from numerous databases using more specific keywords to conduct a more thorough study. Meanwhile, although bibliometric methods are objective and repeatable, they need less in-depth knowledge than qualitative procedures. Citation and co-citation analytics, for instance, provide the most cited sources but not the rationale for the citation. So, the conceptual map highlights the main ideas but does not explore the paper’s content in detail. The output of this study also can aid in identifying new study fields.
The type of document data shows a rapid increase in articles from 2013 to 2021. 6G research will focus on blockchain, intelligent surfaces, edge computing, IoT, etc. The open-access type found that publishing in a fully open-access scientific journal was more popular. 2021 research shows 6G mobile communication is getting increasingly prevalent. When combined with 6G mobile, the IoT becomes a big topic. AI and wireless communication are the newest trends, with AI able to use a 6G wireless connection. The trend topics analysis will help 6G researchers uncover new attractive ideas. China, the US, and Germany are the most published countries. Also, China, India, and the USA have the most collaboration and 6G citations.
In conclusion, we identified numerous study subjects and examined the most referenced papers using primary keywords to estimate the most active areas. Thus, this study can assist researchers, especially those new to the 6G domain, in adopting interdisciplinary strategies with numerous scientific fields and technologies.
Acknowledgement: The authors are grateful to the anonymous reviewers and journal editors for their valuable feedback, which helped us improve the logic and quality of our paper.
Funding Statement: The authors received Universiti Malaysia Pahang Al-Sultan Abdullah (UMPSA) grant under Internal Research Grant with Grant Number PDU223209. Author received grant is: Ahmad Firdaus Website of the sponsor: https://www.ump.edu.my/en.
Author Contributions: Conceptualization, all authors; Methodology, Q.M.S., A.R., A.F.; Software, Q.M.S., A.H.; Validation, Q.M.S., A.F.; Formal analysis, Q.M.S.; Investigation, Q.M.S., A.R., A.F.; Resources, Q.M.S.; Data curation, Q.M.S.; Writing original draft preparation, Q.M.S., A.H.; Writing review and editing, Q.M.S., M.A.A.; Visualization, H.K., M.R.J., J.M.Z., A.H.A.; Supervision, Q.M.S.; Project administration, Q.M.S., A.R., A.F.; Funding acquisition, J.M.Z. All authors have read and agreed to the published version of the manuscript.
Availability of Data and Materials: The data that supports the findings of this study are available in the supporting information of this article.
Conflicts of Interest: The authors declare that they have no conflicts of interest to report regarding the present study.
References
1. Donthu, N., Kumar, S., Mukherjee, D., Pandey, N., Lim, W. M. (2021). How to conduct a bibliometric analysis: An overview and guidelines. Journal of Business Research, 133, 285–296. https://doi.org/10.1016/j.jbusres.2021.04.070 [Google Scholar] [CrossRef]
2. Aria, M., Cuccurullo, C. (2017). Bibliometrix: An R-tool for comprehensive science mapping analysis. Journal of Informetrics, 11(4), 959–975. https://doi.org/10.1016/j.joi.2017.08.007 [Google Scholar] [CrossRef]
3. Oliveira, O. J. D., da Silva, F. F., Juliani, F., Barbosa, L. C. F. M., Nunhes, T. V. (2019). Bibliometric method for mapping the state-of-the-art and identifying research gaps and trends in literature: An essential instrument to support the development of scientific projects. Rijeka: IntechOpen. [Google Scholar]
4. Rappaport, T. S., Xing, Y., Kanhere, O., Ju, S., Madanayake, A. et al. (2019). Wireless communications and applications above 100 GHZ: Opportunities and challenges for 6G and beyond. IEEE Access, 7, 78729–78757. https://doi.org/10.1109/Access.6287639 [Google Scholar] [CrossRef]
5. Ermel, A. P. C., Lacerda, D. P., Morandi, M. I. W. M., Gauss, L. (2021). Literature reviews: Modern methods for investigating scientific and technological knowledge. Springer Nature. [Google Scholar]
6. Kumar, S., Kumar, S. (2008). Collaboration in research productivity in oil seed research institutes of India. Proceedings of Fourth International Conference on Webometrics, Informetrics and Scientometrics, vol. 28. Humboldt-Universitat zu Berlin, Institute for Library and Information. [Google Scholar]
7. Secinaro, S., Brescia, V., Calandra, D., Biancone, P. (2020). Employing bibliometric analysis to identify suitable business models for electric cars. Journal of Cleaner Production, 264, 121503. https://doi.org/10.1016/j.jclepro.2020.121503 [Google Scholar] [CrossRef]
8. Basar, E., DiRenzo, M., DeRosny, J., Debbah, M., Alouini, M. S. et al. (2019). Wireless communications through reconfigurable intelligent surfaces. IEEE Access, 7, 116753–116773. https://doi.org/10.1109/Access.6287639 [Google Scholar] [CrossRef]
9. Saad, W., Bennis, M., Chen, M. (2020). A vision of 6G wireless systems: Applications, trends, technologies, and open research problems. IEEE Network, 34(3), 134–142. https://doi.org/10.1109/MNET.65 [Google Scholar] [CrossRef]
10. Firdaus, A., Razak, M. F. A., Feizollah, A., Hashem, I. A. T., Hazim, M. et al. (2019). The rise of blockchain: Bibliometric analysis of blockchain study. Scientometrics, 120(3), 1289–1331. https://doi.org/10.1007/s11192-019-03170-4 [Google Scholar] [CrossRef]
11. Letaief, K. B., Chen, W., Shi, Y., Zhang, J., Zhang, Y. J. A. (2019). The roadmap to 6G: AI empowered wireless networks. IEEE Communications Magazine, 57(8), 84–90. https://doi.org/10.1109/MCOM.35 [Google Scholar] [CrossRef]
12. Zhang, Z., Xiao, Y., Ma, Z., Xiao, M., Ding, Z. et al. (2019). 6G wireless networks: Vision, requirements, architecture, and key technologies. IEEE Vehicular Technology Magazine, 14(3), 28–41. https://doi.org/10.1109/MVT.10209 [Google Scholar] [CrossRef]
13. Dang, S., Amin, O., Shihada, B., Alouini, M. S. (2020). What should 6G be? Nature Electronics, 3(1), 20–29. https://doi.org/10.1038/s41928-019-0355-6 [Google Scholar] [CrossRef]
14. Yang, P., Xiao, Y., Xiao, M., Li, S. (2019). 6G wireless communications: Vision and potential techniques. IEEE Network, 33(4), 70–75. https://doi.org/10.1109/MNET.65 [Google Scholar] [CrossRef]
15. Soundiraraju, B., George, B. K. (2017). Two-dimensional titanium nitride (Ti2N) MXene: Synthesis, characterization, and potential application as surface-enhanced Raman scattering substrate. ACS Nano, 11(9), 8892–8900. https://doi.org/10.1021/acsnano.7b03129. [Google Scholar] [PubMed] [CrossRef]
16. David, K., Berndt, H. (2018). 6G vision and requirements: Is there any need for beyond 5G? IEEE Vehicular Technology Magazine, 13(3), 72–80. https://doi.org/10.1109/MVT.10209 [Google Scholar] [CrossRef]
17. Giordani, M., Polese, M., Mezzavilla, M., Rangan, S., Zorzi, M. (2020). Toward 6G networks: Use cases and technologies. IEEE Communications Magazine, 58(3), 55–61. https://doi.org/10.1109/MCOM.35 [Google Scholar] [CrossRef]
18. DiRenzo, M., Zappone, A., Debbah, M., Alouini, M. S., Yuen, C. et al. (2020). Smart radio environments empowered by reconfigurable intelligent surfaces: How it works, state of research, and the road ahead. IEEE Journal on Selected Areas in Communications, 38(11), 2450–2525. https://doi.org/10.1109/JSAC.49 [Google Scholar] [CrossRef]
19. Huang, C., Hu, S., Alexandropoulos, G. C., Zappone, A., Yuen, C. et al. (2020). Holographic MIMO surfaces for 6G wireless networks: Opportunities, challenges, and trends. IEEE Wireless Communications, 27(5), 118–125. https://doi.org/10.1109/MWC.7742 [Google Scholar] [CrossRef]
20. Calvanese Strinati, E., Barbarossa, S., Gonzalez-Jimenez, J. L., Ktenas, D., Cassiau, N. et al. (2019). 6G: The next frontier: From holographic messaging to artificial intelligence using sub tera hertz and visible light communication. IEEE Vehicular Technology Magazine, 14(3), 42–50. https://doi.org/10.1109/MVT.10209 [Google Scholar] [CrossRef]
21. Tang, F., Kawamoto, Y., Kato, N., Liu, J. (2020). Future intelligent and secure vehicular network toward 6G: Machine-learning approaches. Proceedings of the IEEE, 108(2), 292–307. https://doi.org/10.1109/PROC.5 [Google Scholar] [CrossRef]
22. Zhang, C., Li, C., Yu, J., Jiang, S., Xu, S. et al. (2018). Sers activated platform with three-dimensional hot spots and tunable nanometer gap. Sensors and Actuators B: Chemical, 258, 163–171. https://doi.org/10.1016/j.snb.2017.11.080 [Google Scholar] [CrossRef]
23. Park, J., Samarakoon, S., Bennis, M., Debbah, M. (2019). Wireless network intelligence at the edge. Proceedings of the IEEE, 107(11), 2204–2239. https://doi.org/10.1109/PROC.5 [Google Scholar] [CrossRef]
24. Nawaz, S. J., Sharma, S. K., Wyne, S., Patwary, M. N., Asaduzzaman, M. (2019). Quantum machine learning for 6G communication networks: State-of-the-art and vision for the future. IEEE Access, 7, 46317–46350. https://doi.org/10.1109/Access.6287639 [Google Scholar] [CrossRef]
25. Gui, G., Liu, M., Tang, F., Kato, N., Adachi, F. (2020). 6G: Opening new horizons for integration of comfort, security, and intelligence. IEEE Wireless Communications, 27(5), 126–132. https://doi.org/10.1109/MWC.7742 [Google Scholar] [CrossRef]
26. Basar, E. (2020). Reconfigurable intelligent surface-based index modulation: A new beyond MIMO paradigm for 6G. IEEE Transactions on Communications, 68(5), 3187–3196. https://doi.org/10.1109/TCOMM.26 [Google Scholar] [CrossRef]
27. Huang, C., Mo, R., Yuen, C. (2020). Reconfigurable intelligent surface assisted multiuser MISO systems exploiting deep reinforcement learning. IEEE Journal on Selected Areas in Communications, 38(8), 1839–1850. https://doi.org/10.1109/JSAC.49 [Google Scholar] [CrossRef]
28. Zhang, S., Zhu, D. (2020). Towards artificial intelligence enabled 6G: State of the art, challenges, and opportunities. Computer Networks, 183, 107556. https://doi.org/10.1016/j.comnet.2020.107556 [Google Scholar] [CrossRef]
29. Chowdhury, M. Z., Shahjalal, M., Ahmed, S., Jang, Y. M. (2020). 6G wireless communication systems: Applications, requirements, technologies, challenges, and research directions. IEEE Open Journal of the Communications Society, 1, 957–975. https://doi.org/10.1109/OJCOMS [Google Scholar] [CrossRef]
30. Abbas, G., Abbas, Z. H., Bilal, M., Shah, S. C., Song, H. et al. (2022). Reliability analysis of cognitive radio networks with reserved spectrum for 6G-IoT. IEEE Transactions on Network and Service Management, 19(3), 2726–2737. https://doi.org/10.1109/TNSM.2022.3168669 [Google Scholar] [CrossRef]
31. Kamruzzaman, M., Hossin, M. A., Alrashdi, I., Alanazi, S., Alruwaili, M. et al. (2022). Wireless communication channel modeling using machine learning. Transactions on Emerging Telecommunications Technologies, e4661. https://doi.org/10.1002/ett.4661 [Google Scholar] [CrossRef]
32. Asim, J., Khan, A. S., Saqib, R. M., Abdullah, J., Ahmad, Z. et al. (2022). Blockchain-based multifactor authentication for future 6G cellular networks: A systematic review. Applied Sciences, 12(7), 3551. https://doi.org/10.3390/app12073551 [Google Scholar] [CrossRef]
33. Kouhalvandi, L., Shayea, I., Ozoguz, S., Mohamad, H. (2022). Overview of evolutionary algorithms and neural networks for modern mobile communication. Transactions on Emerging Telecommunications Technologies, 33(9), e4579. https://doi.org/10.1002/ett.v33.9 [Google Scholar] [CrossRef]
34. He, Y., Zhang, L., Liu, S., Zhang, H., Yu, X. (2022). Secure transmission of terahertz signals with multiple eavesdroppers. Micromachines, 13(8), 1300. https://doi.org/10.3390/mi13081300 [Google Scholar] [PubMed] [CrossRef]
35. Nguyen, D. C., Ding, M., Pathirana, P. N., Seneviratne, A., Li, J. et al. (2021). 6G internet of things: A comprehensive survey. IEEE Internet of Things Journal, 9(1), 359–383. [Google Scholar]
36. Wei, Z., Liu, F., Masouros, C., Su, N., Petropulu, A. P. (2022). Toward multi-functional 6G wireless networks: Integrating sensing, communication, and security. IEEE Communications Magazine, 60(4), 65–71. https://doi.org/10.1109/MCOM.002.2100972 [Google Scholar] [CrossRef]
37. Deebak, B. D., Al-Turjman, F., Alazab, M. (2021). Dynamic-driven congestion control and segment rerouting in the 6G-enabled data networks. IEEE Transactions on Industrial Informatics, 17(10), 7165–7173. https://doi.org/10.1109/TII.2020.3023944 [Google Scholar] [CrossRef]
38. Nomikos, N., Zoupanos, S., Charalambous, T., Krikidis, I. (2022). A survey on reinforcement learning-aided caching in heterogeneous mobile edge networks. IEEE Access, 10, 4380–4413. https://doi.org/10.1109/ACCESS.2022.3140719 [Google Scholar] [CrossRef]
39. Li, M., Yu, F. R., Si, P., Zhang, Y., Qian, Y. (2022). Intelligent resource optimization for blockchain-enabled IoT in 6G via collective reinforcement learning. IEEE Network, 36(6), 175–182. https://doi.org/10.1109/MNET.105.2100516 [Google Scholar] [CrossRef]
40. Shafin, R., Liu, L., Chandrasekhar, V., Chen, H., Reed, J. et al. (2020). Artificial intelligence-enabled cellular networks: A critical path to beyond-5G and 6G. IEEE Wireless Communications, 27(2), 212–217. https://doi.org/10.1109/MWC.7742 [Google Scholar] [CrossRef]
41. Prathiba, S. B., Raja, G., Anbalagan, S., Gurumoorthy, S., Kumar, N. et al. (2021). CyberTwin-driven federated learning based personalized service provision for 6G-V2X. IEEE Transactions on Vehicular Technology, 71(5), 4632–4641. [Google Scholar]
42. Rana, A., Taneja, A., Saluja, N., Rani, S., Singh, A. et al. (2022). Intelligent network solution for improved efficiency in 6G-enabled expanded IoT network. Electronics, 11(16), 2569. https://doi.org/10.3390/electronics11162569 [Google Scholar] [CrossRef]
43. Ahmad, I., Rodriguez, F., Huusko, J., Seppänen, K. (2023). On the dependability of 6G networks. Electronics, 12(6), 1472. https://doi.org/10.3390/electronics12061472 [Google Scholar] [CrossRef]
44. Shahzad, K., Aseeri, A. O., Shah, M. A. (2022). A blockchain-based authentication solution for 6G communication security in tactile networks. Electronics, 11(9), 1374. https://doi.org/10.3390/electronics11091374 [Google Scholar] [CrossRef]
45. Lin, J., Long, W., Zhang, A., Chai, Y. (2020). Blockchain and IoT-based architecture design for intellectual property protection. International Journal of Crowd Science, 4(3), 283–293. https://doi.org/10.1108/IJCS-03-2020-0007 [Google Scholar] [CrossRef]
46. Yang, H., Alphones, A., Xiong, Z., Niyato, D., Zhao, J. et al. (2020). Artificial-intelligence-enabled intelligent 6G networks. IEEE Network, 34(6), 272–280. https://doi.org/10.1109/MNET.65 [Google Scholar] [CrossRef]
47. Wang, M., Zhu, T., Zhang, T., Zhang, J., Yu, S. et al. (2020). Security and privacy in 6G networks: New areas and new challenges. Digital Communications and Networks, 6(3), 281–291. https://doi.org/10.1016/j.dcan.2020.07.003 [Google Scholar] [CrossRef]
48. Li, J., Shi, W., Ye, Q., Zhang, S., Zhuang, W. et al. (2021). Joint virtual network topology design and embedding for CyberTwin-enabled 6G core networks. IEEE Internet of Things Journal, 8(22), 16313–16325. https://doi.org/10.1109/JIOT.2021.3097053 [Google Scholar] [CrossRef]
49. Saad, A., Al-Ma’aitah, M., Alwadain, A. (2020). 6G technology based advanced virtual multi-purpose embedding algorithm to solve far-reaching network effects. Computer Communications, 160, 749–758. https://doi.org/10.1016/j.comcom.2020.07.025 [Google Scholar] [CrossRef]
50. Marinho, R., Vieira, L., Vieira, M., Loureiro, A. (2023). CAIN: An energy-aware and intelligent increasing coverage area routing protocol for future 6G networks. Computer Networks, 228, 109733. https://doi.org/10.1016/j.comnet.2023.109733 [Google Scholar] [CrossRef]
51. Su, Z., Bentley, B. L., McDonnell, D., Ahmad, J., He, J. et al. (2022). 6G and artificial intelligence technologies for dementia care: Literature review and practical analysis. Journal of Medical Internet Research, 24(4), e30503. https://doi.org/10.2196/30503 [Google Scholar] [PubMed] [CrossRef]
52. Sajjad, M., Pasha, M., Pasha, U. (2022). Parametric evaluation of e-health systems. International Journal of Information Systems and Computer Technologies, 1(1). [Google Scholar]
53. Barakabitze, A. A., Walshe, R. (2022). SDN and NFV for QOE-driven multimedia services delivery: The road towards 6G and beyond networks. Computer Networks, 214. [Google Scholar]
54. Meena, P., Pal, M. B., Jain, P. K., Pamula, R. (2022). 6G communication networks: Introduction, vision, challenges, and future directions. Wireless Personal Communications, 125(2), 1097–1123. https://doi.org/10.1007/s11277-022-09590-5 [Google Scholar] [CrossRef]
55. Kamruzzaman, M. M. (2022). Key technologies, applications and trends of internet of things for energy-efficient 6G wireless communication in smart cities. Energies, 15(15), 5608. https://doi.org/10.3390/en15155608 [Google Scholar] [CrossRef]
56. Renda, A., Ducange, P., Marcelloni, F., Sabella, D., Filippou, M. et al. (2022). Federated learning of explainable AI models in 6G systems: Towards secure and automated vehicle networking. Information, 13(8), 395. https://doi.org/10.3390/info13080395 [Google Scholar] [CrossRef]
57. JaiVinita, L., Vetriselvi, V. (2023). Federated learning-based misbehaviour detection on an emergency message dissemination scenario for the 6G-enabled internet of vehicles. Ad Hoc Networks, 144, 103153. https://doi.org/10.1016/j.adhoc.2023.103153 [Google Scholar] [CrossRef]
58. Iannacci, J., Poor, H. V. (2022). Review and perspectives of micro/nano technologies as key-enablers of 6G. IEEE Access, 10, 55428–55458. https://doi.org/10.1109/ACCESS.2022.3176348 [Google Scholar] [CrossRef]
59. Jain, D. K., Tyagi, S. K. S., Neelakandan, S., Prakash, M., Natrayan, L. (2022). Metaheuristic optimization-based resource allocation technique for CyberTwin-driven 6G on IoE environment. IEEE Transactions on Industrial Informatics, 18(7), 4884–4892. https://doi.org/10.1109/TII.2021.3138915 [Google Scholar] [CrossRef]
60. Huo, L., Jiang, D., Lv, Z. (2022). A software-defined networks-based measurement method of network traffic for 6G technologies. Transactions on Emerging Telecommunications Technologies, 33(4), e4172. https://doi.org/10.1002/ett.v33.4 [Google Scholar] [CrossRef]
61. Hassan, N. H., Fakharudin, A. S. (2021). Model for phishing websites classification using artificial neural network. International Journal of Software Engineering and Computer Systems, 7(2), 1–8. https://doi.org/10.15282/ijsecs [Google Scholar] [CrossRef]
62. Stergiou, C., Psannis, K. E., Gupta, B. B., Ishibashi, Y. (2018). Security, privacy & efficiency of sustainable cloud computing for big data & IoT. Sustainable Computing: Informatics and Systems, 19, 174–184. [Google Scholar]
63. Wang, C., Li, B., Li, K., Chen, J. (2023). A UAV migration-based decision-making scheme for on-demand service in 6G network. Alexandria Engineering Journal, 69, 25–33. https://doi.org/10.1016/j.aej.2023.01.034 [Google Scholar] [CrossRef]
64. Singh, H., Kumar, V., Saxena, K., Kapse, V., Bonev, B. et al. (2023). Smart channel modelling for cloud and fog attenuation using ML for designing of 6G networks at D and G bands. Wireless Personal Communications, 129(3), 1669–1692. https://doi.org/10.1007/s11277-023-10201-0 [Google Scholar] [CrossRef]
65. Hajiyat, Z. R., Ismail, A., Sali, A., Hamidon, M. N. (2021). Antenna in 6G wireless communication system: Specifications, challenges, and research directions. Optik, 231, 166415. https://doi.org/10.1016/j.ijleo.2021.166415 [Google Scholar] [CrossRef]
66. Manogaran, G., Baabdullah, T., Rawat, D. B., Shakeel, P. M. (2022). AI-assisted service virtualization and flow management framework for 6G-enabled cloud-software-defined network-based IoT. IEEE Internet of Things Journal, 9(16), 14644–14654. https://doi.org/10.1109/JIOT.2021.3077895 [Google Scholar] [CrossRef]
67. Soni, M., Singh, D. (2023). Blockchain-based group authentication scheme for 6G communication network. Physical Communication, 57, 102005. https://doi.org/10.1016/j.phycom.2023.102005 [Google Scholar] [CrossRef]
68. Aljubayrin, S. (2023). Computational energy efficient trajectory planning for UAV-enabled 6G MEC communication network. Physical Communication, 57, 102000. https://doi.org/10.1016/j.phycom.2023.102000 [Google Scholar] [CrossRef]
69. Kavaiya, S., Patel, D. (2023). Restricting passive attacks in 6G vehicular networks: A physical layer security perspective. Wireless Networks, 29(3), 1355–1365. https://doi.org/10.1007/s11276-022-03189-1 [Google Scholar] [CrossRef]
70. Yang, X., Xing, H., Su, X. (2023). AI-based sound source localization system with higher accuracy. Future Generation Computer Systems, 141, 1–15. https://doi.org/10.1016/j.future.2022.10.023 [Google Scholar] [CrossRef]
71. Pawase, C., Chang, K. (2023). 5G-NR physical layer-based solutions to support high mobility in 6G non-terrestrial networks. Drones, 7(3). [Google Scholar]
72. Chaoub, A., Mammela, A., Martinez-Julia, P., Chaparadza, R., Elkotob, M. et al. (2023). Hybrid self-organizing networks: Evolution, standardization trends, and a 6G architecture vision. IEEE Communications Standards Magazine, 7(1), 14–22. https://doi.org/10.1109/MCOMSTD.0002.2200049 [Google Scholar] [CrossRef]
73. Pei, J., Li, S., Yu, Z., Ho, L., Liu, W. et al. (2023). Federated learning encounters 6G wireless communication in the scenario of internet of things. IEEE Communications Standards Magazine, 7(1), 94–100. https://doi.org/10.1109/MCOMSTD.0005.2200044 [Google Scholar] [CrossRef]
74. Faisal, T., Lucena, J., Lopez, D., Wang, C., Dohler, M. (2023). How to design autonomous service level agreements for 6G. IEEE Communications Magazine, 61(3), 80–85. https://doi.org/10.1109/MCOM.001.2200131 [Google Scholar] [CrossRef]
75. Jain, P., Gupta, A., Kumar, N., Guizani, M. (2023). Dynamic and efficient spectrum utilization for 6G with THz, mmWave, and RF band. IEEE Transactions on Vehicular Technology, 72(3), 3264–3273. https://doi.org/10.1109/TVT.2022.3215487 [Google Scholar] [CrossRef]
76. Louvros, S., Paraskevas, M., Chrysikos, T. (2023). QoS-aware resource management in 5G and 6G cloud-based architectures with priorities. Information, 14(3), 175. https://doi.org/10.3390/info14030175 [Google Scholar] [CrossRef]
77. Jiang, W., Han, B., Habibi, M. A., Schotten, H. D. (2021). The road towards 6G: A comprehensive survey. IEEE Open Journal of the Communications Society, 2, 334–366. https://doi.org/10.1109/OJCOMS [Google Scholar] [CrossRef]
78. Liu, F., Cui, Y., Masouros, C., Xu, J., Han, T. X. et al. (2022). Integrated sensing and communications: Toward dual-functional wireless networks for 6G and beyond. IEEE Journal on Selected Areas in Communications, 40(6), 1728–1767. https://doi.org/10.1109/JSAC.2022.3156632 [Google Scholar] [CrossRef]
79. Hu, J., Chen, C., Cai, L., Khosravi, M. R., Pei, Q. et al. (2021). UAV-assisted vehicular edge computing for the 6G internet of vehicles: Architecture, intelligence, and challenges. IEEE Communications Standards Magazine, 5(2), 12–18. https://doi.org/10.1109/MCOMSTD.001.2000017 [Google Scholar] [CrossRef]
80. Gismalla, M. S. M., Azmi, A. I., BinSalim, M. R., Abdullah, M. F. L., Iqbal, F. et al. (2022). Survey on device to device (D2D) communication for 5GB/6G networks: Concept, applications, challenges, and future directions. IEEE Access, 10, 30792–30821. https://doi.org/10.1109/ACCESS.2022.3160215 [Google Scholar] [CrossRef]
81. Mozaffari, M., Lin, X., Hayes, S. (2021). Toward 6G with connected sky: UAVs and beyond. IEEE Communications Magazine, 59(12), 74–80. https://doi.org/10.1109/MCOM.005.2100142 [Google Scholar] [CrossRef]
82. Porambage, P., Gür, G., Osorio, D. P. M., Liyanage, M., Gurtov, A. et al. (2021). The roadmap to 6G security and privacy. IEEE Open Journal of the Communications Society, 2, 1094–1122. https://doi.org/10.1109/OJCOMS.2021.3078081 [Google Scholar] [CrossRef]
83. Liu, R., Liu, A., Qu, Z., Xiong, N. N. (2021). An UAV-enabled intelligent connected transportation system with 6G communications for internet of vehicles. IEEE Transactions on Intelligent Transportation Systems. [Google Scholar]
84. Imoize, A. L., Adedeji, O., Tandiya, N., Shetty, S. (2021). 6G enabled smart infrastructure for sustainable society: Opportunities, challenges, and research roadmap. Sensors, 21(5), 1709. https://doi.org/10.3390/s21051709 [Google Scholar] [PubMed] [CrossRef]
85. Slalmi, A., Chaibi, H., Chehri, A., Saadane, R., Jeon, G. (2021). Toward 6G: Understanding network requirements and key performance indicators. Transactions on Emerging Telecommunications Technologies, 32(3), e4201. https://doi.org/10.1002/ett.v32.3 [Google Scholar] [CrossRef]
86. Catak, F. O., Kuzlu, M., Catak, E., Cali, U., Unal, D. (2022). Security concerns on machine learning solutions for 6G networks in mmWave beam prediction. Physical Communication, 52, 101626. https://doi.org/10.1016/j.phycom.2022.101626 [Google Scholar] [CrossRef]
87. Guan, K., Yi, H., He, D., Ai, B., Zhong, Z. (2021). Towards 6G: Paradigm of realistic terahertz channel modeling. China Communications, 18(5), 1–18. https://doi.org/10.23919/JCC.2021.05.001 [Google Scholar] [CrossRef]
88. Yi, H. (2022). A secure blockchain system for internet of vehicles based on 6G-enabled network in box. Computer Communications, 186, 45–50. https://doi.org/10.1016/j.comcom.2022.01.007 [Google Scholar] [CrossRef]
89. Zhang, Z., Cao, Y., Cui, Z., Zhang, W., Chen, J. (2021). A many-objective optimization based intelligent intrusion detection algorithm for enhancing security of vehicular networks in 6G. IEEE Transactions on Vehicular Technology, 70(6), 5234–5243. https://doi.org/10.1109/TVT.2021.3057074 [Google Scholar] [CrossRef]
90. Qi, Q., Chen, X., Khalili, A., Zhong, C., Zhang, Z. et al. (2022). Integrating sensing, computing, and communication in 6G wireless networks: Design and optimization. IEEE Transactions on Communications, 70(9), 6212–6227. https://doi.org/10.1109/TCOMM.2022.3190363 [Google Scholar] [CrossRef]
91. Duong, T. Q., Kim, K. J., Kaleem, Z., Bui, M. P., Vo, N. S. (2022). UAV caching in 6G networks: A survey on models, techniques, and applications. Physical Communication, 51, 101532. https://doi.org/10.1016/j.phycom.2021.101532 [Google Scholar] [CrossRef]
92. Ray, P. P. (2021). A perspective on 6G: Requirement, technology, enablers, challenges and future road map. Journal of Systems Architecture, 118, 102180. https://doi.org/10.1016/j.sysarc.2021.102180 [Google Scholar] [CrossRef]
93. Asghar, M. Z., Memon, S. A., Hamalainen, J. (2022). Evolution of wireless communication to 6G: Potential applications and research directions. Sustainability, 14(10), 6356. https://doi.org/10.3390/su14106356 [Google Scholar] [CrossRef]
94. Li, T., Liu, W., Zeng, Z., Xiong, N. N. (2022). DRLR: A deep-reinforcement-learning-based recruitment scheme for massive data collections in 6G-based IoT networks. IEEE Internet of Things Journal, 9(16), 14595–14609. https://doi.org/10.1109/JIOT.2021.3067904 [Google Scholar] [CrossRef]
95. Kamruzzaman, M. M. (2023). 6G wireless communication assisted security management using cloud edge computing. Expert Systems, 40(4), e13061. https://doi.org/10.1111/exsy.v40.4 [Google Scholar] [CrossRef]
96. Hui, Y., Cheng, N., Su, Z., Huang, Y., Zhao, P. et al. (2022). Secure and personalized edge computing services in 6G heterogeneous vehicular networks. IEEE Internet of Things Journal, 9(8), 5920–5931. https://doi.org/10.1109/JIOT.2021.3065970 [Google Scholar] [CrossRef]
97. Sun, B., Geng, R., Zhang, L., Li, S., Shen, T. et al. (2022). Securing 6G-enabled IoT/IoV networks by machine learning and data fusion. EURASIP Journal on Wireless Communications and Networking, 2022(1), 1–17. [Google Scholar]
98. Huang, T., Yang, W., Wu, J., Ma, J., Zhang, X. et al. (2019). A survey on green 6G network: Architecture and technologies. IEEE Access, 7, 175758–175768. https://doi.org/10.1109/Access.6287639 [Google Scholar] [CrossRef]
99. Mao, B., Tang, F., Kawamoto, Y., Kato, N. (2022). Ai models for green communications towards 6G. IEEE Communications Surveys and Tutorials, 24(1), 210–247. https://doi.org/10.1109/COMST.2021.3130901 [Google Scholar] [CrossRef]
100. Liang, J., Li, L., Zhao, C. (2021). A transfer learning approach for compressed sensing in 6G-IoT. IEEE Internet of Things Journal, 8(20), 15276–15283. https://doi.org/10.1109/JIOT.2021.3053088 [Google Scholar] [CrossRef]
101. Akyildiz, I. F., Kak, A., Nie, S. (2020). 6G and beyond: The future of wireless communications systems. IEEE Access, 8, 133995–134030. https://doi.org/10.1109/Access.6287639 [Google Scholar] [CrossRef]
102. Sizer, T., Samardzija, D., Viswanathan, H., Le, S., Bidkar, S. et al. (2022). Integrated solutions for deployment of 6G mobile networks. Journal of Lightwave Technology, 40(2), 346–357. https://doi.org/10.1109/JLT.2021.3110436 [Google Scholar] [CrossRef]
103. Aslam, M. M., Du, L., Zhang, X., Chen, Y., Ahmed, Z. et al. (2021). Sixth generation (6G) cognitive radio network (CRN) application, requirements, security issues, and key challenges. Wireless Communications & Mobile Computing, 2021, 1331428. [Google Scholar]
104. Zhang, X., Wang, J., Poor, H. V. (2020). Statistical delay and error-rate bounded QOS provisioning over mmWave cell-free M-MIMO and FBC-HARQ-IR based 6G wireless networks. IEEE Journal on Selected Areas in Communications, 38(8), 1661–1677. https://doi.org/10.1109/JSAC.49 [Google Scholar] [CrossRef]
105. Viswanathan, H., Mogensen, P. E. (2020). Communications in the 6G Era. IEEE Access, 8, 57063–57074. https://doi.org/10.1109/Access.6287639 [Google Scholar] [CrossRef]
106. Qi, W., Li, Q., Song, Q., Guo, L., Jamalipour, A. (2021). Extensive edge intelligence for future vehicular networks in 6G. IEEE Wireless Communications, 28(4), 128–135. https://doi.org/10.1109/MWC.001.2000393 [Google Scholar] [CrossRef]
107. Kouroshnezhad, S., Peiravi, A., Haghighi, M. S., Jolfaei, A. (2021). Energy-efficient drone trajectory planning for the localization of 6G-enabled IoT devices. IEEE Internet of Things Journal, 8(7), 5202–5210. https://doi.org/10.1109/JIOT.2020.3032347 [Google Scholar] [CrossRef]
108. Feng, Z., Wei, Z., Chen, X., Yang, H., Zhang, Q. et al. (2021). Joint communication, sensing, and computation enabled 6G intelligent machine system. IEEE Network, 35(6), 34–42. https://doi.org/10.1109/MNET.121.2100320 [Google Scholar] [CrossRef]
109. Zhang, S., Liu, J., Guo, H., Qi, M., Kato, N. (2020). Envisioning device-to-device communications in 6G. IEEE Network, 34(3), 86–91. https://doi.org/10.1109/MNET.65 [Google Scholar] [CrossRef]
110. Shen, X., Liao, W., Yin, Q. (2022). A novel wireless resource management for the 6G-enabled high-density internet of things. IEEE Wireless Communications, 29(1), 32–39. https://doi.org/10.1109/MWC.003.00311 [Google Scholar] [CrossRef]
111. Leyva, L., Castanheira, D., Silva, A., Gameiro, A., Hanzo, L. (2021). Cooperative multiterminal radar and communication: A new paradigm for 6G mobile networks. IEEE Vehicular Technology Magazine, 16(4), 38–47. https://doi.org/10.1109/MVT.2021.3114138 [Google Scholar] [CrossRef]
112. Wang, C. X., Huang, J., Wang, H., Gao, X., You, X. et al. (2020). 6G wireless channel measurements and models: Trends and challenges. IEEE Vehicular Technology Magazine, 15(4), 22–32. https://doi.org/10.1109/MVT.10209 [Google Scholar] [CrossRef]
113. Li, Y., Huang, J., Sun, Q., Sun, T., Wang, S. (2021). Cognitive service architecture for 6G core network. IEEE Transactions on Industrial Informatics, 17(10), 7193–7203. https://doi.org/10.1109/TII.2021.3063697 [Google Scholar] [CrossRef]
114. Eldrandaly, K. A., Abdel-Fatah, L., Abdel-Basset, M., El-hoseny, M., Abdel-Aziz, N. M. (2021). Green communication for sixth-generation intent-based networks: An architecture based on hybrid computational intelligence algorithm. Wireless Communications & Mobile Computing, 2021, 9931677. [Google Scholar]
115. Sheth, K., Patel, K., Shah, H., Tanwar, S., Gupta, R. et al. (2020). A taxonomy of AI techniques for 6G communication networks. Computer Communications, 161, 279–303. https://doi.org/10.1016/j.comcom.2020.07.035 [Google Scholar] [CrossRef]
116. Kaur, J., Khan, M. A., Iftikhar, M., Imran, M., UlHaq, Q. E. (2021). Machine learning techniques for 5G and beyond. IEEE Access, 9, 23472–23488. https://doi.org/10.1109/Access.6287639 [Google Scholar] [CrossRef]
117. Matin, M. A., Goudos, S. K., Wan, S., Sarigiannidis, P., Tentzeris, E. M. (2023). Artificial intelligence (AI) and machine learning (ML) for beyond 5G/6G communications. EURASIP Journal on Wireless Communications and Networking, 2023, 22. [Google Scholar]
118. Ahmed, R., Matin, M. A. (2020). Towards 6G wireless networks-challenges and potential technologies. Journal of Electrical Engineering, 71(4), 290–297. https://doi.org/10.2478/jee-2020-0040 [Google Scholar] [CrossRef]
119. Zhang, M., Zhang, H., Fang, Y., Yuan, D. (2022). Learning-based data transmissions for future 6G enabled industrial IoT: A data compression perspective. IEEE Network, 36(5), 180–187. https://doi.org/10.1109/MNET.109.2100384 [Google Scholar] [CrossRef]
120. Darman, I., Mahmood, M. K., Chaudhry, S. A., Khan, S. A., Lim, H. (2022). Designing an enhanced user authenticated key management scheme for 6G-based industrial applications. IEEE Access, 10, 92774–92787. https://doi.org/10.1109/ACCESS.2022.3198642 [Google Scholar] [CrossRef]
121. Raddo, T. R., Rommel, S., Cimoli, B., Vagionas, C., Perez-Galacho, D. et al. (2021). Transition technologies towards 6G networks. EURASIP Journal on Wireless Communications and Networking, 2021(1), 1–22. [Google Scholar]
122. Qi, Q., Chen, X., Khalili, A., Zhong, C., Zhang, Z. et al. (2022). Integrating sensing, computing, and communication in 6G wireless networks: Design and optimization. IEEE Transactions on Communications, 70(9), 6212–6227. https://doi.org/10.1109/TCOMM.2022.3190363 [Google Scholar] [CrossRef]
123. Khan, L. U., Yaqoob, I., Imran, M., Han, Z., Hong, C. S. (2020). 6G wireless systems: A vision, architectural elements, and future directions. IEEE Access, 8, 147029–147044. https://doi.org/10.1109/Access.6287639 [Google Scholar] [CrossRef]
124. Ma, Z., Yuan, X., Liang, K., Feng, J., Zhu, L. et al. (2023). Blockchain-escorted distributed deep learning with collaborative model aggregation towards 6G networks. Future Generation Computer Systems-The International Journal of E-Science, 141, 555–566. https://doi.org/10.1016/j.future.2022.11.029 [Google Scholar] [CrossRef]
125. Ahmed, S., Kumar, V., Singh, K., Singh, A., Muthukumaran, V. et al. (2022). 6G enabled federated learning for secure IoMT resource recommendation and propagation analysis. Computers and Electrical Engineering, 102, 108210. https://doi.org/10.1016/j.compeleceng.2022.108210 [Google Scholar] [CrossRef]
126. Alsabah, M., Naser, M. A., Mahmmod, B. M., Abdulhussain, S. H., Eissa, M. R. et al. (2021). 6G wireless communications networks: A comprehensive survey. IEEE Access, 9, 148191–148243. https://doi.org/10.1109/ACCESS.2021.3124812 [Google Scholar] [CrossRef]
127. Fiandrino, C., Attanasio, G., Fiore, M., Widmer, J. (2022). Toward native explainable and robust AI in 6G networks: Current state, challenges and road ahead. Computer Communications, 193, 47–52. https://doi.org/10.1016/j.comcom.2022.06.036 [Google Scholar] [CrossRef]
128. Hao, S., Zhang, H. (2022). Performance analysis of PHY layer for RIS-assisted wireless communication systems with retransmission protocols. Journal of King Saud University-Computer and Information Sciences, 34(8), 5388–5404. https://doi.org/10.1016/j.jksuci.2022.06.006 [Google Scholar] [CrossRef]
129. Xiao, Y., Shi, G., Li, Y., Saad, W., Poor, H. V. (2020). Toward self-learning edge intelligence in 6G. IEEE Communications Magazine, 58(12), 34–40. https://doi.org/10.1109/MCOM.35 [Google Scholar] [CrossRef]
130. Penttinen, J. T. (2021). On 6G visions and requirements. Journal of ICT Standardization, 9(3), 311–325. [Google Scholar]
131. Yap, K., Chin, H., Klemeš, J. (2022). Future outlook on 6G technology for renewable energy sources (RES). Renewable & Sustainable Energy Reviews, 167, 112722. https://doi.org/10.1016/j.rser.2022.112722 [Google Scholar] [CrossRef]
132. Yrjola, S., Ahokangas, P., Matinmikko-Blue, M. (2022). Value creation and capture from technology innovation in the 6G era. IEEE Access, 10, 16299–16319. https://doi.org/10.1109/ACCESS.2022.3149590 [Google Scholar] [CrossRef]
133. Hakeem, S. A. A., Hussein, H. H., Kim, H. (2022). Vision and research directions of 6G technologies and applications. Journal of King Saud University-Computer and Information Sciences, 34(6), 2419–2442. https://doi.org/10.1016/j.jksuci.2022.03.019 [Google Scholar] [CrossRef]
134. Feng, W., Wei, S., Cao, J. (2021). 6G technology development vision and terahertz communication. Acta Physica Sinica, 70(24), 244303. https://doi.org/10.7498/aps [Google Scholar] [CrossRef]
135. Jang, H. S., Jung, B. C., Quek, T. Q. S., Sung, D. K. (2021). Resource-hopping-based grant-free multiple access for 6G-enabled massive IoT networks. IEEE Internet of Things Journal, 8(20), 15349–15360. https://doi.org/10.1109/JIOT.2021.3064872 [Google Scholar] [CrossRef]
136. Lv, Z., Qiao, L., You, I. (2020). 6G-enabled network in box for internet of connected vehicles. IEEE Transactions on Intelligent Transportation Systems, 22(8), 5275–5282. [Google Scholar]
137. De Alwis, C., Kumar, P., Pham, Q. V., Dev, K., Kalla, A. et al. (2023). Towards 6G: Key technological directions. ICT Express, 9(4), 525–533. [Google Scholar]
138. Cao, K., Wang, B., Ding, H., Lv, L., Tian, J. et al. (2021). Achieving reliable and secure communications in wireless-powered NOMA systems. IEEE Transactions on Vehicular Technology, 70(2), 1978–1983. https://doi.org/10.1109/TVT.2021.3053093 [Google Scholar] [CrossRef]
139. Lv, Z., Kumar, N. (2020). Software defined solutions for sensors in 6G/IoE. Computer Communications, 153, 42–47. https://doi.org/10.1016/j.comcom.2020.01.060 [Google Scholar] [CrossRef]
140. AlDuhayyim, M., Obayya, M., Al-Wesabi, F. N., Hilal, A. M., Rizwanullah, M. et al. (2022). Energy aware data collection with route planning for 6G enabled UAV communication. Computers, Materials & Continua, 71(1), 825–842. https://doi.org/10.32604/cmc.2022.021490 [Google Scholar] [CrossRef]
141. Hussain, M., Shah, N., Amin, R., Alshamrani, S. S., Alotaibi, A. et al. (2022). Software-defined networking: Categories, analysis, and future directions. Sensors, 22(15), 5551. https://doi.org/10.3390/s22155551. [Google Scholar] [PubMed] [CrossRef]
142. Liao, L., Zhao, J., Hu, H., Sun, X. (2022). Secure and efficient message authentication scheme for 6G-enabled VANETs. Electronics, 11(15), 2385. https://doi.org/10.3390/electronics11152385 [Google Scholar] [CrossRef]
143. Lin, S. C., Chen, K. C., Karimoddini, A. (2021). SDVEC: Software-defined vehicular edge computing with ultra-low latency. IEEE Communications Magazine, 59(12), 66–72. https://doi.org/10.1109/MCOM.004.2001124 [Google Scholar] [CrossRef]
144. Long, Q., Chen, Y., Zhang, H., Lei, X. (2019). Software defined 5G and 6G networks: A survey. Mobile Networks and Applications, 27, 1792–1812. [Google Scholar]
145. Deebak, B., Hwang, S. O. (2023). Intelligent drone-assisted robust lightweight multi-factor authentication for military zone surveillance in the 6G era. Computer Networks, 225, 109664. https://doi.org/10.1016/j.comnet.2023.109664 [Google Scholar] [CrossRef]
146. Kamble, P., Shaikh, A. N. (2022). 6G wireless networks: Vision, requirements, applications and challenges. 2022 5th International Conference on Advances in Science and Technology (ICAST), Mumbai, India, IEEE. [Google Scholar]
Cite This Article
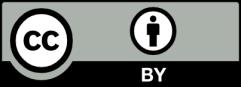