Open Access
ARTICLE
Contrast Normalization Strategies in Brain Tumor Imaging: From Preprocessing to Classification
1 Department of Information Systems, College of Computer Science and Information Systems, Najran University, Najran, 61441, Saudi Arabia
2 Department of Electronic Engineering, The University of Larkano, Larkana, Sindh, 75660, Pakistan
3 Computer Science Department, Sukkur IBA University, Sukkur, Sindh, 65200, Pakistan
4 Eletrical Engineering Department, Sukkur IBA University, Sukkur, Sindh, 65200, Pakistan
5 Electrical Engineering Department, College of Engineering, Najran University, Najran, 61441, Saudi Arabia
6 Radiological Sciences Department, College of Applied Medical Sciences, Najran University, Najran, 61441, Saudi Arabia
* Corresponding Author: Toufique A. Soomro. Email:
Computer Modeling in Engineering & Sciences 2024, 140(2), 1539-1562. https://doi.org/10.32604/cmes.2024.051475
Received 06 March 2024; Accepted 15 April 2024; Issue published 20 May 2024
Abstract
Cancer-related to the nervous system and brain tumors is a leading cause of mortality in various countries. Magnetic resonance imaging (MRI) and computed tomography (CT) are utilized to capture brain images. MRI plays a crucial role in the diagnosis of brain tumors and the examination of other brain disorders. Typically, manual assessment of MRI images by radiologists or experts is performed to identify brain tumors and abnormalities in the early stages for timely intervention. However, early diagnosis of brain tumors is intricate, necessitating the use of computerized methods. This research introduces an innovative approach for the automated segmentation of brain tumors and a framework for classifying different regions of brain tumors. The proposed methods consist of a pipeline with several stages: preprocessing of brain images with noise removal based on Wiener Filtering, enhancing the brain using Principal Component Analysis (PCA) to obtain well-enhanced images, and then segmenting the region of interest using the Fuzzy C-Means (FCM) clustering technique in the third step. The final step involves classification using the Support Vector Machine (SVM) classifier. The classifier is applied to various types of brain tumors, such as meningioma and pituitary tumors, utilizing the Contrast-Enhanced Magnetic Resonance Imaging (CE-MRI) database. The proposed method demonstrates significantly improved contrast and validates the effectiveness of the classification framework, achieving an average sensitivity of 0.974, specificity of 0.976, accuracy of 0.979, and a Dice Score (DSC) of 0.957. Additionally, this method exhibits a shorter processing time of 0.44 s compared to existing approaches. The performance of this method emphasizes its significance when compared to state-of-the-art methods in terms of sensitivity, specificity, accuracy, and DSC. To enhance the method further in the future, it is feasible to standardize the approach by incorporating a set of classifiers to increase the robustness of the brain classification method.Graphical Abstract
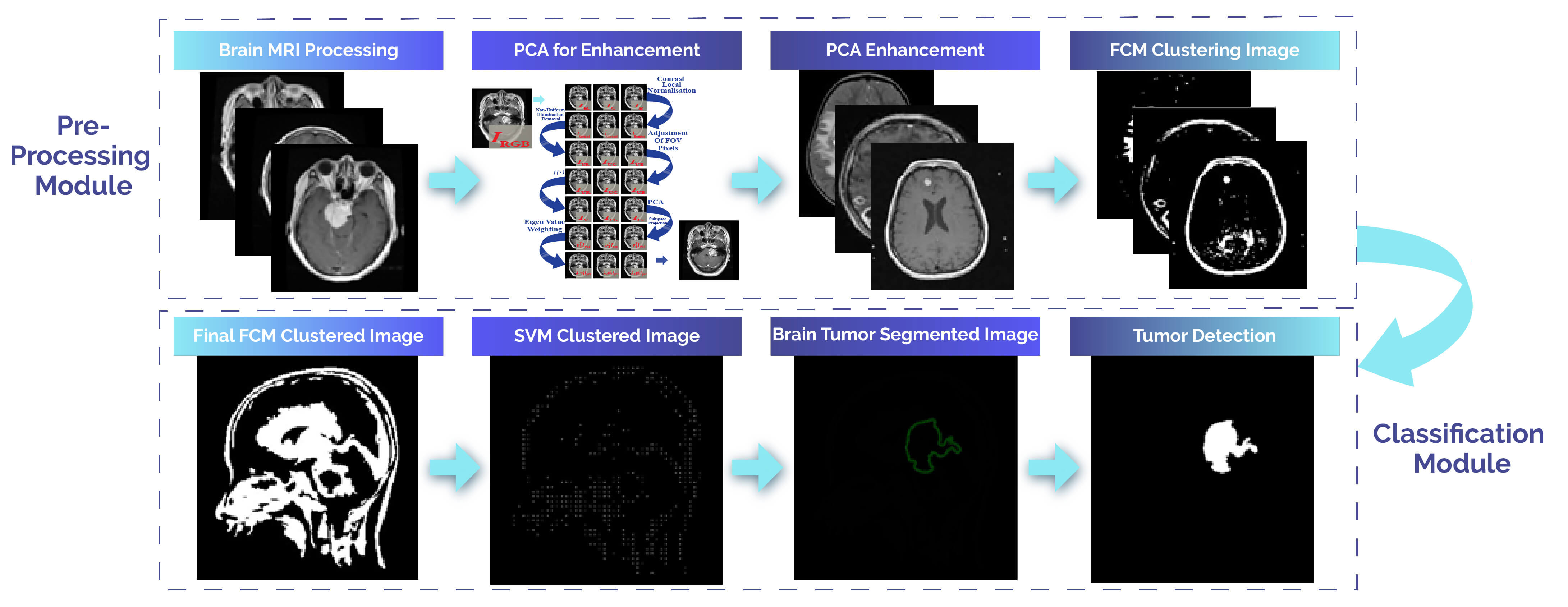
Keywords
Cite This Article
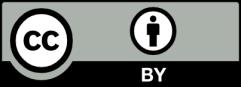