Open Access
ARTICLE
Highly Differentiated Target Detection under Extremely Low-Light Conditions Based on Improved YOLOX Model
1 School of Computer, Jiangsu University of Science and Technology, Zhenjiang, 212003, China
2 Department of Electrical and Computer Engineering, University of Nevada, Las Vegas, NV, 89154, USA
3 Zhejiang Geely Automobile Research Institute, Ningbo, 315336, China
* Corresponding Author: Haijian Shao. Email:
Computer Modeling in Engineering & Sciences 2024, 140(2), 1507-1537. https://doi.org/10.32604/cmes.2024.050140
Received 28 January 2024; Accepted 12 April 2024; Issue published 20 May 2024
Abstract
This paper expounds upon a novel target detection methodology distinguished by its elevated discriminatory efficacy, specifically tailored for environments characterized by markedly low luminance levels. Conventional methodologies struggle with the challenges posed by luminosity fluctuations, especially in settings characterized by diminished radiance, further exacerbated by the utilization of suboptimal imaging instrumentation. The envisioned approach mandates a departure from the conventional YOLOX model, which exhibits inadequacies in mitigating these challenges. To enhance the efficacy of this approach in low-light conditions, the dehazing algorithm undergoes refinement, effecting a discerning regulation of the transmission rate at the pixel level, reducing it to values below 0.5, thereby resulting in an augmentation of image contrast. Subsequently, the coiflet wavelet transform is employed to discern and isolate high-discriminatory attributes by dismantling low-frequency image attributes and extracting high-frequency attributes across divergent axes. The utilization of CycleGAN serves to elevate the features of low-light imagery across an array of stylistic variances. Advanced computational methodologies are then employed to amalgamate and conflate intricate attributes originating from images characterized by distinct stylistic orientations, thereby augmenting the model’s erudition potential. Empirical validation conducted on the PASCAL VOC and MS COCO 2017 datasets substantiates pronounced advancements. The refined low-light enhancement algorithm yields a discernible 5.9% augmentation in the target detection evaluation index when compared to the original imagery. Mean Average Precision (mAP) undergoes enhancements of 9.45% and 0.052% in low-light visual renditions relative to conventional YOLOX outcomes. The envisaged approach presents a myriad of advantages over prevailing benchmark methodologies in the realm of target detection within environments marked by an acute scarcity of luminosity.Graphical Abstract
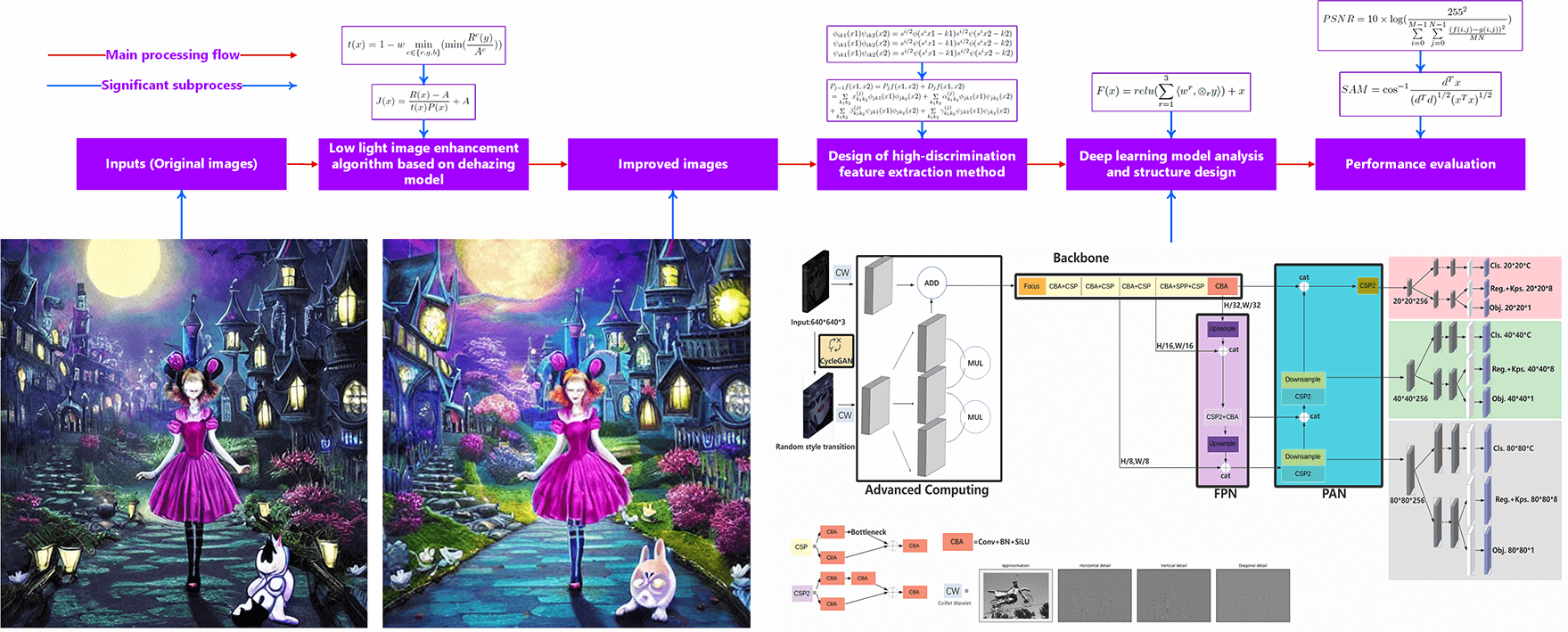
Keywords
Cite This Article
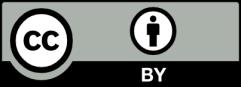