Open Access
ARTICLE
Energy-Saving Distributed Flexible Job Shop Scheduling Optimization with Dual Resource Constraints Based on Integrated Q-Learning Multi-Objective Grey Wolf Optimizer
1 School of Management Science and Engineering, Anhui University of Technology, Ma’anshan, 243032, China
2 Laboratory of Multidisciplinary Management and Control of Complex Systems of Anhui Higher Education Institutes, Anhui University of Technology, Ma’anshan, 243032, China
3 Department of Industrial Engineering, School of Mechanical Engineering, Northwestern Polytechnical University, Xi’an, 710072, China
* Corresponding Author: Gongjie Xu. Email:
Computer Modeling in Engineering & Sciences 2024, 140(2), 1459-1483. https://doi.org/10.32604/cmes.2024.049756
Received 17 January 2024; Accepted 27 March 2024; Issue published 20 May 2024
Abstract
The distributed flexible job shop scheduling problem (DFJSP) has attracted great attention with the growth of the global manufacturing industry. General DFJSP research only considers machine constraints and ignores worker constraints. As one critical factor of production, effective utilization of worker resources can increase productivity. Meanwhile, energy consumption is a growing concern due to the increasingly serious environmental issues. Therefore, the distributed flexible job shop scheduling problem with dual resource constraints (DFJSP-DRC) for minimizing makespan and total energy consumption is studied in this paper. To solve the problem, we present a multi-objective mathematical model for DFJSP-DRC and propose a Q-learning-based multi-objective grey wolf optimizer (Q-MOGWO). In Q-MOGWO, high-quality initial solutions are generated by a hybrid initialization strategy, and an improved active decoding strategy is designed to obtain the scheduling schemes. To further enhance the local search capability and expand the solution space, two wolf predation strategies and three critical factory neighborhood structures based on Q-learning are proposed. These strategies and structures enable Q-MOGWO to explore the solution space more efficiently and thus find better Pareto solutions. The effectiveness of Q-MOGWO in addressing DFJSP-DRC is verified through comparison with four algorithms using 45 instances. The results reveal that Q-MOGWO outperforms comparison algorithms in terms of solution quality.Keywords
Cite This Article
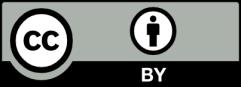