Open Access
ARTICLE
Sleep Posture Classification Using RGB and Thermal Cameras Based on Deep Learning Model
1 Department of ICT Convergence, Soonchunhyang University, Asan, 31538, Korea
2 ICT Convergence Research Center, Soonchunhyang University, Asan, 31538, Korea
3 Emotional and Intelligent Child Care Convergence Research Center, Soonchunhyang University, Asan, 31538, Korea
* Corresponding Author: Yunyoung Nam. Email:
(This article belongs to the Special Issue: Artificial Intelligence Emerging Trends and Sustainable Applications in Image Processing and Computer Vision)
Computer Modeling in Engineering & Sciences 2024, 140(2), 1729-1755. https://doi.org/10.32604/cmes.2024.049618
Received 12 January 2024; Accepted 14 March 2024; Issue published 20 May 2024
Abstract
Sleep posture surveillance is crucial for patient comfort, yet current systems face difficulties in providing comprehensive studies due to the obstruction caused by blankets. Precise posture assessment remains challenging because of the complex nature of the human body and variations in sleep patterns. Consequently, this study introduces an innovative method utilizing RGB and thermal cameras for comprehensive posture classification, thereby enhancing the analysis of body position and comfort. This method begins by capturing a dataset of sleep postures in the form of videos using RGB and thermal cameras, which depict six commonly adopted postures: supine, left log, right log, prone head, prone left, and prone right. The study involves 10 participants under two conditions: with and without blankets. Initially, the database is normalized into a video frame. The subsequent step entails training a fine-tuned, pretrained Visual Geometry Group (VGG16) and ResNet50 model. In the third phase, the extracted features are utilized for classification. The fourth step of the proposed approach employs a serial fusion technique based on the normal distribution to merge the vectors derived from both the RGB and thermal datasets. Finally, the fused vectors are passed to machine learning classifiers for final classification. The dataset, which includes human sleep postures used in this study’s experiments, achieved a 96.7% accuracy rate using the Quadratic Support Vector Machine (QSVM) without the blanket. Moreover, the Linear SVM, when utilized with a blanket, attained an accuracy of 96%. When normal distribution serial fusion was applied to the blanket features, it resulted in a remarkable average accuracy of 99%.Graphic Abstract
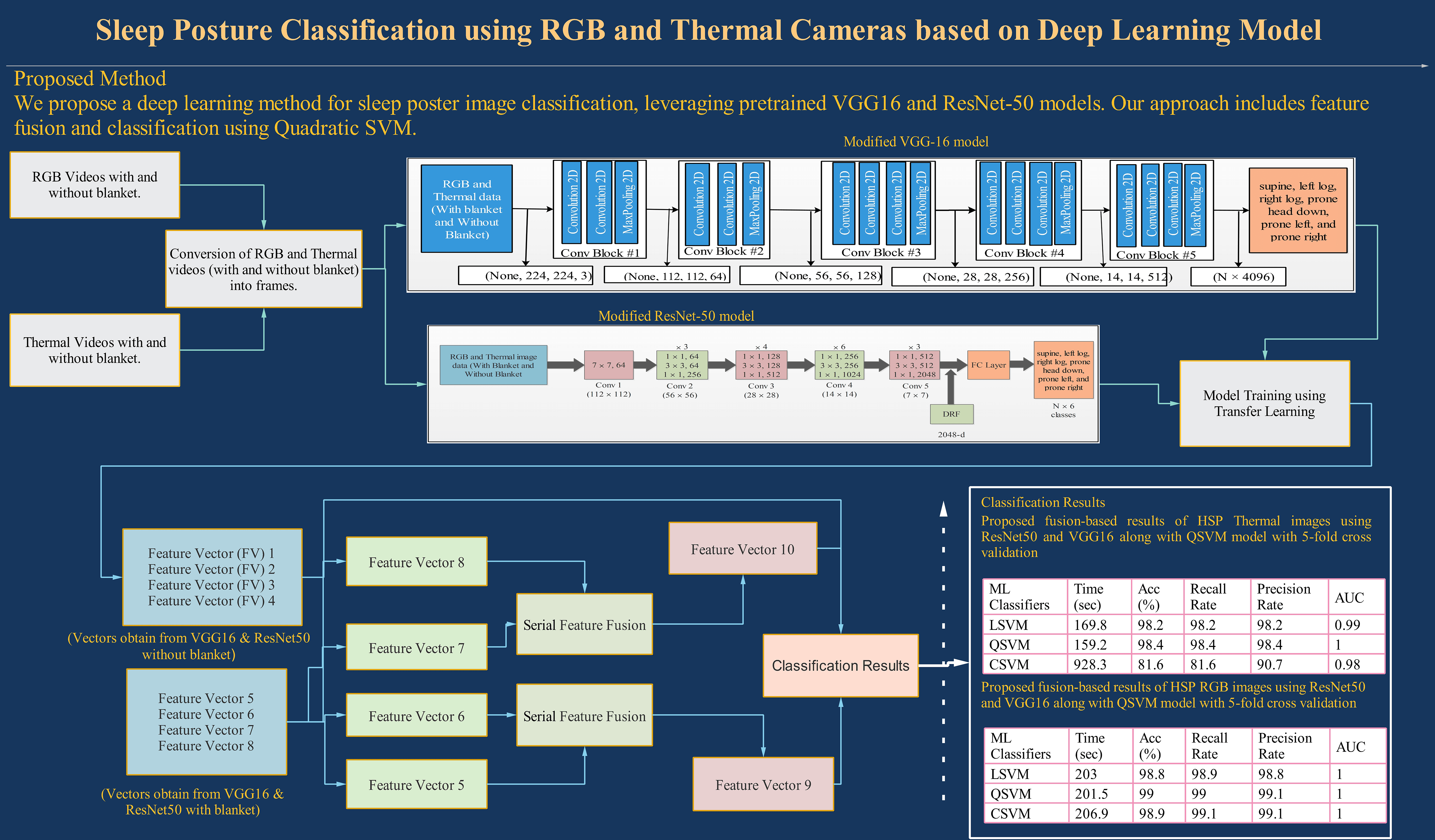
Keywords
Cite This Article
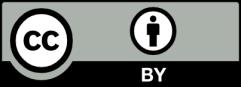
This work is licensed under a Creative Commons Attribution 4.0 International License , which permits unrestricted use, distribution, and reproduction in any medium, provided the original work is properly cited.