Open Access
ARTICLE
2P3FL: A Novel Approach for Privacy Preserving in Financial Sectors Using Flower Federated Learning
School of Computer Science Engineering and Information Systems, Vellore Institute of Technology, Vellore, 632014, India
* Corresponding Author: Rajesh Kaluri. Email:
(This article belongs to the Special Issue: Privacy-Preserving Technologies for Large-scale Artificial Intelligence)
Computer Modeling in Engineering & Sciences 2024, 140(2), 2035-2051. https://doi.org/10.32604/cmes.2024.049152
Received 29 December 2023; Accepted 06 March 2024; Issue published 20 May 2024
Abstract
The increasing data pool in finance sectors forces machine learning (ML) to step into new complications. Banking data has significant financial implications and is confidential. Combining users data from several organizations for various banking services may result in various intrusions and privacy leakages. As a result, this study employs federated learning (FL) using a flower paradigm to preserve each organization’s privacy while collaborating to build a robust shared global model. However, diverse data distributions in the collaborative training process might result in inadequate model learning and a lack of privacy. To address this issue, the present paper proposes the implementation of Federated Averaging (FedAvg) and Federated Proximal (FedProx) methods in the flower framework, which take advantage of the data locality while training and guaranteeing global convergence. Resultantly improves the privacy of the local models. This analysis used the credit card and Canadian Institute for Cybersecurity Intrusion Detection Evaluation (CICIDS) datasets. Precision, recall, and accuracy as performance indicators to show the efficacy of the proposed strategy using FedAvg and FedProx. The experimental findings suggest that the proposed approach helps to safely use banking data from diverse sources to enhance customer banking services by obtaining accuracy of 99.55% and 83.72% for FedAvg and 99.57%, and 84.63% for FedProx.Graphic Abstract
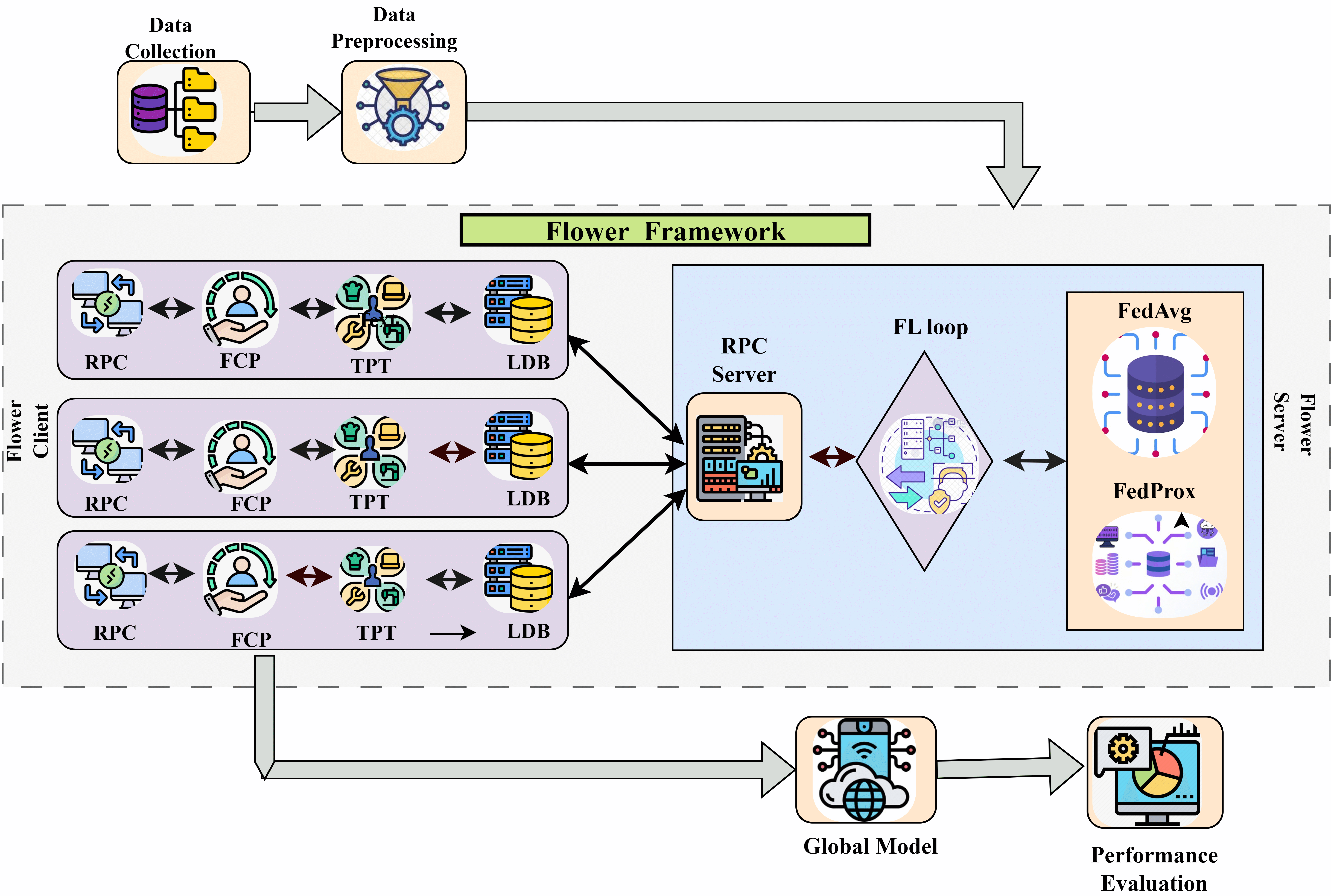
Keywords
Cite This Article
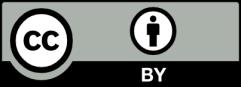
This work is licensed under a Creative Commons Attribution 4.0 International License , which permits unrestricted use, distribution, and reproduction in any medium, provided the original work is properly cited.