Open Access
ARTICLE
Traffic Flow Prediction with Heterogeneous Spatiotemporal Data Based on a Hybrid Deep Learning Model Using Attention-Mechanism
1 Departement of Computer Science and Information Engineering, Asia University, Taichung, Taiwan
2 Departement of Information Technology, Universitas Muhammadiyah Yogyakarta, Yogyakarta, Indonesia
* Corresponding Author: Chayadi Oktomy Noto Susanto. Email:
(This article belongs to the Special Issue: Artificial Intelligence Emerging Trends and Sustainable Applications in Image Processing and Computer Vision)
Computer Modeling in Engineering & Sciences 2024, 140(2), 1711-1728. https://doi.org/10.32604/cmes.2024.048955
Received 22 December 2023; Accepted 08 March 2024; Issue published 20 May 2024
Abstract
A significant obstacle in intelligent transportation systems (ITS) is the capacity to predict traffic flow. Recent advancements in deep neural networks have enabled the development of models to represent traffic flow accurately. However, accurately predicting traffic flow at the individual road level is extremely difficult due to the complex interplay of spatial and temporal factors. This paper proposes a technique for predicting short-term traffic flow data using an architecture that utilizes convolutional bidirectional long short-term memory (Conv-BiLSTM) with attention mechanisms. Prior studies neglected to include data pertaining to factors such as holidays, weather conditions, and vehicle types, which are interconnected and significantly impact the accuracy of forecast outcomes. In addition, this research incorporates recurring monthly periodic pattern data that significantly enhances the accuracy of forecast outcomes. The experimental findings demonstrate a performance improvement of 21.68% when incorporating the vehicle type feature.Keywords
Cite This Article
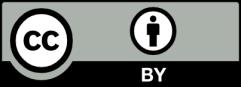
This work is licensed under a Creative Commons Attribution 4.0 International License , which permits unrestricted use, distribution, and reproduction in any medium, provided the original work is properly cited.