Open Access
ARTICLE
An Improved Deep Learning Framework for Automated Optic Disc Localization and Glaucoma Detection
1 Department of Information Technology, College of Computer and Information Sciences, Princess Nourah bint Abdulrahman University, P.O. Box 84428, Riyadh, 11671, Saudi Arabia
2 Department of Computer Sciences, College of Computer and Information Sciences, Princess Nourah bint Abdulrahman University, P.O. Box 84428, Riyadh, 11671, Saudi Arabia
3 Department of Information System, College of Computer and Information Sciences, Princess Nourah bint Abdulrahman University, P.O. Box 84428, Riyadh, 11671, Saudi Arabia
* Corresponding Author: Hela Elmannai. Email:
Computer Modeling in Engineering & Sciences 2024, 140(2), 1429-1457. https://doi.org/10.32604/cmes.2024.048557
Received 06 December 2023; Accepted 27 March 2024; Issue published 20 May 2024
Abstract
Glaucoma disease causes irreversible damage to the optical nerve and it has the potential to cause permanent loss of vision. Glaucoma ranks as the second most prevalent cause of permanent blindness. Traditional glaucoma diagnosis requires a highly experienced specialist, costly equipment, and a lengthy wait time. For automatic glaucoma detection, state-of-the-art glaucoma detection methods include a segmentation-based method to calculate the cup-to-disc ratio. Other methods include multi-label segmentation networks and learning-based methods and rely on hand-crafted features. Localizing the optic disc (OD) is one of the key features in retinal images for detecting retinal diseases, especially for glaucoma disease detection. The approach presented in this study is based on deep classifiers for OD segmentation and glaucoma detection. First, the optic disc detection process is based on object detection using a Mask Region-Based Convolutional Neural Network (Mask-RCNN). The OD detection task was validated using the Dice score, intersection over union, and accuracy metrics. The OD region is then fed into the second stage for glaucoma detection. Therefore, considering only the OD area for glaucoma detection will reduce the number of classification artifacts by limiting the assessment to the optic disc area. For this task, VGG-16 (Visual Geometry Group), Resnet-18 (Residual Network), and Inception-v3 were pre-trained and fine-tuned. We also used the Support Vector Machine Classifier. The feature-based method uses region content features obtained by Histogram of Oriented Gradients (HOG) and Gabor Filters. The final decision is based on weighted fusion. A comparison of the obtained results from all classification approaches is provided. Classification metrics including accuracy and ROC curve are compared for each classification method. The novelty of this research project is the integration of automatic OD detection and glaucoma diagnosis in a global method. Moreover, the fusion-based decision system uses the glaucoma detection result obtained using several convolutional deep neural networks and the support vector machine classifier. These classification methods contribute to producing robust classification results. This method was evaluated using well-known retinal images available for research work and a combined dataset including retinal images with and without pathology. The performance of the models was tested on two public datasets and a combined dataset and was compared to similar research. The research findings show the potential of this methodology in the early detection of glaucoma, which will reduce diagnosis time and increase detection efficiency. The glaucoma assessment achieves about 98% accuracy in the classification rate, which is close to and even higher than that of state-of-the-art methods. The designed detection model may be used in telemedicine, healthcare, and computer-aided diagnosis systems.Keywords
Cite This Article
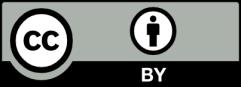
This work is licensed under a Creative Commons Attribution 4.0 International License , which permits unrestricted use, distribution, and reproduction in any medium, provided the original work is properly cited.