Open Access
ARTICLE
Advanced Machine Learning Methods for Prediction of Blast-Induced Flyrock Using Hybrid SVR Methods
1 College of Civil and Environmental Engineering, Hunan University of Science and Engineering, Yongzhou, 425006, China
2 Hunan Provincial Key Laboratory of Intelligent Protection and Utilization Technology in Masonry Artifacts, Hunan University of Science and Engineering, Yongzhou, 425006, China
3 School of Civil Engineering, Guangzhou University, Guangzhou, 510006, China
4 Institute of Research and Development, Duy Tan University, Da Nang, 550000, Vietnam
5 School of Engineering & Technology, Duy Tan University, Da Nang, 550000, Vietnam
* Corresponding Authors: Mahdi Hasanipanah. Email: ,
; Jiandong Huang. Email:
(This article belongs to the Special Issue: Meta-heuristic Algorithms in Materials Science and Engineering)
Computer Modeling in Engineering & Sciences 2024, 140(2), 1595-1617. https://doi.org/10.32604/cmes.2024.048398
Received 06 December 2023; Accepted 06 March 2024; Issue published 20 May 2024
Abstract
Blasting in surface mines aims to fragment rock masses to a proper size. However, flyrock is an undesirable effect of blasting that can result in human injuries. In this study, support vector regression (SVR) is combined with four algorithms: gravitational search algorithm (GSA), biogeography-based optimization (BBO), ant colony optimization (ACO), and whale optimization algorithm (WOA) for predicting flyrock in two surface mines in Iran. Additionally, three other methods, including artificial neural network (ANN), kernel extreme learning machine (KELM), and general regression neural network (GRNN), are employed, and their performances are compared to those of four hybrid SVR models. After modeling, the measured and predicted flyrock values are validated with some performance indices, such as root mean squared error (RMSE). The results revealed that the SVR-WOA model has the most optimal accuracy, with an RMSE of 7.218, while the RMSEs of the KELM, GRNN, SVR-GSA, ANN, SVR-BBO, and SVR-ACO models are 10.668, 10.867, 15.305, 15.661, 16.239, and 18.228, respectively. Therefore, combining WOA and SVR can be a valuable tool for accurately predicting flyrock distance in surface mines.Keywords
Cite This Article
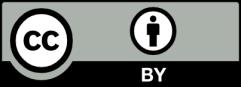
This work is licensed under a Creative Commons Attribution 4.0 International License , which permits unrestricted use, distribution, and reproduction in any medium, provided the original work is properly cited.